Peanut origin traceability: A hybrid neural network combining an electronic nose system and a hyperspectral system
Food Chemistry(2024)
摘要
Peanuts, sourced from various regions, exhibit noticeable differences in quality owing to the impact of their natural environments. This study proposes a fast and nondestructive detection method to identify peanut quality by combining an electronic nose system with a hyperspectral system. First, the electronic nose and hyperspectral systems are used to gather gas and spectral information from peanuts. Second, a module for extracting gas and spectral information is designed, combining the lightweight multi-head transposed attention mechanism (LMTA) and convolutional computation. The fusion of gas and spectral information is achieved through matrix combination and lightweight convolution. A hybrid neural network, named UnitFormer, is designed based on the information extraction and fusion processes. UnitFormer demonstrates an accuracy of 99.06 %, a precision of 99.12 %, and a recall of 99.05 %. In conclusion, UnitFormer effectively distinguishes quality differences among peanuts from various regions, offering an effective technological solution for quality supervision in the food market.
更多查看译文
关键词
Peanut quality determination,Nondestructive testing,Information fusion,Deep learning
AI 理解论文
溯源树
样例
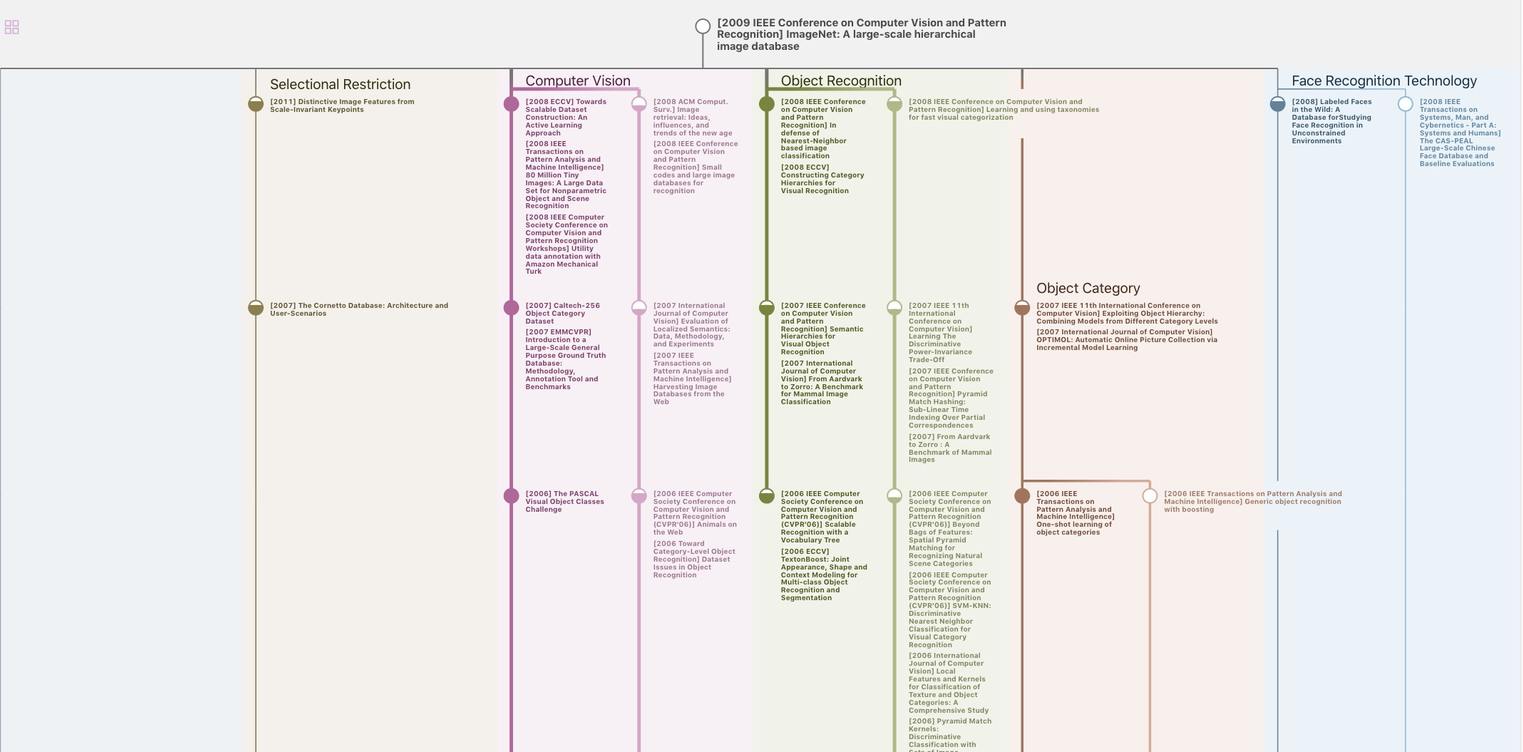
生成溯源树,研究论文发展脉络
Chat Paper
正在生成论文摘要