DLFSI: A deep learning static fluid-structure interaction model for hydrodynamic-structural optimization of composite tidal turbine blade
RENEWABLE ENERGY(2024)
摘要
Horizontal axis tidal turbines (HATT) conversion of ocean tidal waves into electricity represents a promising source of clean and sustainable energy. However, the widespread adoption of these turbines has been hindered by persistent challenges, primarily stemming from the high costs associated with their construction and maintenance; and efficient conversion of tidal energy. Addressing these challenges is paramount for propelling tidal turbine technology and ensuring its economic viability. This study focuses on the efficient conversion of tidal energy into electricity by optimization of composite material turbine blades which is a complex problem that spans multiple physical domains, including hydrodynamics (the study of water flow) and structural mechanics (the study of material behavior under loads). To tackle these multifaceted challenges, we introduce an innovative DLFSI (Deep learning fluid -structure interaction) model which represents a groundbreaking approach to predict and optimize the hydrodynamic and structural performance of tidal turbine blades. DLFSI leverages the power of convolutional neural networks (CNN) to recognize intricate geometric features of turbine blades rapidly and accurately. By seamlessly integrating the blade element momentum (BEM) theory and finite element method (FEM), the DLFSI model facilitates comprehensive predictions of how composite blades will perform in realworld conditions. With this approach, we have achieved substantial improvements in critical performance metrics such as the power coefficient (a measure of energy conversion efficiency) and the maximum equivalent stress (a key indicator of structural integrity). The innovative DLFSI model presented in this study holds the potential for practical application within the realm of tidal turbine design and is poised to catalyze the sustainable progression of renewable energy technologies.
更多查看译文
关键词
Horizontal axis tidal turbines (HATT),Composite blade,Multi-objective optimization,Convolutional neural networks (CNN),Blade element momentum (BEM),Finite element method (FEM)
AI 理解论文
溯源树
样例
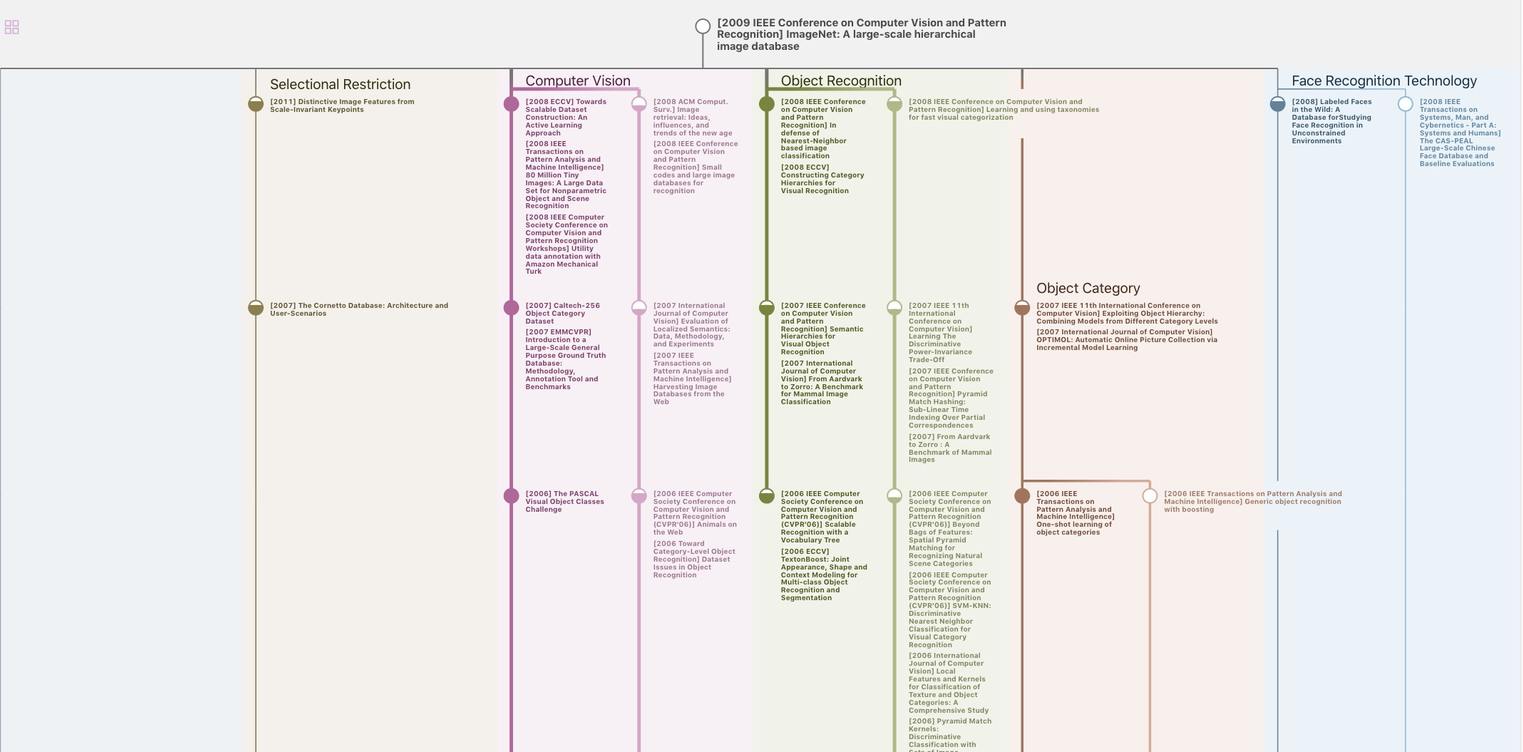
生成溯源树,研究论文发展脉络
Chat Paper
正在生成论文摘要