Evaluating practical adversarial robustness of fault diagnosis systems via spectrogram-aware ensemble method
ENGINEERING APPLICATIONS OF ARTIFICIAL INTELLIGENCE(2024)
摘要
While machine learning models have shown superior performance in fault diagnosis systems, researchers have revealed their vulnerability to subtle noises generated by adversarial attacks. Given that this vulnerability can lead to misdiagnosis or unnecessary maintenance, the assessment of the practical robustness of fault diagnosis models is crucial for their deployment and use in real -world scenarios. However, research on the practical adversarial robustness of fault diagnosis models remains limited. In this work, we present a comprehensive analysis on rotating machinery diagnostics and discover that existing attacks often over -estimate the robustness of these models in practical settings. In order to precisely estimate the practical robustness of models, we propose a novel method that unveils the hidden risks of fault diagnosis models by manipulating the spectrum of signal frequencies-an area that has been rarely explored in the domain of adversarial attacks. Our proposed attack, Spectrogram -Aware Ensemble Method (SAEM), the hidden vulnerability of fault diagnosis systems through achieving a higher attack performance in practical black -box settings. Through experiments, we reveal the potential dangers of employing non -robust fault diagnosis models in real -world applications and suggest directions for future research in industrial applications.
更多查看译文
关键词
Bearing fault diagnosis system,Adversarial attack,Spectrogram
AI 理解论文
溯源树
样例
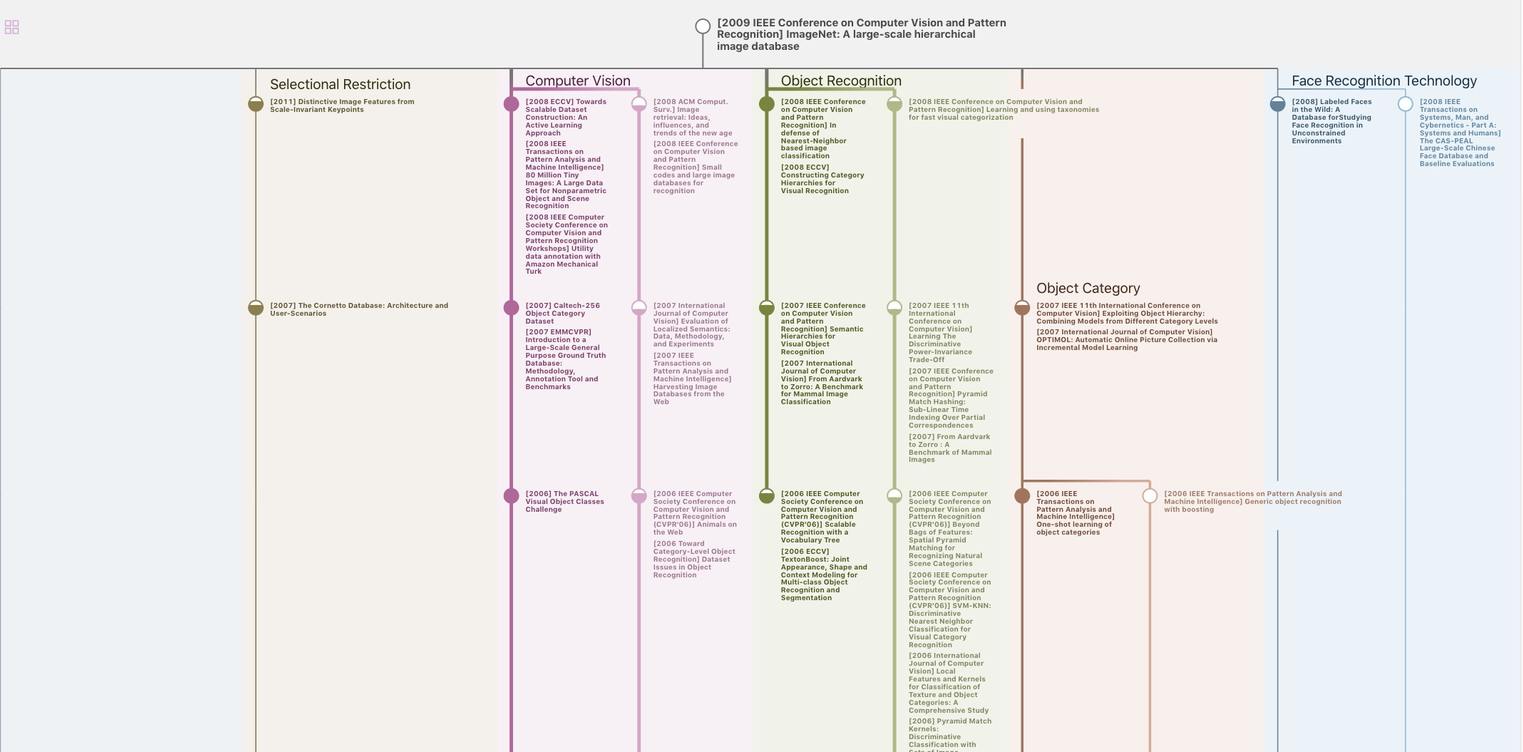
生成溯源树,研究论文发展脉络
Chat Paper
正在生成论文摘要