Hierarchical relationship modeling in multi-agent reinforcement learning for mixed cooperative-competitive environments
INFORMATION FUSION(2024)
摘要
In multi-agent reinforcement learning (MARL), information fusion through relationship modeling can effectively learn behavior strategies. However, the high dynamics among heterogeneous interactive agents in mixed cooperative-competitive environments pose difficulties for relational modeling. Traditional MARL solutions concatenate all agents' states based on the global relationship, which is unrealistic and unscalable under largescale conditions. Other methods fuse local information by modeling neighbor relationships, but local features lead to suboptimal strategies. From this perspective, we propose a novel relational information fusion approach for mixed tasks to fuse local and global features by modeling heterogeneous relationships through a hierarchical graph. During training, remote agents' global features are fused through second-order graph representation to help strategy optimization. During decision making, the practicality and scalability of strategy are improved by fusing neighbor agents' local features through first-order graph representation. Our approach consistently outperforms the state-of-the-art MARL methods in several multi-agent tasks, such as the Predator-Prey and Soccer Games. In particular, it achieves an 83.7% win rate, which is 11.5% higher than baselines in the 4 vs. 4 Soccer Game, and can scale from 4 vs. 4 to 13 vs. 13 in the Predator-Prey Game while maintaining good performance.
更多查看译文
关键词
Multi-agent reinforcement learning,Mixed cooperative-competitive environment,Graph neural networks,Relationship modeling
AI 理解论文
溯源树
样例
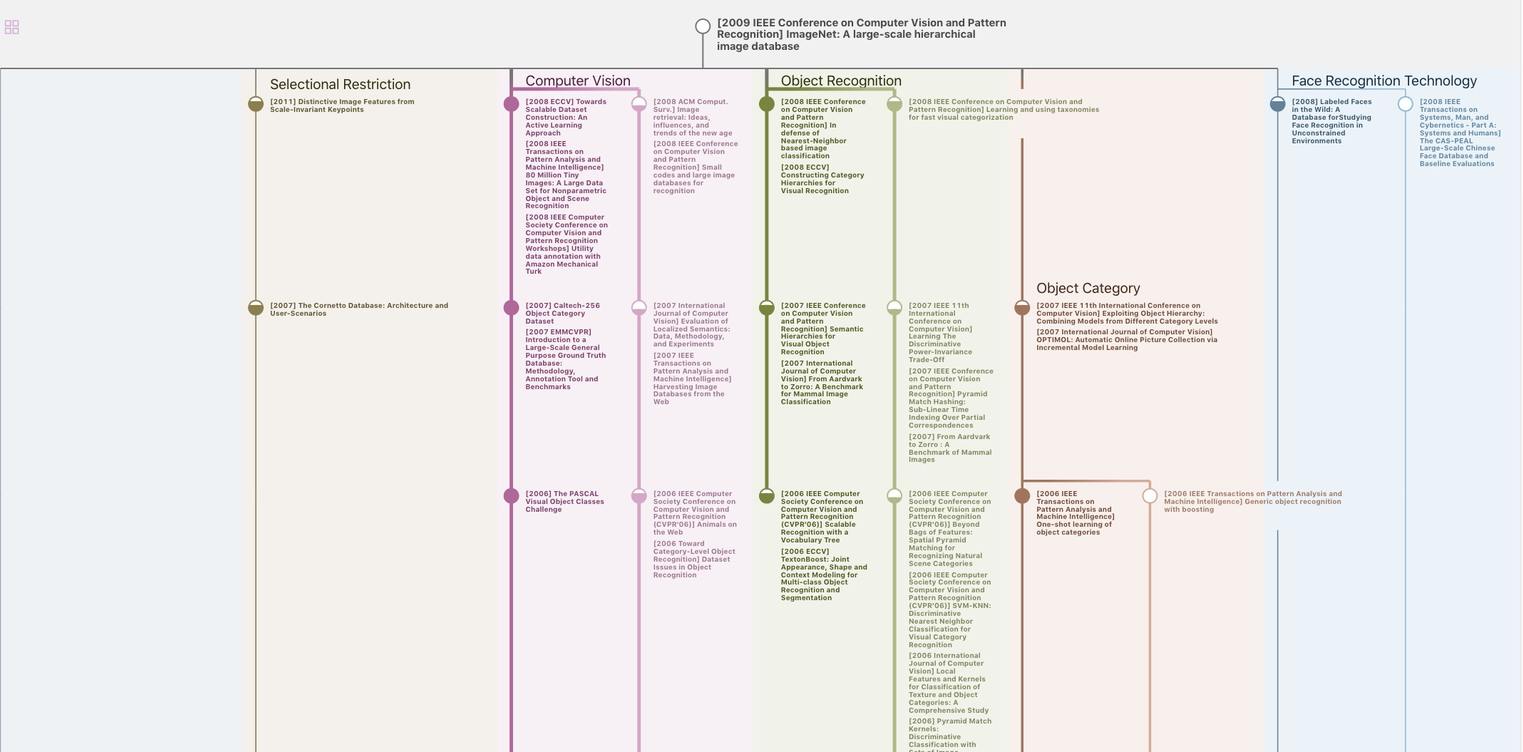
生成溯源树,研究论文发展脉络
Chat Paper
正在生成论文摘要