Prediction of the energetics of stable self-interstitial atoms at tungsten grain boundaries via machine learning
JOURNAL OF NUCLEAR MATERIALS(2024)
摘要
The stable sites of self-interstitial atoms (SIAs) at grain boundaries (GBs) and corresponding energetics are fundamental to explore both the SIA-GB interaction and the effects of SIA segregation on the role of GBs in microstructural evolution under irradiation. In this study, by combing the support vector machine (SVM) model suitable for handling small samples with the differential evolution (DE) algorithm designed for global optimization, a machine learning (ML) framework for modeling and predicting the formation energies of stable SIAs (EfSIAmin) at tungsten GBs was established. The stable SIA site was related to the atomic site with maximum atomic volume in the pure GB, and the feature variables for constructing descriptors were thus extracted. Meanwhile, three GB characteristics of tilt angle, GB energy and interplanar spacing were also selected to construct the descriptors. The optimal descriptors consisting of both the local structure information and the GB characteristics were determined by the property-feature correlation analysis, wrapper and enumeration methods. The model from 7-fold and leave-one-out cross validation has mean absolute percentage error (MAPE) close to 6.0 % and square correlation coefficient (R2) close to 0.9, showing a promising prediction capability for EfSIAmin out of the training set based on the currently constructed descriptors. The performance comparison with other ML methods reflects the effectiveness of our constructed descriptors and the high efficiency of SVM in dealing with small-size datasets. The model transferability was further verified by its application to alpha-iron and molybdenum based systems. The present work establishes the relationship between the energetics of the stable SIA and the GB structure via ML, and shows great prospect in the application of ML methods on modeling GB-relevant defect properties.
更多查看译文
关键词
Radiation damage,Self-interstitial atom,Grain boundary,Differential evolution,Support vector machine
AI 理解论文
溯源树
样例
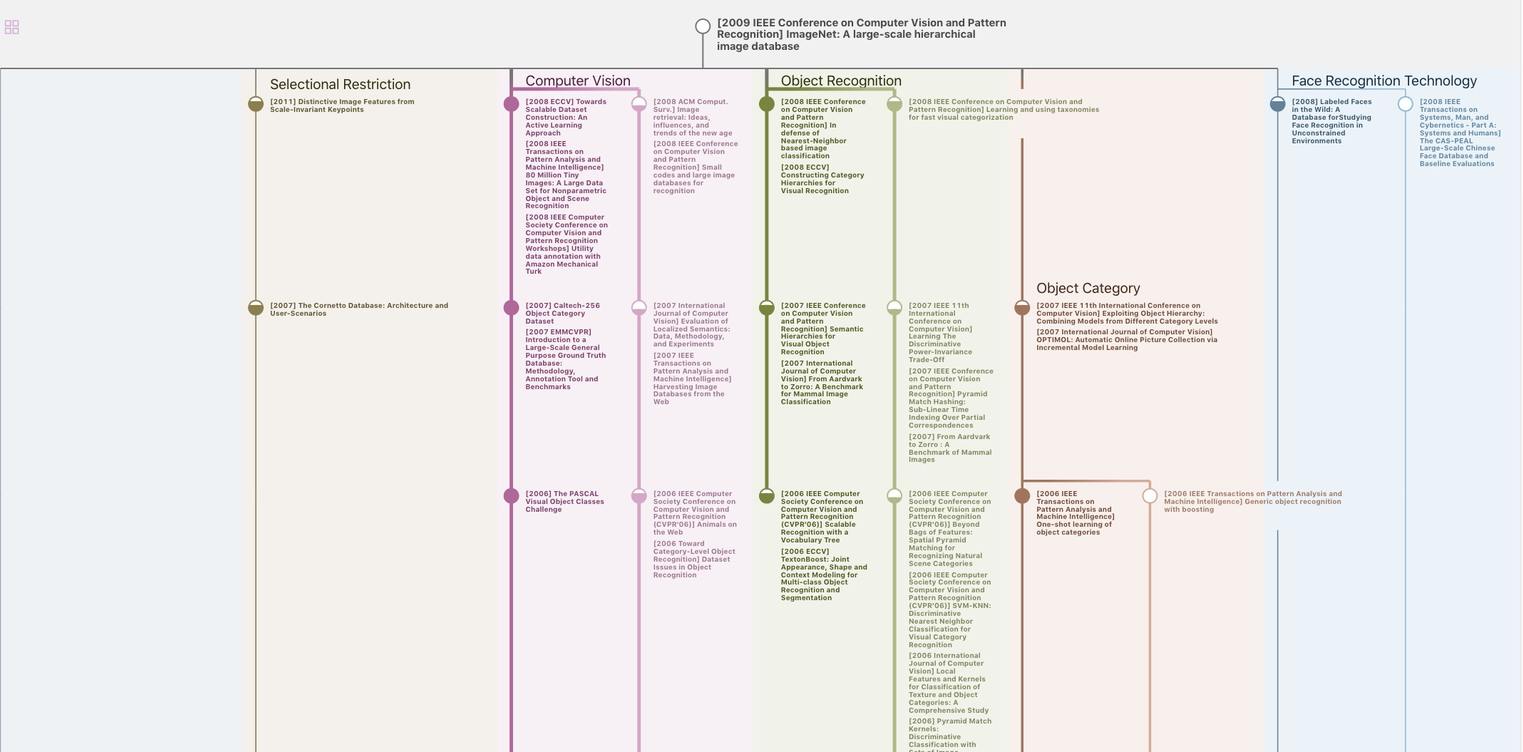
生成溯源树,研究论文发展脉络
Chat Paper
正在生成论文摘要