Prediction of collector flotation performance based on machine learning and quantum chemistry: A case of sulfide minerals
Separation and Purification Technology(2024)
摘要
Flotation, as one of the most important separation technologies in the 21st century, enables the large-scale utilization of mineral resources. The development of high-performance surfactants, especially collectors, is at the core of flotation separation. The evaluation and prediction of collector flotation performance on mineral recovery are prerequisites for its high-efficient development. In this work, we present a novel machine learning (ML) model that can evaluate and predict the recoveries of sulfide minerals (chalcopyrite, galena, pyrite and sphalerite) using the collectors under different flotation conditions (pulp pH, flotation time, collector concentration, etc.). In order to build the model, the features of 116 collectors (electrostatic properties, atomic charges, molecular orbitals, etc.) and 4 sulfide minerals (surface charges, band gap, adsorption energies, etc.) are characterized by quantum chemistry (QC) computation. The features, along with the flotation conditions reported in the literature, were used as input, and the experimental recoveries of four sulfide minerals are the output. Among 116 collectors, 10 randomly selected collectors were employed to refine the model until the mean absolute error (MAE) between the mineral recoveries from experiments and model prediction reaches a minimum of 10.0%. The optimized ML model was proved to successfully predict the flotation performance of 23 new collectors with MAE of 5.2%. We can conclude that this QC-ML model will offer great assistance in the future high-throughput screening and design of flotation reagents.
更多查看译文
关键词
Flotation,Machine learning,Quantum chemistry,Sulfide mineral,Surfactant development,Performance prediction
AI 理解论文
溯源树
样例
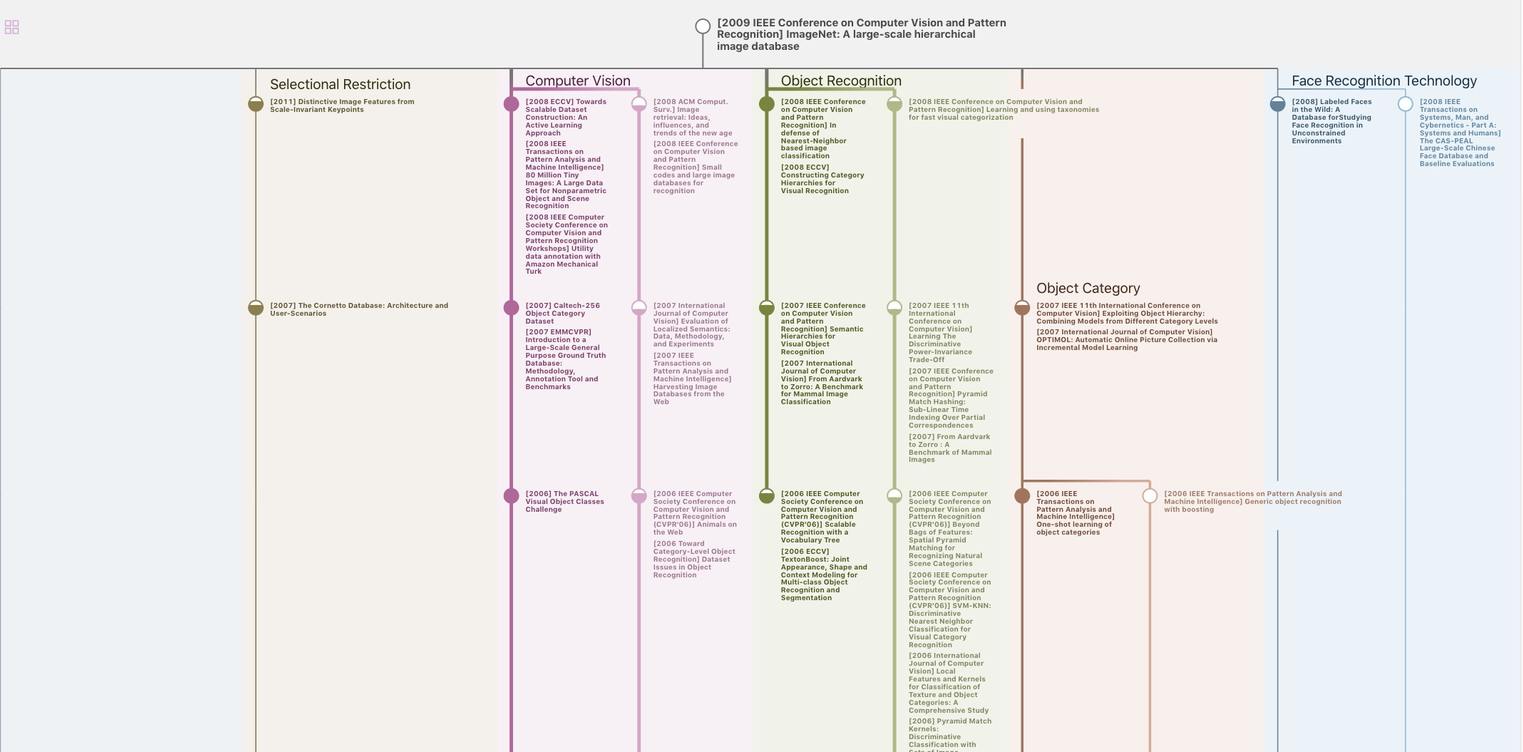
生成溯源树,研究论文发展脉络
Chat Paper
正在生成论文摘要