A Comparative Study of Rapidly-exploring Random Tree Algorithms Applied to Ship Trajectory Planning and Behavior Generation
arxiv(2024)
摘要
Rapidly Exploring Random Tree (RRT) algorithms are popular for sampling-based
planning for nonholonomic vehicles in unstructured environments. However, we
argue that previous work does not illuminate the challenges when employing such
algorithms. Thus, in this article, we do a first comparison study of the
performance of the following previously proposed RRT algorithm variants;
Potential-Quick RRT* (PQ-RRT*), Informed RRT* (IRRT*), RRT* and RRT, for
single-query nonholonomic motion planning over several cases in the
unstructured maritime environment. The practicalities of employing such
algorithms in the maritime domain are also discussed. On the side, we contend
that these algorithms offer value not only for Collision Avoidance Systems
(CAS) trajectory planning, but also for the verification of CAS through vessel
behavior generation.
Naturally, optimal RRT variants yield more distance-optimal paths at the cost
of increased computational time due to the tree wiring process with nearest
neighbor consideration. PQ-RRT* achieves marginally better results than IRRT*
and RRT*, at the cost of higher tuning complexity and increased wiring time.
Based on the results, we argue that for time-critical applications the
considered RRT algorithms are, as stand-alone planners, more suitable for use
in smaller problems or problems with low obstacle congestion ratio. This is
attributed to the curse of dimensionality, and trade-off with available memory
and computational resources.
更多查看译文
AI 理解论文
溯源树
样例
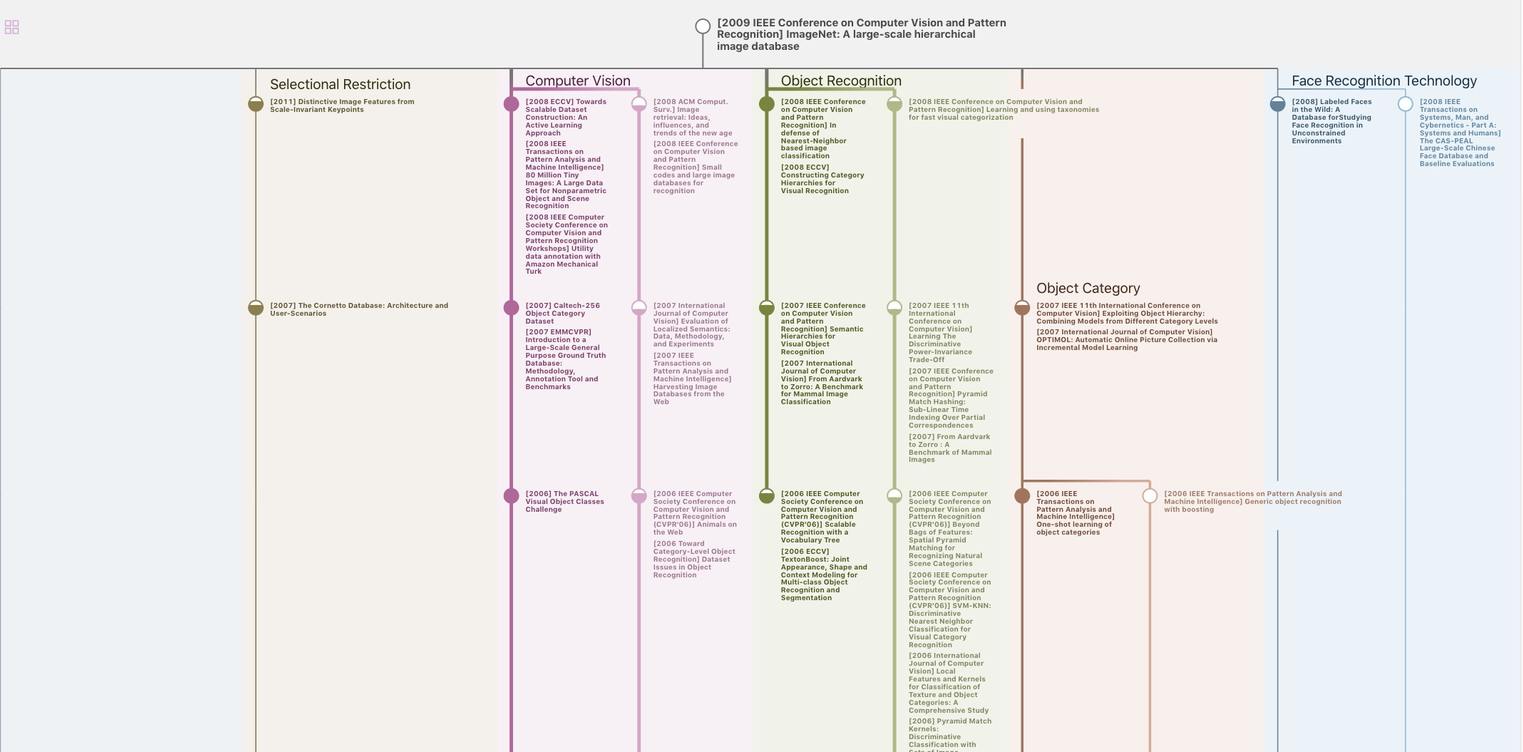
生成溯源树,研究论文发展脉络
Chat Paper
正在生成论文摘要