Accelerating Hydrodynamic Fabrication of Microstructures using Deep Neural Networks
CoRR(2024)
摘要
Manufacturing of microstructures using a microfluidic device is a largely
empirical effort due to the multi-physical nature of the fabrication process.
As such, models are desired that will predict microstructure performance
characteristics (e.g., size, porosity, and stiffness) based on known inputs,
such as sheath and core fluid flow rates. Potentially more useful is the
prospect of inputting desired performance characteristics into a design model
to extract appropriate manufacturing parameters. In this study, we demonstrate
that deep neural networks (DNNs) trained with sparse datasets augmented by
synthetic data can produce accurate predictive and design models. For our
predictive model with known sheath and core flow rates and bath solution
percentage, calculated solid microfiber dimensions are shown to be greater than
95
accuracy for a majority of conditions. Likewise, the design model is able to
recover sheath and core flow rates with 95
microfiber dimensions, porosity, and Young's modulus. As a result, DNN-based
modeling of the microfiber fabrication process demonstrates high potential for
reducing time to manufacture of microstructures with desired characteristics.
更多查看译文
AI 理解论文
溯源树
样例
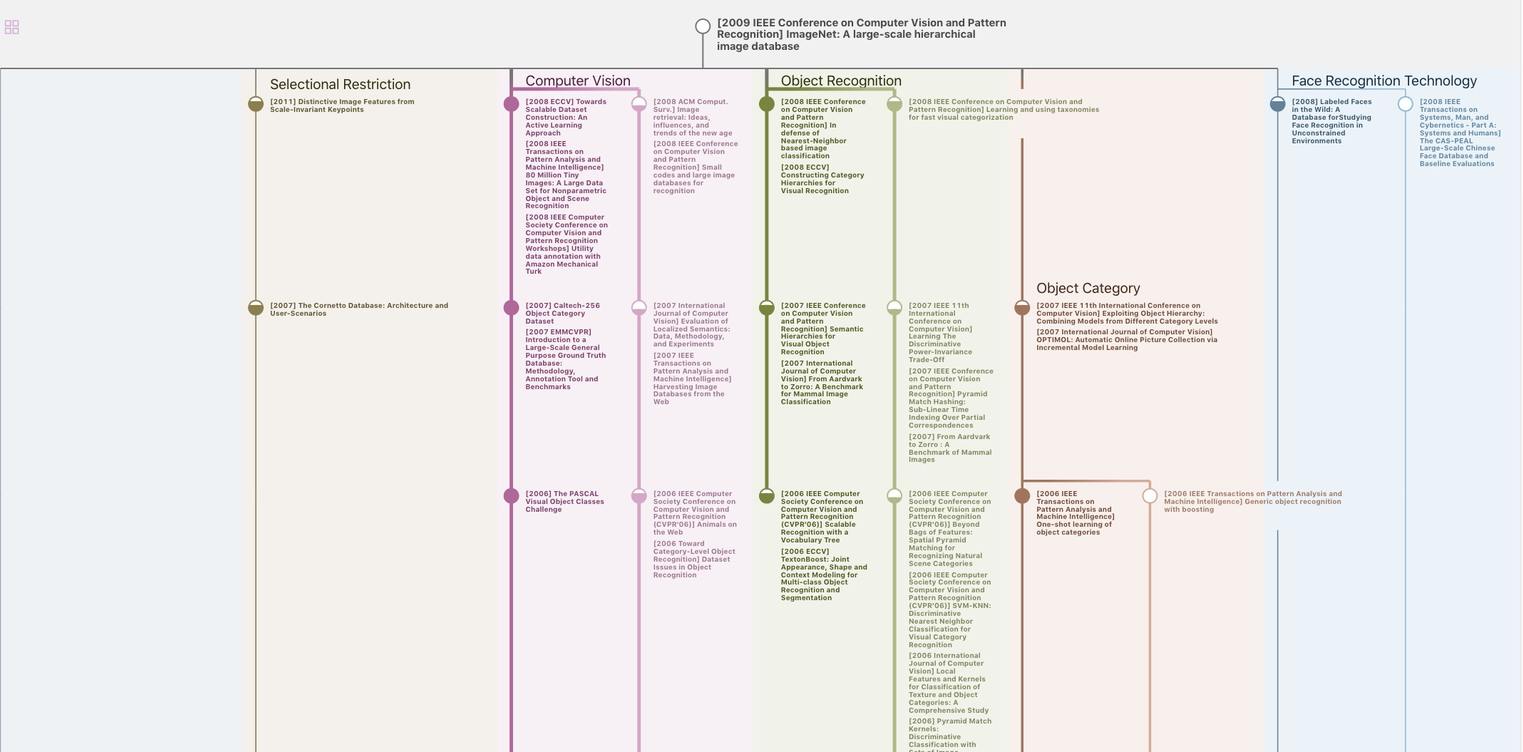
生成溯源树,研究论文发展脉络
Chat Paper
正在生成论文摘要