Parallelized variational quantum classifier with shallow QRAM circuit
Quantum Information Processing(2024)
摘要
In this paper, we present a novel quantum variational circuit, harnessing the capabilities of a pre-determined quantum random access memory (QRAM) circuit to enhance machine learning tasks. Our approach enables parallel training on entire datasets, facilitated by QRAM’s streamlined data loading onto qubits through probability amplitudes. This leads to a logarithmic reduction in the qubit width (number of qubits) concerning data dimension and dataset size. Our model is adaptable to various loss functions, rendering it suitable for binary and multi-class classification tasks. To validate our methodology, we conducted numerical simulations using well-established benchmark datasets, Iris and handwritten digits, with the Pennylane platform. Impressively, our approach yielded high classification accuracy in these illustrations. This work demonstrates promising potential for quantum machine learning applications, especially when dealing with large datasets.
更多查看译文
关键词
Quantum random access memory,Variational quantum circuit,Quantum classifier,Multi-class classification
AI 理解论文
溯源树
样例
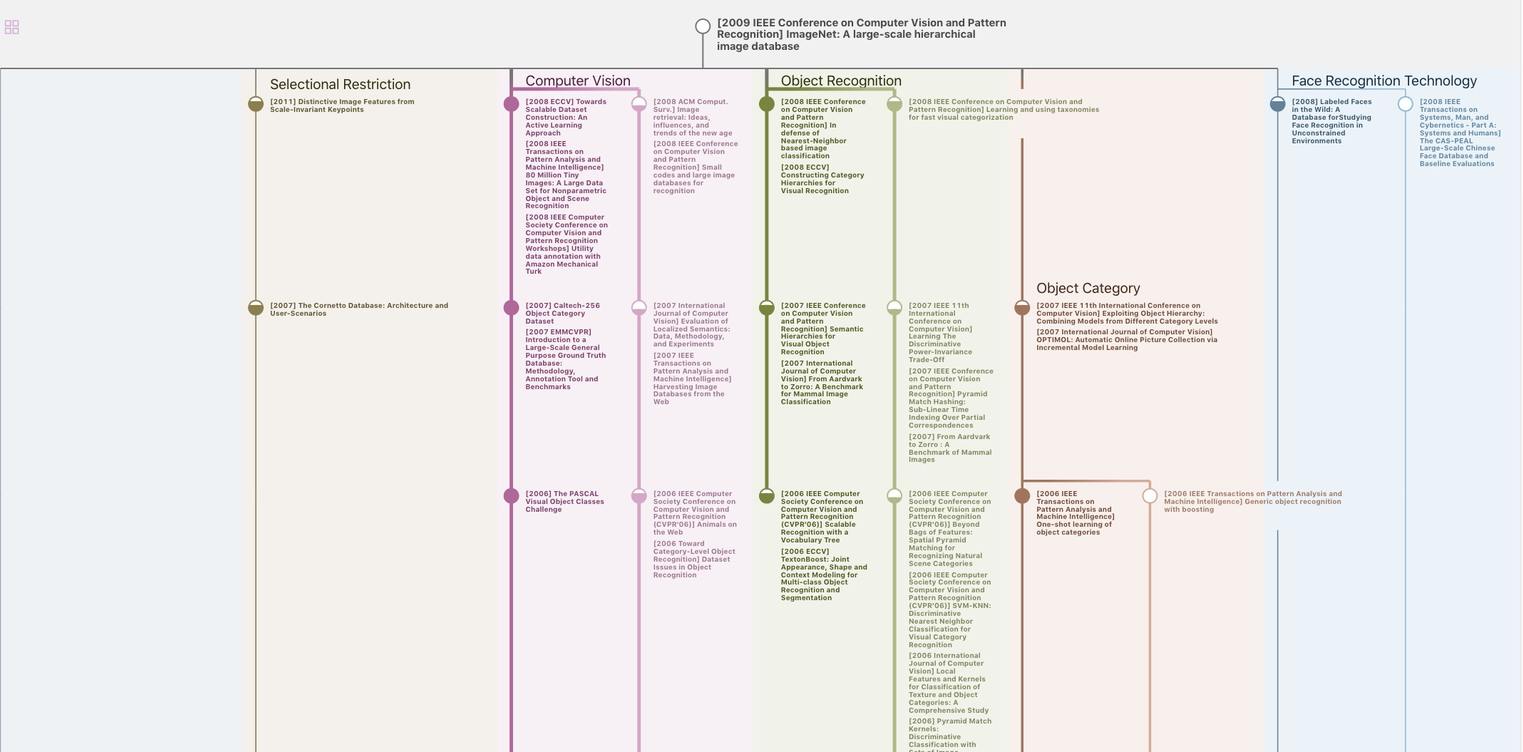
生成溯源树,研究论文发展脉络
Chat Paper
正在生成论文摘要