Pruned lightweight neural networks for arrhythmia classification with clinical 12-Lead ECGs
APPLIED SOFT COMPUTING(2024)
摘要
Real-time electrocardiogram (ECG) monitoring through portable or wearable devices is critical for detecting lethal arrhythmias. Despite the accuracy of 12-lead ECGs in clinical image analysis, their integration into portable devices poses challenges. This paper introduces a novel method for nonmalignant arrhythmia classification, optimized for wearable and portable devices. We utilize ECG records from Shanghai First People's Hospital, proposing a lightweight neural network strategy involving benchmark network selection, model pruning, and learning rate decay-based finetuning. The proposed Random Horizontal Flip (RHF)-based classification method demonstrated superior performance, achieving a 94.8 % accuracy on a 12-lead clinical ECG test dataset. Utilizing the modified pruning method, the classification accuracy for five-class ECGs improved by 7.2 % over the benchmark network. The model size was reduced by 51.26 %, with parameters and FLOPs decreasing by 47.6 % and 49.1 %, respectively, compared to the benchmark, all under identical hardware conditions. Further experiments were conducted on the Hold-out Test Set (HTS), designed to include ECGs that present slight variations to the original conditions, yielding a slightly lower accuracy of 92.4 %, reflecting the dataset's complexity and clinical variability. Moreover, benchmarking tests using the Massachusetts Institute of Technology-Beth Israel Hospital (MIT-BIH) arrhythmia database validated the method's effectiveness, achieving a 99.24 % accuracy and maintaining a lightweight model of 4366KB. Comparative analysis with existing methods confirmed the proposed method's superiority in accuracy and real-world applicability. This research presents a significant advancement in ECG analysis, offering a viable solution for efficient arrhythmia monitoring in portable healthcare devices.
更多查看译文
关键词
12-lead clinical ECGs,Arrythmia classification,Network pruning,Lightweight network
AI 理解论文
溯源树
样例
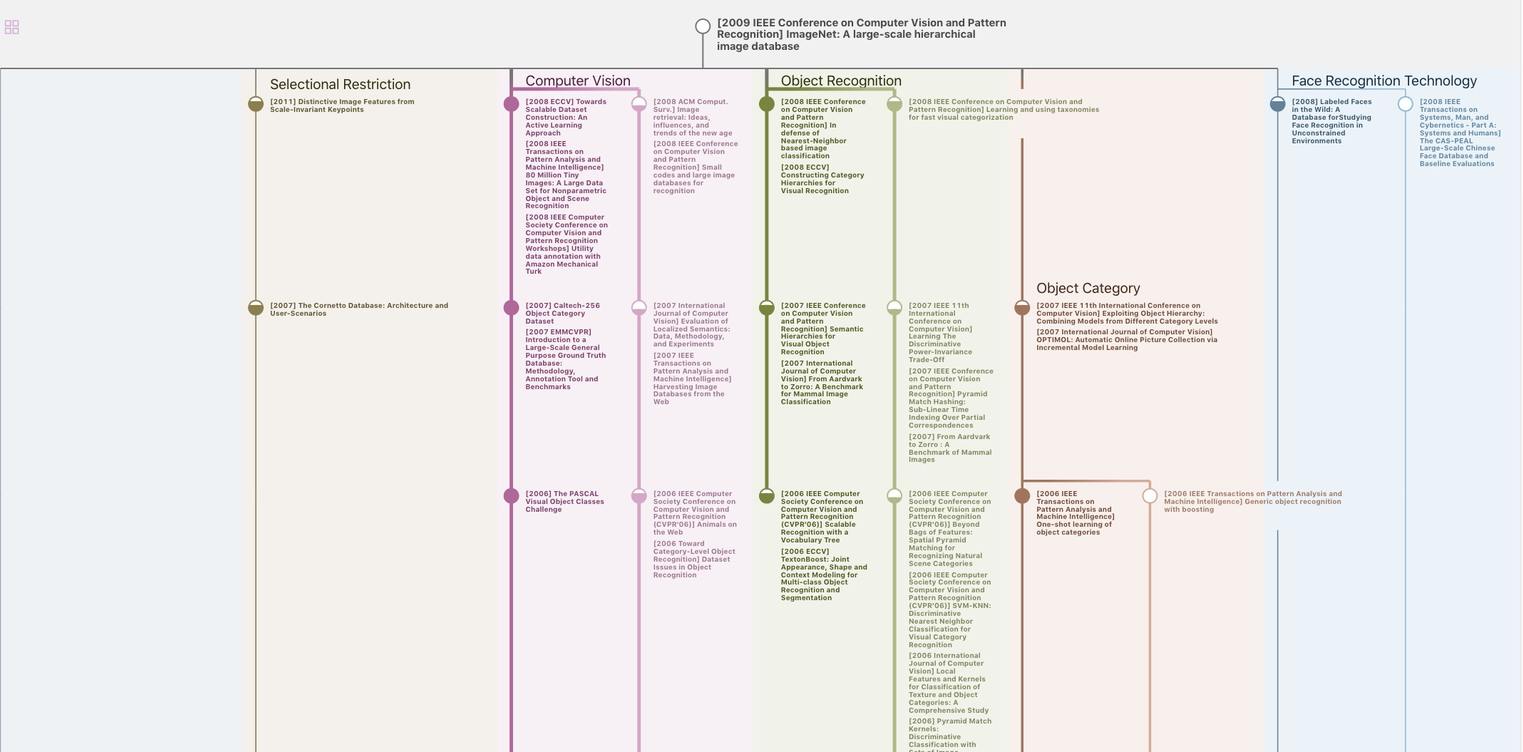
生成溯源树,研究论文发展脉络
Chat Paper
正在生成论文摘要