Novel polynomial Abet data augmentation algorithm with GRU paradigm for nuclear power prediction
Annals of Nuclear Energy(2024)
摘要
In current study innovative approach is presented to improve the accuracy and efficiency of machine learning models on the dataset for each of the 31 US states, solely comprising a single power output column in Terra watt-hours (TWh) spanning from January 2013 to November 2020 by exploiting the knacks of Long Short-Term Memory (LSTM), Gated Recurrent Unit (GRU), hybrid GRU-LSTM, Polynomial Feature Transform (PFT) and Polynomial Abet (PA) data augmentation with GRU for efficacious prediction. Achieving sufficient accuracy, a small univariate dataset of 95 rows was augmented through the PFT method, resulting in a significant reduction in prediction errors. Encouraged by this improvement, a novel algorithm PA data augmentation with GRU is introduced for further enhancing the accuracy, correlation, and computational efficiency of the prediction model. This research not only demonstrates the superior performance of the proposed PA data augmentation method but also highlights the benefit of using 31 individual univariate datasets for each state, allowing comprehensive testing and validation of the novel algorithm, providing compelling evidence of its efficacy in nuclear power prediction. The results from the proposed algorithms are evaluated using Root Mean Square Error (RMSE) and Relative Root Mean Square Error (RRMSE). A quantitative overview of PA and PFT data augmentation techniques’ comparison show the result in terms of accuracy (PA’s 0.464% RRMSE versus PFT’s 1.088 % RRMSE), correlation (PA’s −0.5877 versus PFT’s 0.4892), and computational efficiency (PA’s 14.02 % less computational time then PFT) for the nuclear power prediction model. The research framework introduced in this study, with its focus on utilizing state-specific data for nuclear energy prediction, holds significant implications for governmental decision-making policies. It provides a comprehensive overview of the trends in power generation and can be utilized for both renewable and thermal sources across different states. This information becomes instrumental in allocating funding and setting priorities for states to enhance their generation and transmission infrastructure. Moreover, the research effectively addresses the challenges posed by limited data, offering valuable insights in scenarios where only small datasets are available. The methodological approach and outcomes of this study contribute to the advancement of decision-making processes and policy formulation in the realm of energy governance.
更多查看译文
关键词
Nuclear power prediction,LSTM,Univariate time series,GRU,Hybrid LSTM and GRU,Polynomial feature transform,Novel polynomial abet,Novel data augmentation technique
AI 理解论文
溯源树
样例
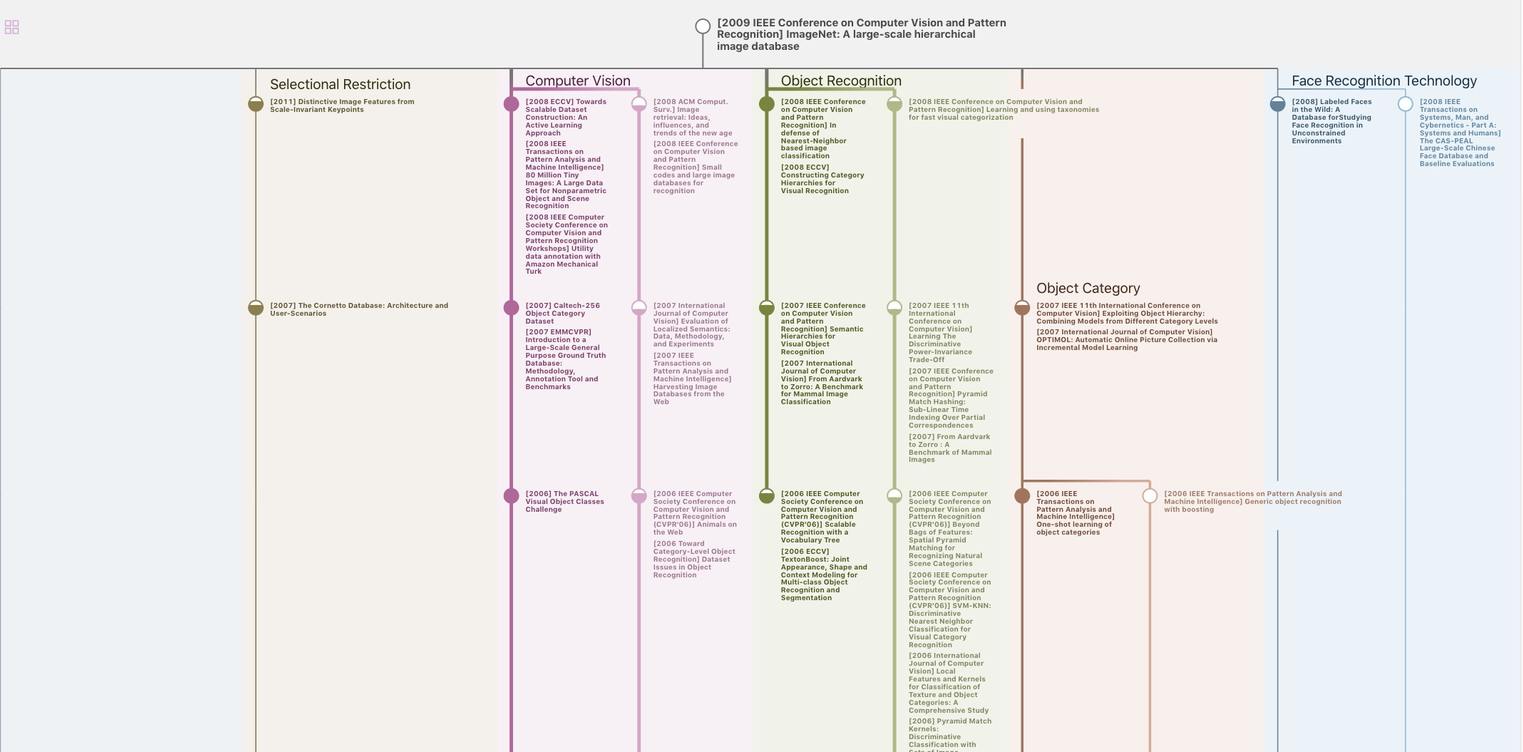
生成溯源树,研究论文发展脉络
Chat Paper
正在生成论文摘要