System energy and band gap prediction of titanium dioxide based on machine learning
JOURNAL OF MOLECULAR STRUCTURE(2024)
摘要
Fast and accurate prediction of certain properties from a given structure, as well as pinpointing key factors influencing particular properties, is crucial for the targeted design of new compounds with desired properties or crystal phase compositions. In the current work, a total of 2,419,200 Gaussian-type structure descriptors (GTSDs) were utilized as a bridge to establish artificial neural network (ANN) potential models between 1400 titanium dioxide (TiO2) structures and their corresponding system energies and band gaps. After training, the ANN showed high accuracy in predicting energy, with Rtrain of 0.985, Rtest of 0.967, and RMSE of 0.0489. However, for the band gap values with intense data fluctuation, the Rtrain, Rtest, and RMSE changed to 0.864, 0.832, and 0.1304, respectively, indicating that the ANN's predictive ability for drastically changing data is limited. Compared with ab initio calculations, using ANN model can increase the calculation speed by 4-6 orders of magnitude. Furthermore, we identified the most significant features affecting the system energy and band gap values as the shape and distortion of [TiOx] polyhedra through the random forest model. Our work aims to provide a new toolkit and research ideas for accelerating the development of new materials.
更多查看译文
关键词
Titanium dioxide,Photocatalyst,Artificial neural network,Property prediction
AI 理解论文
溯源树
样例
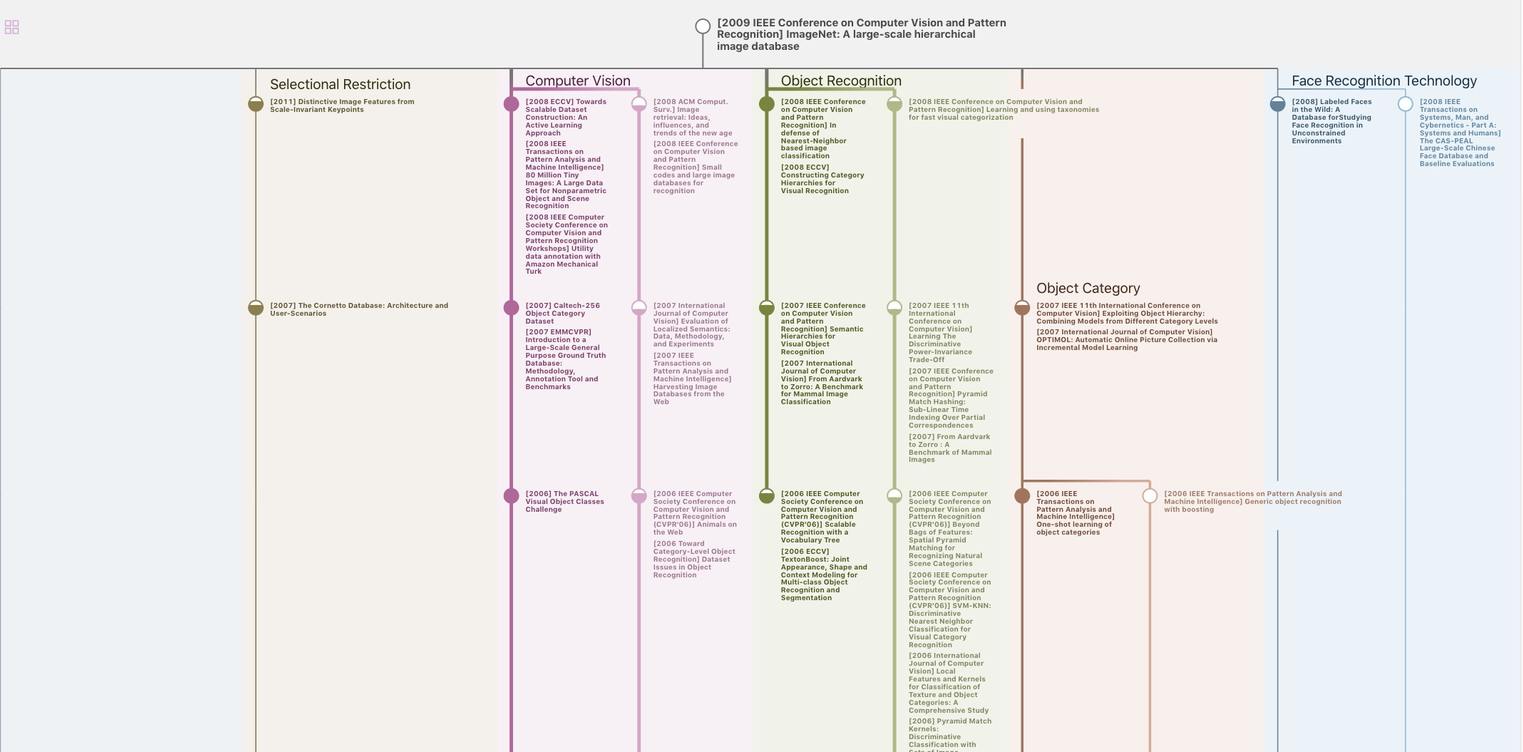
生成溯源树,研究论文发展脉络
Chat Paper
正在生成论文摘要