FA4SANS-GAN: A Novel Machine Learning Generative Adversarial Network to Further Understand Ophthalmic Changes in Spaceflight Associated Neuro-Ocular Syndrome (SANS)
Ophthalmology Science(2024)
摘要
Purpose
To provide automated system for synthesizing fluorescein angiography (FA) images from color fundus (CF) photographs for averting risks associated with fluorescein dye and extend its future application to Space-associated neuro-ocular syndrome (SANS) detection in spaceflight where resources are limited.
Design
Development and validation of a novel conditional generative adversarial network (GAN) trained on limited amount of fluorescein angiography and color fundus images with diabetic retinopathy and control cases.
Participants
Color fundus and fluorescein angiography paired images for unique patients were collected from a publicly available study.
Methods
FA4SANS-GAN was trained to generate fluorescein angiography images from color fundus photographs using two multi-scale generators coupled with two patch-GAN discriminators. 850 CF and FA images were utilized for training by augmenting images from 17 unique patients. The model was evaluated on 56 fluorescein images collected from 14 unique patients. In addition, it was compared with three other GAN architectures trained on the same dataset. Furthermore, we test the robustness of the models against acquisition noise and retaining structural information when introduced to artificially created biological markers.
Main Outcome Measure
For GAN synthesis metric Fréchet Inception Distance (FID) and Kernel Inception Distance (KID). Also, two one-sided tests (TOST) based on Welch's t-test for measuring statistical significance.
Results
On test FA images, mean FID for FA4SANS-GAN was 39.8 (SD 9.9), which is better than GANgio model’s mean of 43.2 (SD 13.7), Pix2PixHD’s mean of 57.3 (SD 11.5) and Pix2Pix’s mean of 67.5 (SD 11.7). Similarly for KID, FA4SANS-GAN achieved mean of 0.00278 (SD 0.00167) which is better than other three model’s mean KID of 0.00303 (SD 0.00216), 0.00609 (SD 0.00238), 0.00784 (SD 0.00218). For TOST measurement, FA4SANS-GAN has proven to be statistically significant vs. GANgio (p = 0.006); vs Pix2PixHD (p < 0.00001); and vs. Pix2Pix (p < 0.00001).
Conclusion
Our study has shown FA4SANS-GAN to be statistically significant for two GAN synthesis metrics. Moreover, it is robust against acquisition noise, and can retain clear biological markers compared to the other three GAN architectures. This deployment of this model can be crucial in International Space Station (ISS) for detecting Spaceflight-associated neuro-ocular syndrome (SANS).
更多查看译文
关键词
Spaceflight Associated Neuro-Ocular Syndrome,Generative Adversarial Networks,Ophthalmic Imaging,Space Medicine,Astronaut
AI 理解论文
溯源树
样例
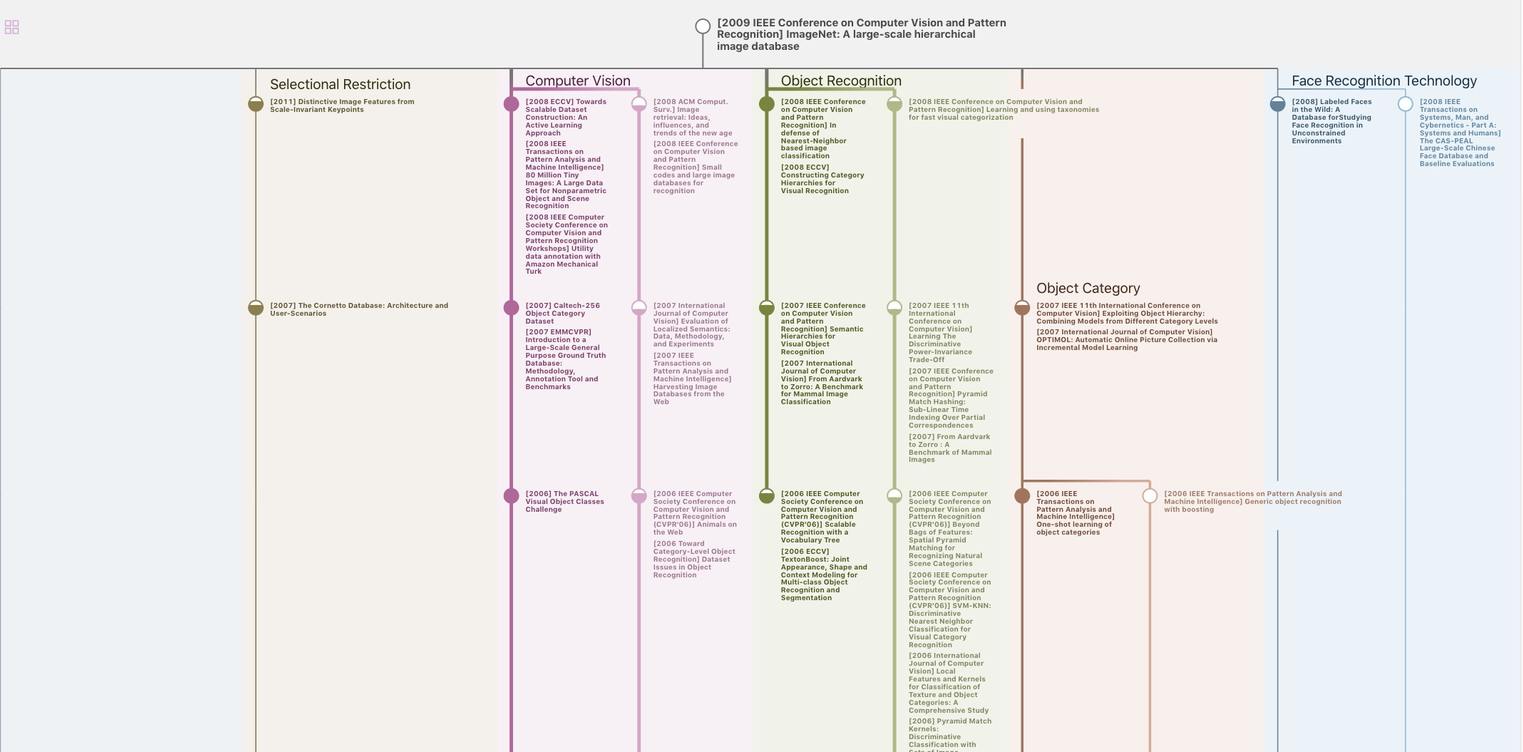
生成溯源树,研究论文发展脉络
Chat Paper
正在生成论文摘要