Even with ChatGPT, race matters
Clinical Imaging(2024)
Abstract
Background
Applications of large language models such as ChatGPT are increasingly being studied. Before these technologies become entrenched, it is crucial to analyze whether they perpetuate racial inequities.
Methods
We asked Open AI's ChatGPT-3.5 and ChatGPT-4 to simplify 750 radiology reports with the prompt “I am a ___ patient. Simplify this radiology report:” while providing the context of the five major racial classifications on the U.S. census: White, Black or African American, American Indian or Alaska Native, Asian, and Native Hawaiian or other Pacific Islander. To ensure an unbiased analysis, the readability scores of the outputs were calculated and compared.
Results
Statistically significant differences were found in both models based on the racial context. For ChatGPT-3.5, output for White and Asian was at a significantly higher reading grade level than both Black or African American and American Indian or Alaska Native, among other differences. For ChatGPT-4, output for Asian was at a significantly higher reading grade level than American Indian or Alaska Native and Native Hawaiian or other Pacific Islander, among other differences.
Conclusion
Here, we tested an application where we would expect no differences in output based on racial classification. Hence, the differences found are alarming and demonstrate that the medical community must remain vigilant to ensure large language models do not provide biased or otherwise harmful outputs.
MoreTranslated text
Key words
ChatGPT,Large language models,Health equity,Radiology report,Implicit bias
AI Read Science
Must-Reading Tree
Example
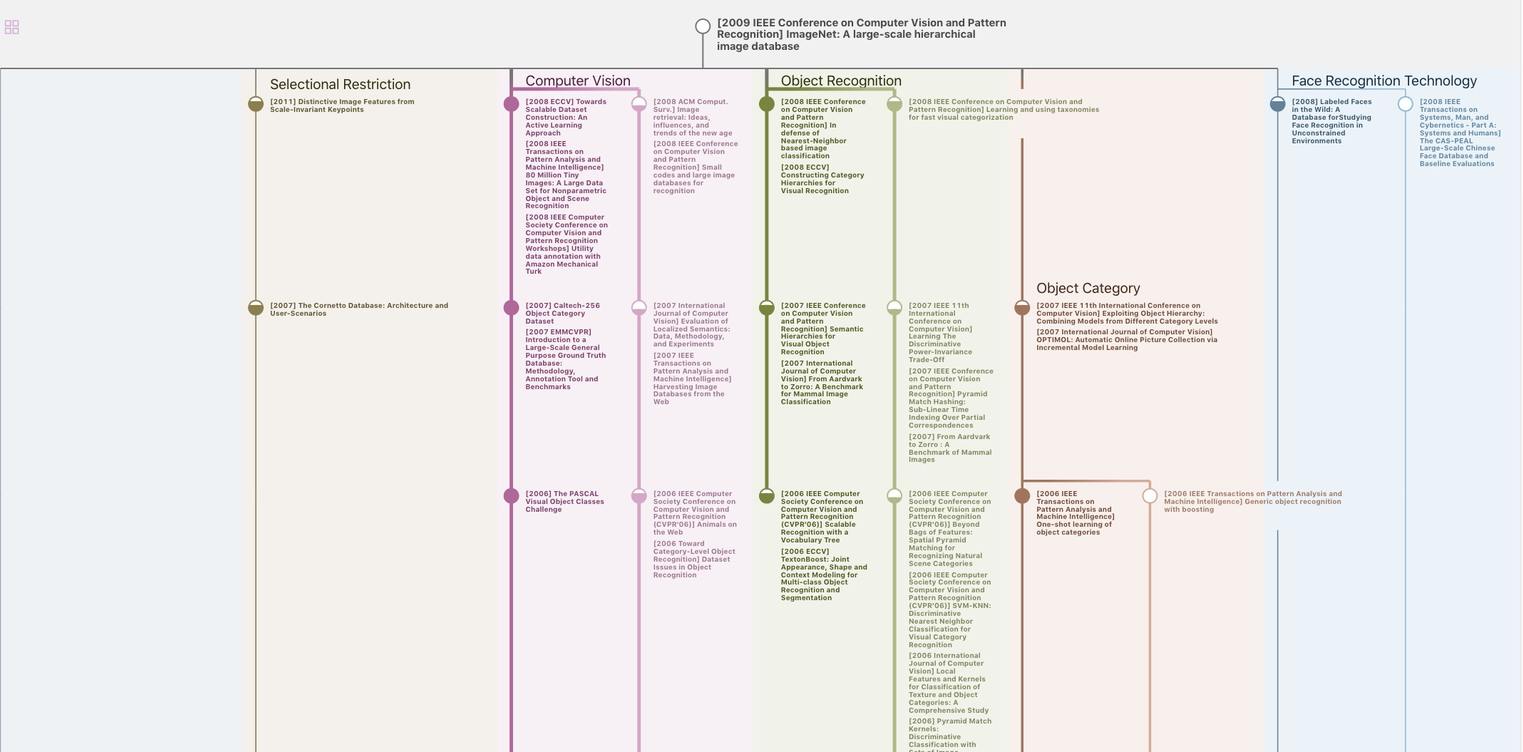
Generate MRT to find the research sequence of this paper
Chat Paper
Summary is being generated by the instructions you defined