Intelligent multi-severity nuclear accident identification under transferable operation conditions
ANNALS OF NUCLEAR ENERGY(2024)
摘要
Nuclear power plants (NPPs) have witnessed significant advancements in intelligent accident identification in recent years. However, comprehensive research on fine-grained analysis of accident severity levels has been lacking, thus limiting its practical application in real operating environments. This study proposes a novel intelligent nuclear accident identification method based on the designed dual-branch domain adaptation neural network (DBDANN). The network employs the multiple kernel maximum mean discrepancy (MK-MMD) to measure the feature distribution discrepancy between the source and target domains. Additionally, a deep residual convolutional neural network (DRCNN) is integrated with two separate classifiers with dual-branch design for accident type and severity identification parallelly. The results from three transferrable scenarios for accident identification in nuclear simulation platforms demonstrate the effectiveness of the proposed method in providing fine-grained severity level recognition. Moreover, the proposed method holds promising potential for future intelligent model expansion and practical deployment.
更多查看译文
关键词
Accident identification,Multi -severity,Dual -branch network design,Transferable operation conditions,NPPs
AI 理解论文
溯源树
样例
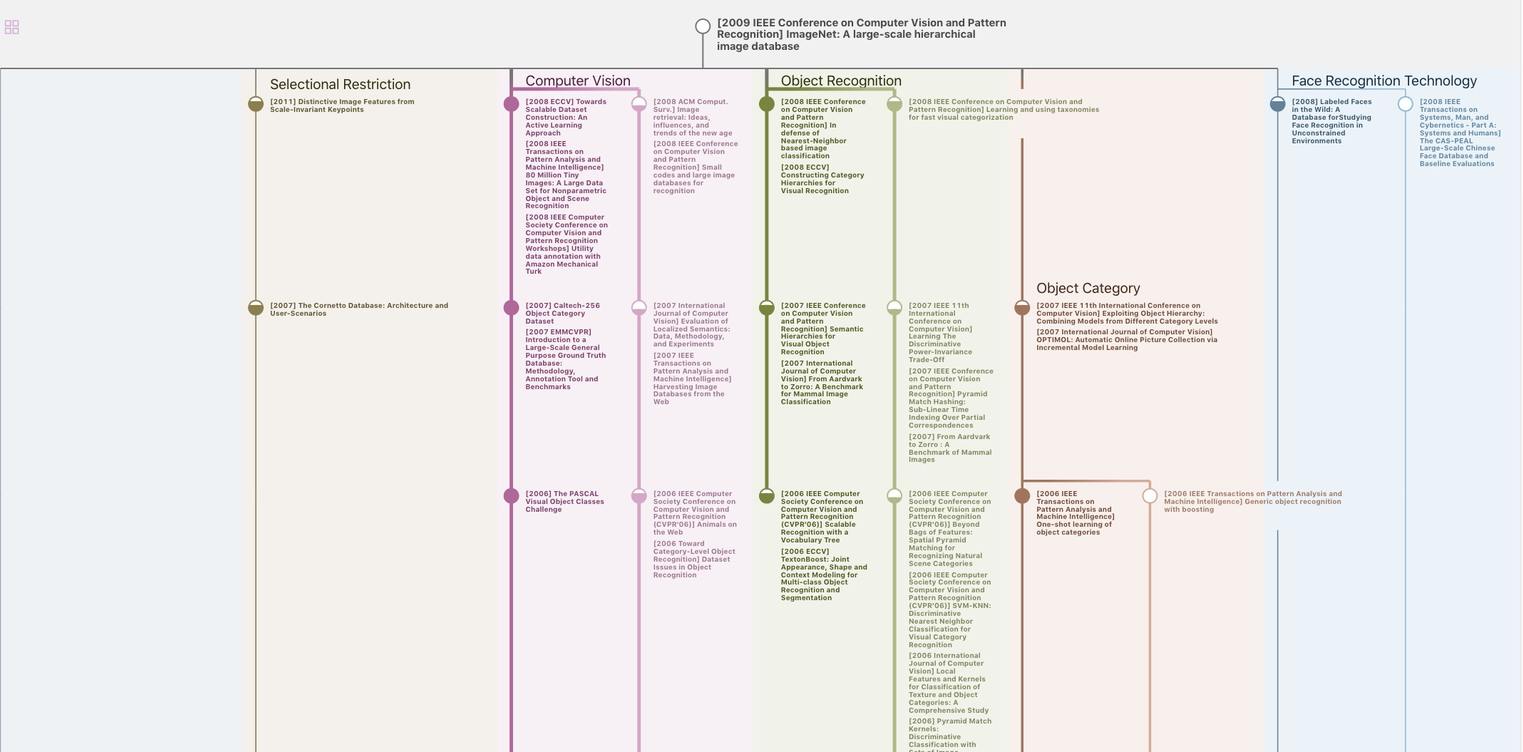
生成溯源树,研究论文发展脉络
Chat Paper
正在生成论文摘要