Multiview adaptive attention pooling for image-text retrieval
KNOWLEDGE-BASED SYSTEMS(2024)
摘要
Image-Text Retrieval (ITR) aims to bridge the heterogeneity gap between image and text and establish the retrieval ability between the two modalities. There is a large intraclass difference in visual language data; that is, the content of an image can be described from different views. Representing images and texts as single embedded features to measure similarity makes it difficult to capture the diversity and fine-grained information of modal features. Previous methods use cumbersome dilated convolution structures or stack multiple feature pooling operators to perform multiview learning of images, and then take the view with the highest similarity score to the text as the alignment result. This may lead to two problems: (1) The model becomes complex, and has poor scalability and limited feature learning ability; (2) The matching strategy with the highest similarity score may have the problem that text view features deliberately emphasize a certain area, resulting in suboptimal matching. Therefore, we propose a multiview adaptive attention pooling (MVAAP) network, a simpler and more effective multiview global feature embedding method. Specifically, MVAAP learns the query for each view, extracts the salient image region of the view through an adaptive attention mechanism, and generates an optimal pooling strategy to aggregate it into the global feature of the view. Beyond that, we introduce multiview embedding of the text branch and consider the response between different views to improve the generalization ability of the model. Plenty of experiments on the two mainstream cross -modal datasets of MS-COCO and Flickr30K prove the accuracy and superiority of the method.
更多查看译文
关键词
Image-text retrieval,Visual semantic embedding,Feature pooling strategy,Multiview embedding
AI 理解论文
溯源树
样例
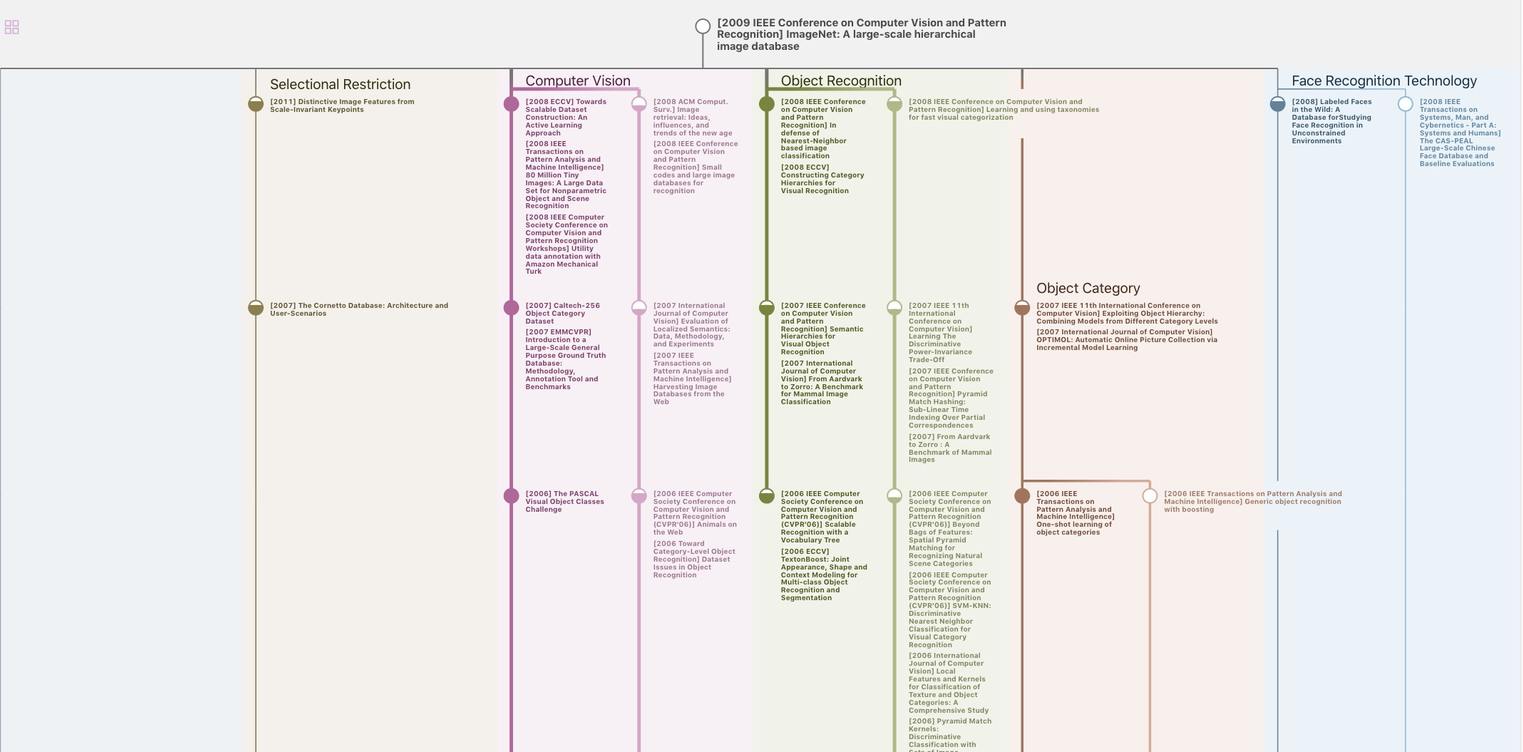
生成溯源树,研究论文发展脉络
Chat Paper
正在生成论文摘要