Prediction of critical temperature of binary refrigerant mixtures by neural network
International Journal of Refrigeration(2024)
摘要
Critical temperature (Tc) is an important property of refrigerant mixtures. In this study, a neural network-based model is established to predict the Tc of binary mixtures from the molar fraction and molecular structure of their components. First, graph neural network (GNN) is applied to generate the representation of the molecular structure of single component. The generated representation is added according to the molar fraction as the input of the proposed model. In order to embed the prior knowledge, pre-trained parameters are reused in the proposed model to accelerate convergence and improve accuracy. A dataset that contains 66 binary mixtures and 505 data points is collected from reference to adjust the remaining model parameters. The performance of the obtained model is comprehensively evaluated and it shows good prediction accuracy and generalization ability. The average absolute relative deviation (AARD) in the total dataset is 0.80%. For most of the mixtures, the AARD is less than 1%. Finally, the proposed model is compared with three empirical methods and the equation of state (EOS)-based method in REFPROP, and the model in this study show satisfactory result and significant improved accuracy for some mixture types.
更多查看译文
关键词
Binary refrigerant mixtures,Critical temperature,Neural network,Transfer learning
AI 理解论文
溯源树
样例
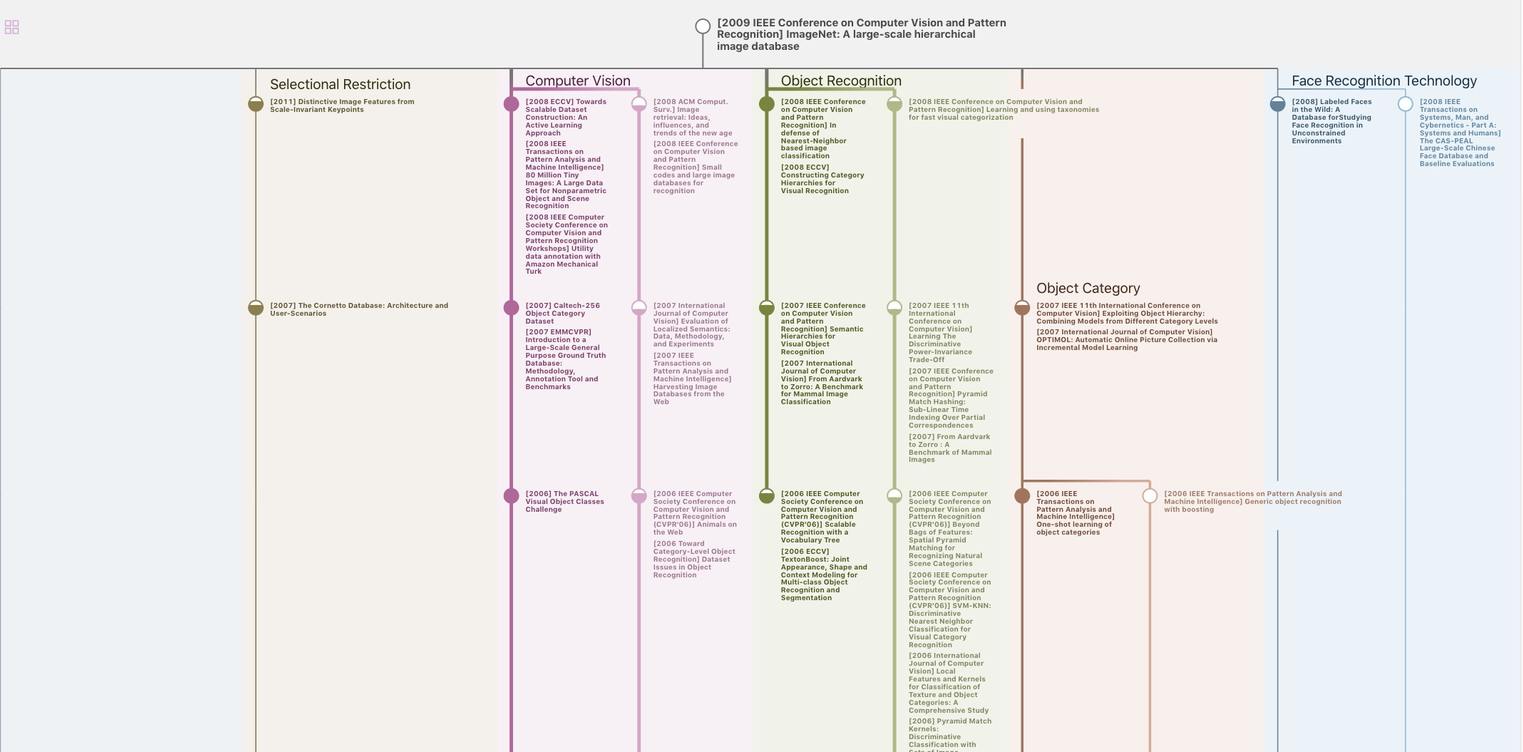
生成溯源树,研究论文发展脉络
Chat Paper
正在生成论文摘要