Peak and ultimate stress-strain model of confined ultra-high-performance concrete (UHPC) using hybrid machine learning model with conditional tabular generative adversarial network
APPLIED SOFT COMPUTING(2024)
Abstract
Ultra-high-performance concrete (UHPC) has gained prominence owing to its exceptional physical and mechanical properties, along with enhanced sustainability, making it ideal for large-scale structural applications. While numerous analytical studies have focused on predicting the stress-strain response of unconfined UHPC, there remains a lack of a reliable model for predicting the stress-strain response of confined UHPC, which poses challenges to efficient design and broader adoption, particularly in seismically active regions. To bridge this gap, the present study introduces a framework that implements machine learning (ML) models augmented by a stateof-the-art conditional tabular generative adversarial network (CTGAN) and Optuna, a next-generation optimization framework, to accurately predict the peak and ultimate axial stress-strain responses of UHPC confined with either normal-strength steel or high-strength steel. The Optuna-optimized CTGAN is employed to address the issue of limited data by generating synthetic datasets of hypothetical confined UHPC specimens. A comprehensive database of confined UHPC stress-strain responses was compiled from existing literature and used to condition the CTGAN. The augmented database is then used to develop a hybrid ML model that integrates extreme gradient boosting, gradient boosting machine, support vector regression, and K-nearest neighbors for predicting peak and ultimate stress-strain responses of confined UHPC. The predictive accuracy of the proposed hybrid ML model is evaluated and compared with a diverse set of ML models of varying complexity, and the results demonstrate its superior performance in predicting the peak and ultimate stress-strain responses of confined UHPC. Furthermore, a graphical user interface of the proposed model is developed to facilitate its practical implementation and provide a rapid, intelligent, and accurate prediction of the stress-strain response of confined UHPC at both peak and ultimate states.
MoreTranslated text
Key words
UHPC,Machine learning,Conditional tabular generative adversarial,network,GAN,Stress-strain model
AI Read Science
Must-Reading Tree
Example
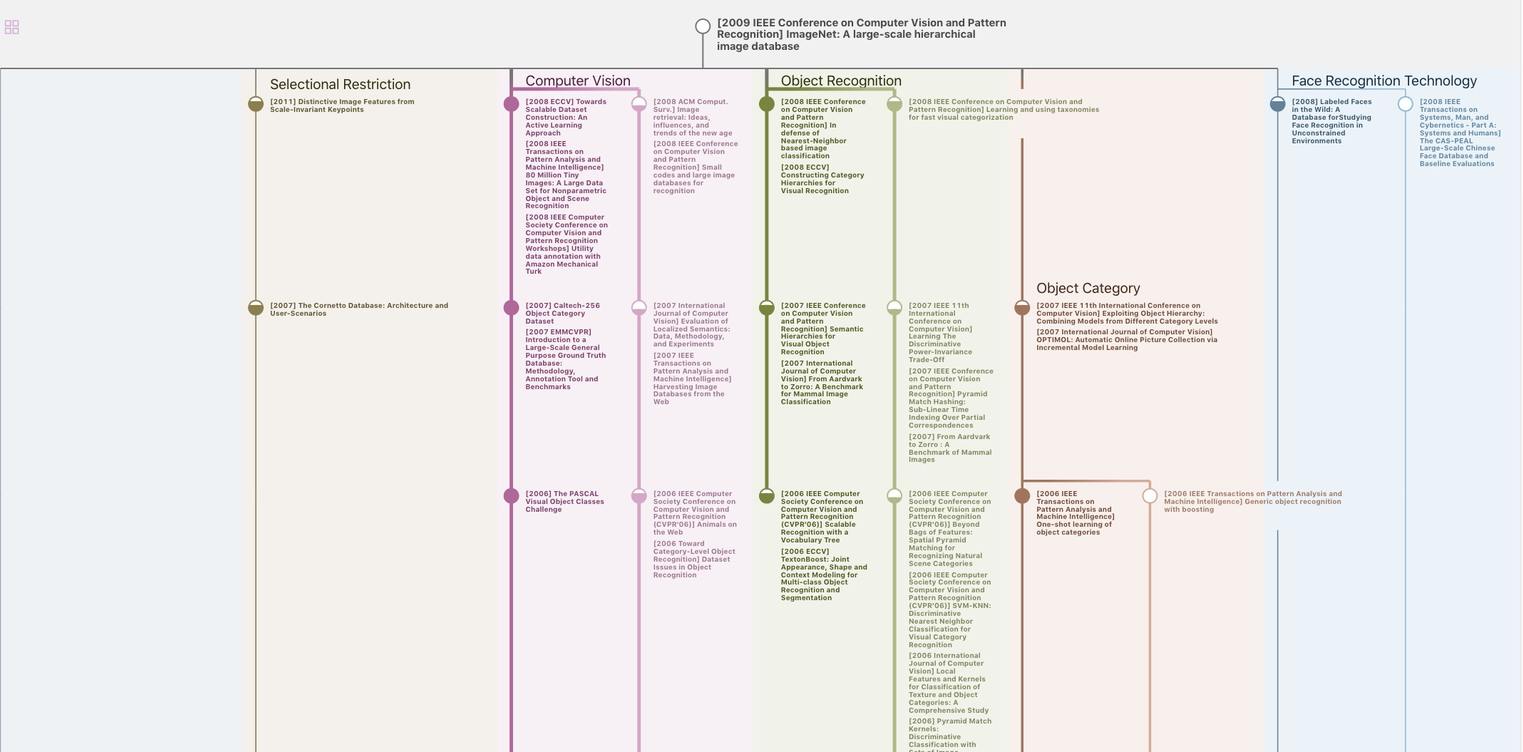
Generate MRT to find the research sequence of this paper
Chat Paper
Summary is being generated by the instructions you defined