Boosting manta rays foraging optimizer by trigonometry operators: a case study on medical dataset
Neural Computing and Applications(2024)
摘要
The selection of attributes has become a crucial research focus in the domains of pattern recognition, machine learning, and big data analysis. In essence, the contemporary challenge revolves around reducing dimensionality while maintaining both a quick response time and improved classification performance. Metaheuristics algorithms (MAs) have emerged as pivotal tools in addressing this issue. Firstly, the problem of attribute selection was approached using the manta ray foraging optimization (MRFO) approach, but the majority of MAs suffer from a problem of convergence toward local minima. To mitigate this challenge, an enhanced variant of MRFO, known as MRFOSCA, employs trigonometric operators inspired by the sine cosine algorithm (SCA) to tackle the feature selection problem. The k-nearest neighbor (k-NN) technique is employed for feature-set selection. Additionally, the statistical significance of the proposed algorithms is assessed using the nonparametric Wilcoxon’s rank-sum test at a 5
更多查看译文
关键词
Manta ray foraging optimization (MRFO),Methaheuristics,Feature selection,Dimensionality reduction,Sine cosine algorithm (SCA)
AI 理解论文
溯源树
样例
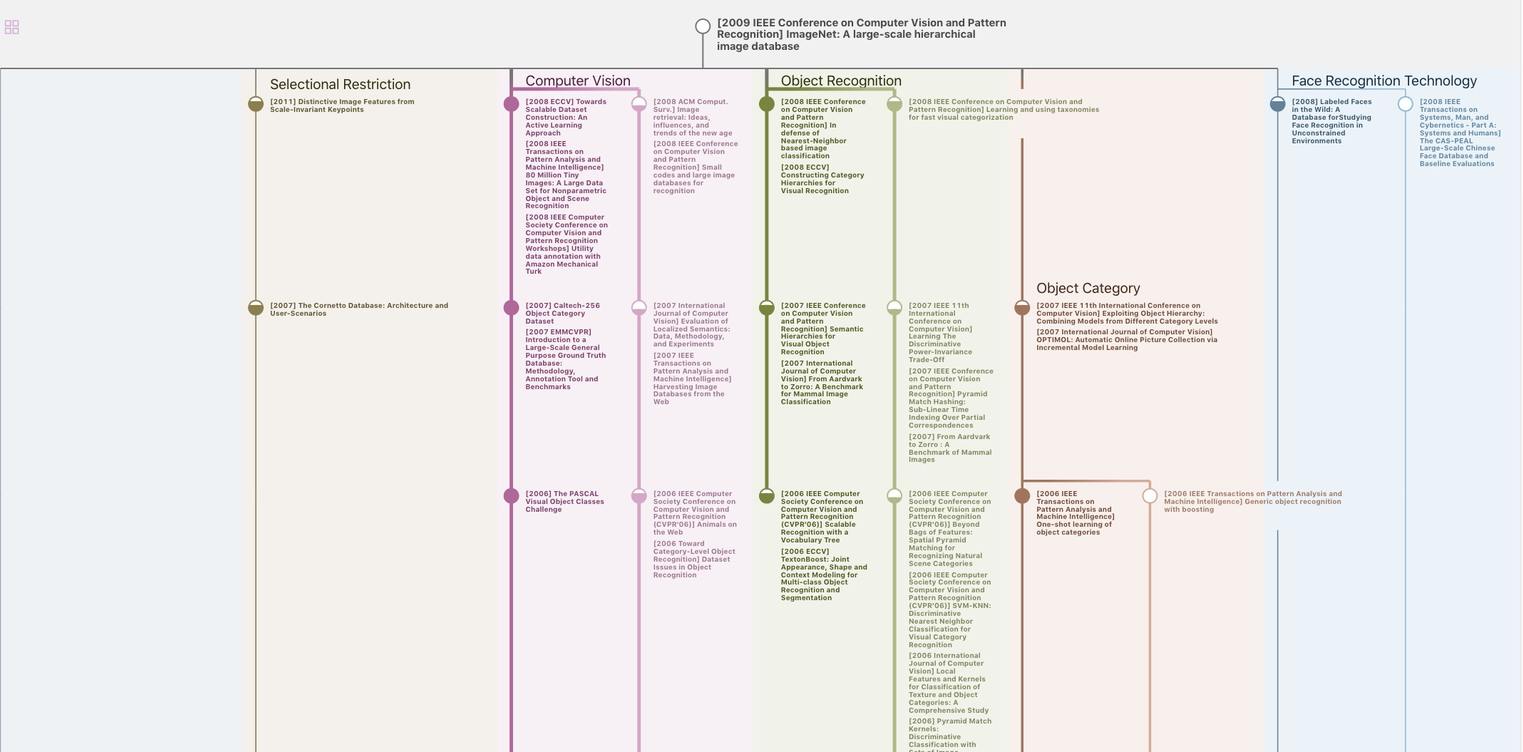
生成溯源树,研究论文发展脉络
Chat Paper
正在生成论文摘要