A machine-learning-based composition design of ternary Cu-based Rochow-Müller catalyst with high M2 selectivity
Applied Catalysis A: General(2024)
摘要
To find ternary Cu-based catalysts (Cu/Cu2O/CuO) compositions with the highest M2 selectivity(SM2) in Rochow-Müller reaction, a machine learning (ML) framework including prediction and optimization models was created. The Stacking integration approach was used to describe the relationship between the catalyst's structures features and SM2 for prediction model. Further, the composition space was effectively searched for compositions with the highest SM2 by optimization model with the genetic algorithm (GA) in the base of prediction model. Finally, the greatest SM2 catalyst compositions were effectively suggested by the ML framework, and the constructed catalyst had an SM2 of 82.35%, with a relative error of only 0.93% from the predicted value. ML model combined with experimental characterization was used to infer the synergistic mechanism of the ternary Cu-based catalysts. Cu/Cu2O and Cu2O/CuO contact surfaces can create heterojunction and Schottky junction structures, respectively, to facilitate the transport of electron-hole pairs and the formation of active spices. ML framework achieved compositional optimization of catalysts and were successfully integrated with materials science theory.
更多查看译文
关键词
Rochow reaction,Machine learning,Composition optimization,M2 selectivity
AI 理解论文
溯源树
样例
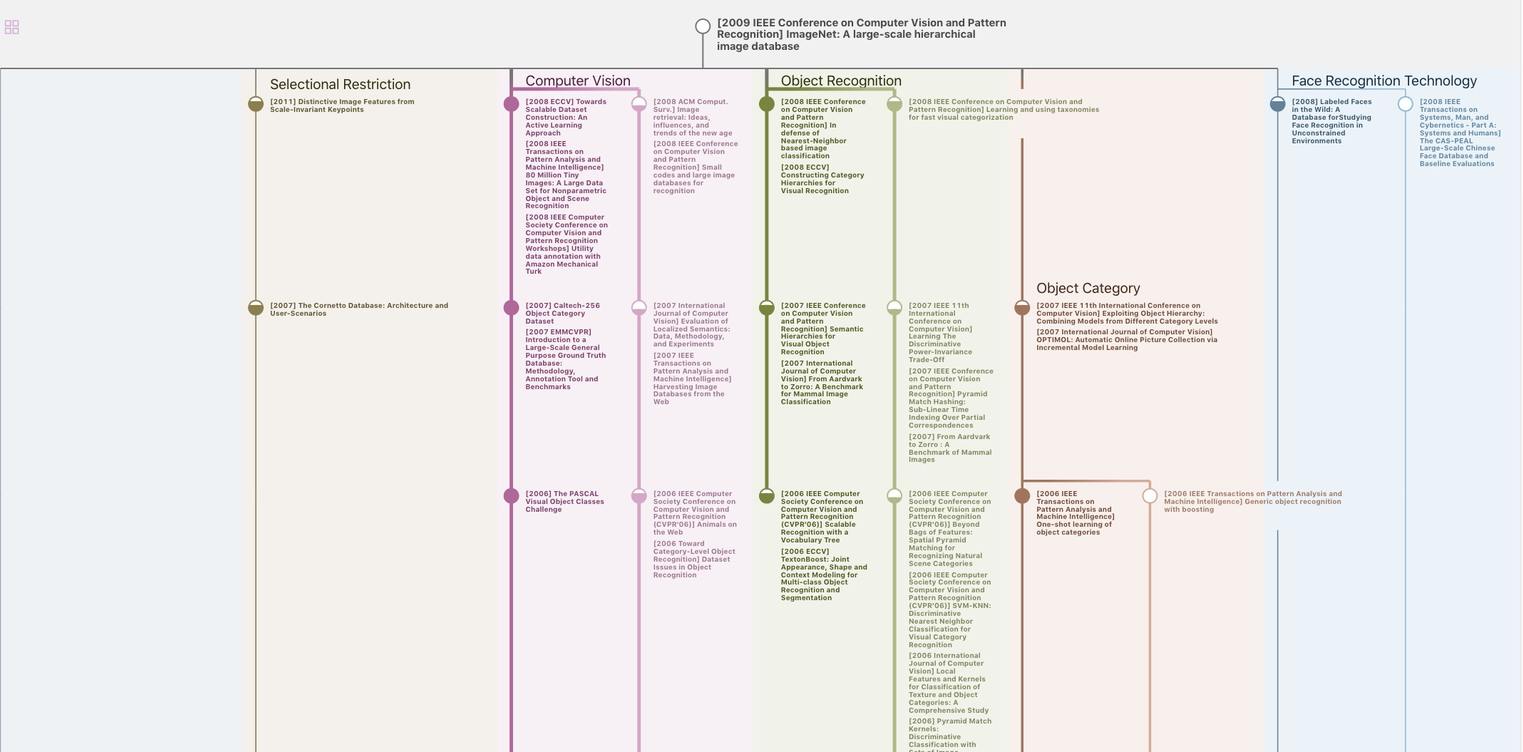
生成溯源树,研究论文发展脉络
Chat Paper
正在生成论文摘要