A novel day-ahead regional and probabilistic wind power forecasting framework using deep CNNs and conformalized regression forests
APPLIED ENERGY(2024)
Abstract
Regional forecasting is crucial for a balanced energy delivery system and for achieving the global transition to clean energy. However, regional wind forecasting is challenging due to uncertain weather prediction and its high dimensional nature. Most solutions are limited to single -turbine or farm/park forecasting; therefore, this work proposes a day -ahead regional wind power forecasting framework using deep Convolutional Neural Networks (CNN) with context -aware turbine maps and Conformal Quantile Regression (CQR) to generate quantile forecasts with valid coverage. Additionally, this work introduces the use of the Split Conformal Predictive System (SCPS) to generate valid prediction distributions, which has not yet been proposed for wind power forecasting in general. As well as a new method to generate calibrated prediction distributions based on SCPS and Quantile Regression Forests (QRF). This new method, named Split Conformal Distribution Regression Forests (SCDRF), allows for conditional conformal predictive distribution that increases efficiency compared to SCPS while maintaining valid coverage. SCDRF, together with CNNs and context -aware turbine maps, outperforms the existing models on the evaluated dataset, reducing the pinball loss by 5.89% while having more flexibility due to the generation of prediction distributions that can be used to generate any quantile prediction without retraining the model.
MoreTranslated text
Key words
Regional wind power forecasting,Quantile forecasting,Convolutional Neural Networks (CNN),Prediction distribution,Conformal predictive distribution,Quantile regression forest
AI Read Science
Must-Reading Tree
Example
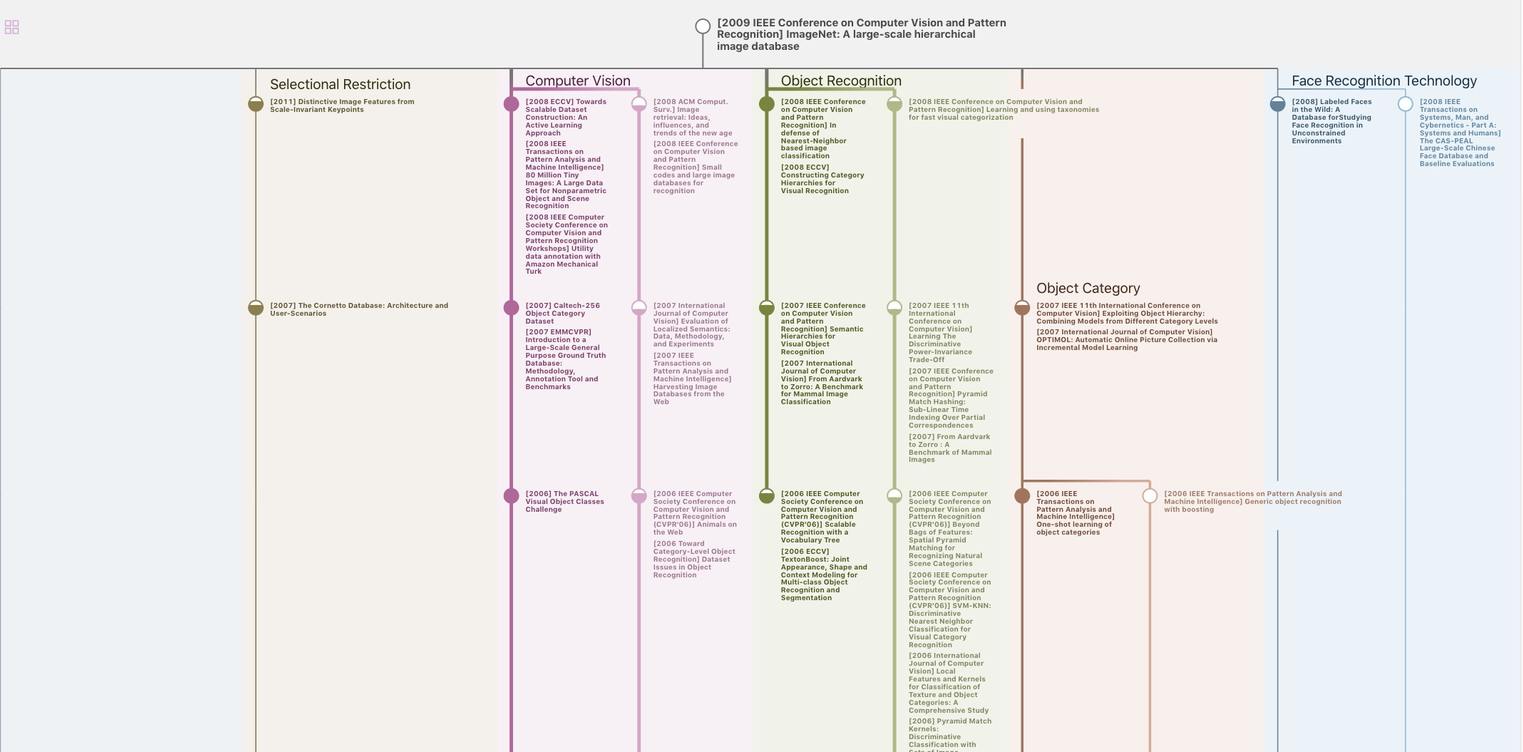
Generate MRT to find the research sequence of this paper
Chat Paper
Summary is being generated by the instructions you defined