Performance analysis of data-driven and physics-informed machine learning methods for thermal-hydraulic processes in Full-scale Emplacement experiment
APPLIED THERMAL ENGINEERING(2024)
摘要
This study centers on the critical aspect of thermal management in deep geological repositories, particularly concerning the heat induced by radioactive nuclear waste. It evaluates eight machine learning methods to analyze thermal-hydraulic processes, focusing on their accuracy in predicting temperature and relative humidity, computational efficiency, robustness, and sensitivity to parameters. These methods are applied to data from the Full-scale Emplacement experiment in the Mont Terri underground laboratory. Notably, the Physics-Informed Machine Learning approach incorporates the experiment's heater power output conditions. The results demonstrate the effectiveness of these methods, especially the Physics-Informed Machine Learning model, in simulating complex processes typical of deep geological repository systems. The study highlights the k-Nearest Neighbor model's efficacy due to its proximity-based decision-making and the superior performance of the Physics-Informed model, suggesting its broader applicability. Our findings offer valuable insights for enhancing the design, management, and safety of underground facilities, contributing novel tools for precision and computational efficiency in energy process simulations.
更多查看译文
关键词
Machine learning,Physics-informed,Thermal-hydraulic,Full-scale Emplacement experiment,Design optimization
AI 理解论文
溯源树
样例
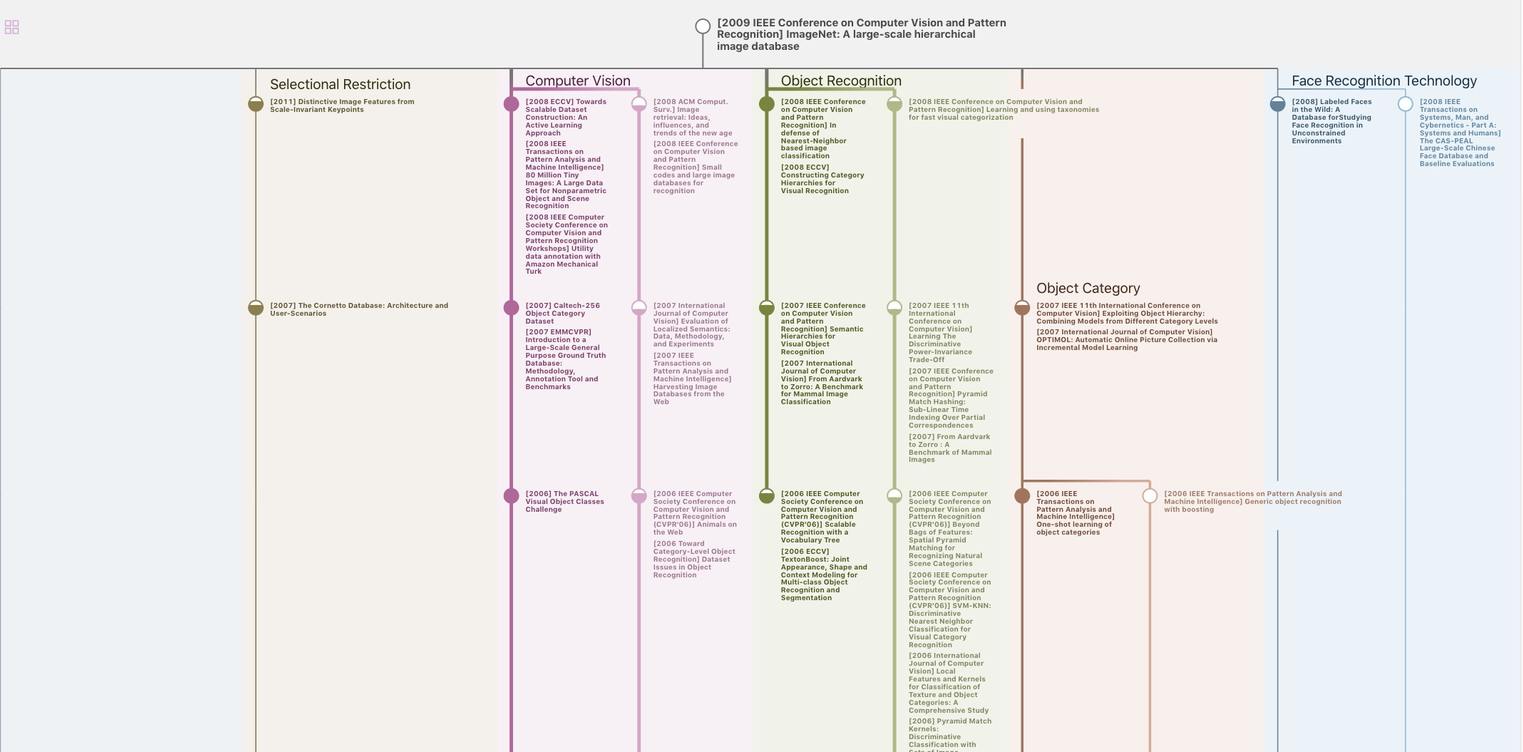
生成溯源树,研究论文发展脉络
Chat Paper
正在生成论文摘要