A fast gradient convolution kernel compensation method for surface electromyogram decomposition
Journal of Electromyography and Kinesiology(2024)
摘要
Decomposition of EMG signals provides the decoding of motor unit (MU) discharge timings. In this study, we propose a fast gradient convolution kernel compensation (fgCKC) decomposition algorithm for high-density surface EMG decomposition and apply it to an offline and real-time estimation of MU spike trains. We modified the calculation of the cross-correlation vectors to improve the calculation efficiency of the gradient convolution kernel compensation (gCKC) algorithm. Specifically, the new fgCKC algorithm considers the past gradient in addition to the current gradient. Furthermore, the EMG signals are divided by sliding windows to simulate real-time decomposition, and the proposed algorithm was validated on simulated and experimental signals. In the offline decomposition, fgCKC has the same robustness as gCKC, with sensitivity differences of 2.6 ± 1.3 % averaged across all trials and subjects. Nevertheless, depending on the number of MUs and the signal-to-noise ratio of signals, fgCKC is approximately 3 times faster than gCKC. In the real-time part, the processing only needed 240 ms average per window of EMG signals on a regular personal computer (IIntel(R) Core(TM) i5-12490F 3 GHz, 16 GB memory). These results indicate that fgCKC achieves real-time decomposition by significantly reducing processing time, providing more possibilities for non-invasive neuronal behavior research.
更多查看译文
关键词
Motor unit,Fast gradient convolution kernel compensation,Simulated signals,Offline,Real-time
AI 理解论文
溯源树
样例
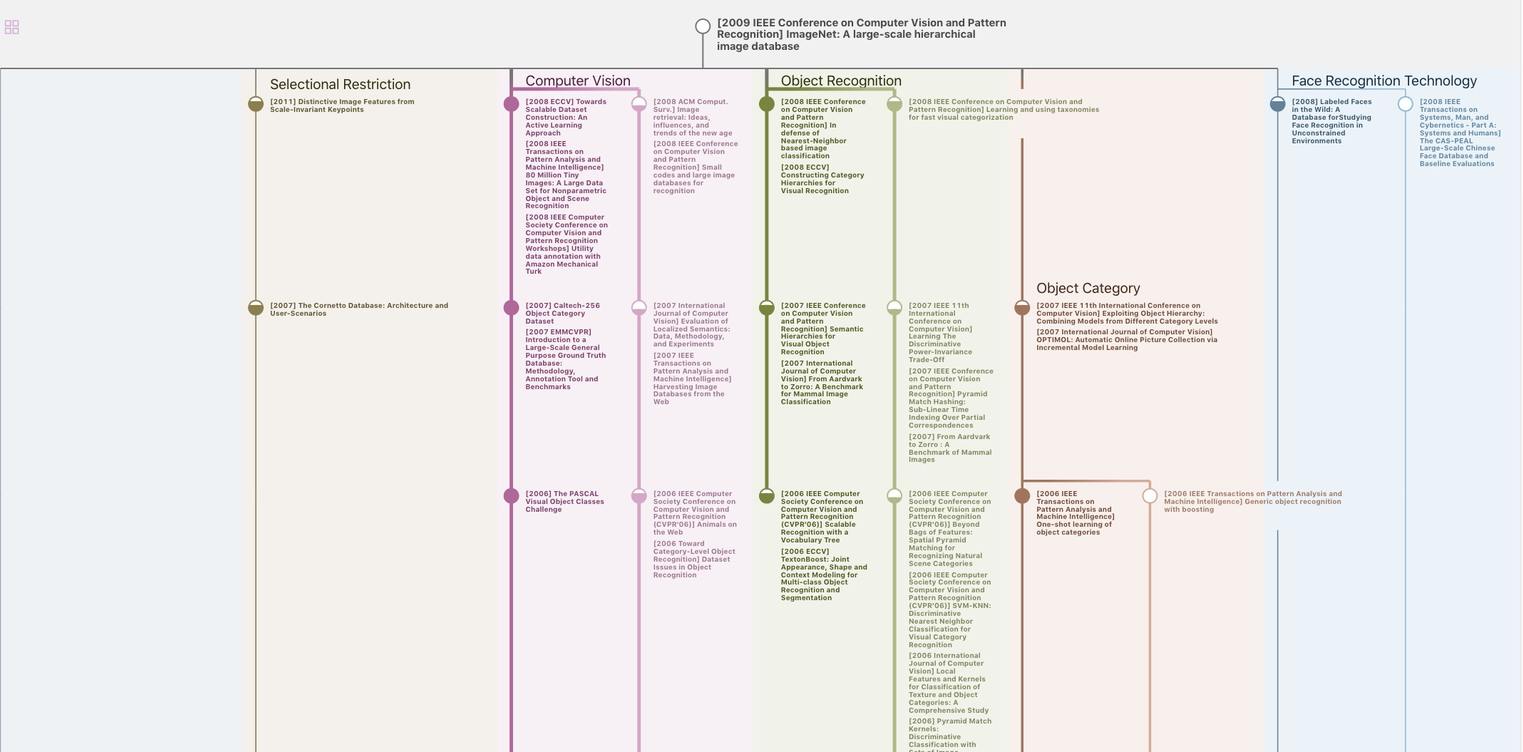
生成溯源树,研究论文发展脉络
Chat Paper
正在生成论文摘要