Joint forecasting of source-load-price for integrated energy system based on multi-task learning and hybrid attention mechanism
Applied Energy(2024)
摘要
In integrated energy systems (IESs), reliable planning and operation are challenging owing to significant uncertainties in energy production, utilization, and trading. To this end, this paper proposes a multi-task joint forecasting method that enables joint source-load-price forecasting. First, three uncertain variables in an IES, namely, renewable energy, the multi-energy load, and the energy price, were investigated and the complex coupling relationships among them were validated. Second, to cope with the redundant noise resulting from various inputs, multi-channel feature extraction and a hybrid attention mechanism were combined to enable separate extraction and unified fusion of features. Additionally, considering the unique one-dimensional input in the prediction domain, a sequential convolution attention module (SCAM) with a hybrid channel and temporal attention mechanism was proposed to guide multi-channel feature fusion. Finally, facing the challenge of multi-layer coupling information learning, a multi-task learning (MTL) integrated shared layer was designed. Based on the coordinated with MTL, multi-column convolutional neural network, SCAM and long short-term memory network, joint forecasting of source-load-price was realized. The simulation results showed that the average mean absolute percentage error of the proposed model was as low as 4.10% in source-load-price long-term forecasting, while that of winter short-term forecasting could reach 3.14%. In addition, the here proposed model was found to be superior to others in terms of computational efficiency and result stability.
更多查看译文
关键词
Integrated energy system,Joint forecasting,Multi-task learning,Attention mechanism
AI 理解论文
溯源树
样例
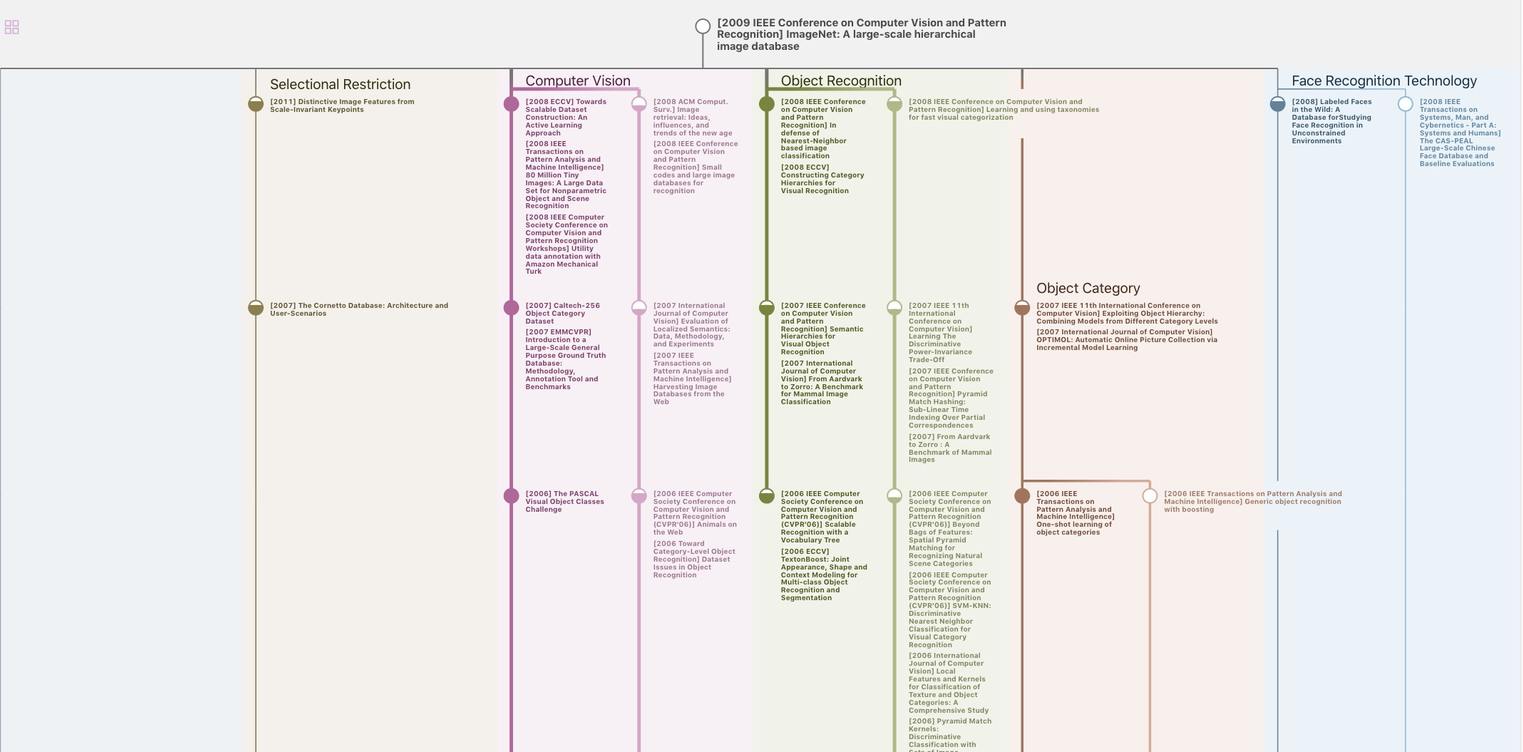
生成溯源树,研究论文发展脉络
Chat Paper
正在生成论文摘要