Γ-VAE: Curvature regularized variational autoencoders for uncovering emergent low dimensional geometric structure in high dimensional data
CoRR(2024)
Abstract
Natural systems with emergent behaviors often organize along low-dimensional
subsets of high-dimensional spaces. For example, despite the tens of thousands
of genes in the human genome, the principled study of genomics is fruitful
because biological processes rely on coordinated organization that results in
lower dimensional phenotypes. To uncover this organization, many nonlinear
dimensionality reduction techniques have successfully embedded high-dimensional
data into low-dimensional spaces by preserving local similarities between data
points. However, the nonlinearities in these methods allow for too much
curvature to preserve general trends across multiple non-neighboring data
clusters, thereby limiting their interpretability and generalizability to
out-of-distribution data. Here, we address both of these limitations by
regularizing the curvature of manifolds generated by variational autoencoders,
a process we coin “Γ-VAE”. We demonstrate its utility using two
example data sets: bulk RNA-seq from the The Cancer Genome Atlas (TCGA) and the
Genotype Tissue Expression (GTEx); and single cell RNA-seq from a lineage
tracing experiment in hematopoietic stem cell differentiation. We find that the
resulting regularized manifolds identify mesoscale structure associated with
different cancer cell types, and accurately re-embed tissues from completely
unseen, out-of distribution cancers as if they were originally trained on them.
Finally, we show that preserving long-range relationships to differentiated
cells separates undifferentiated cells – which have not yet specialized –
according to their eventual fate. Broadly, we anticipate that regularizing the
curvature of generative models will enable more consistent, predictive, and
generalizable models in any high-dimensional system with emergent
low-dimensional behavior.
MoreTranslated text
AI Read Science
Must-Reading Tree
Example
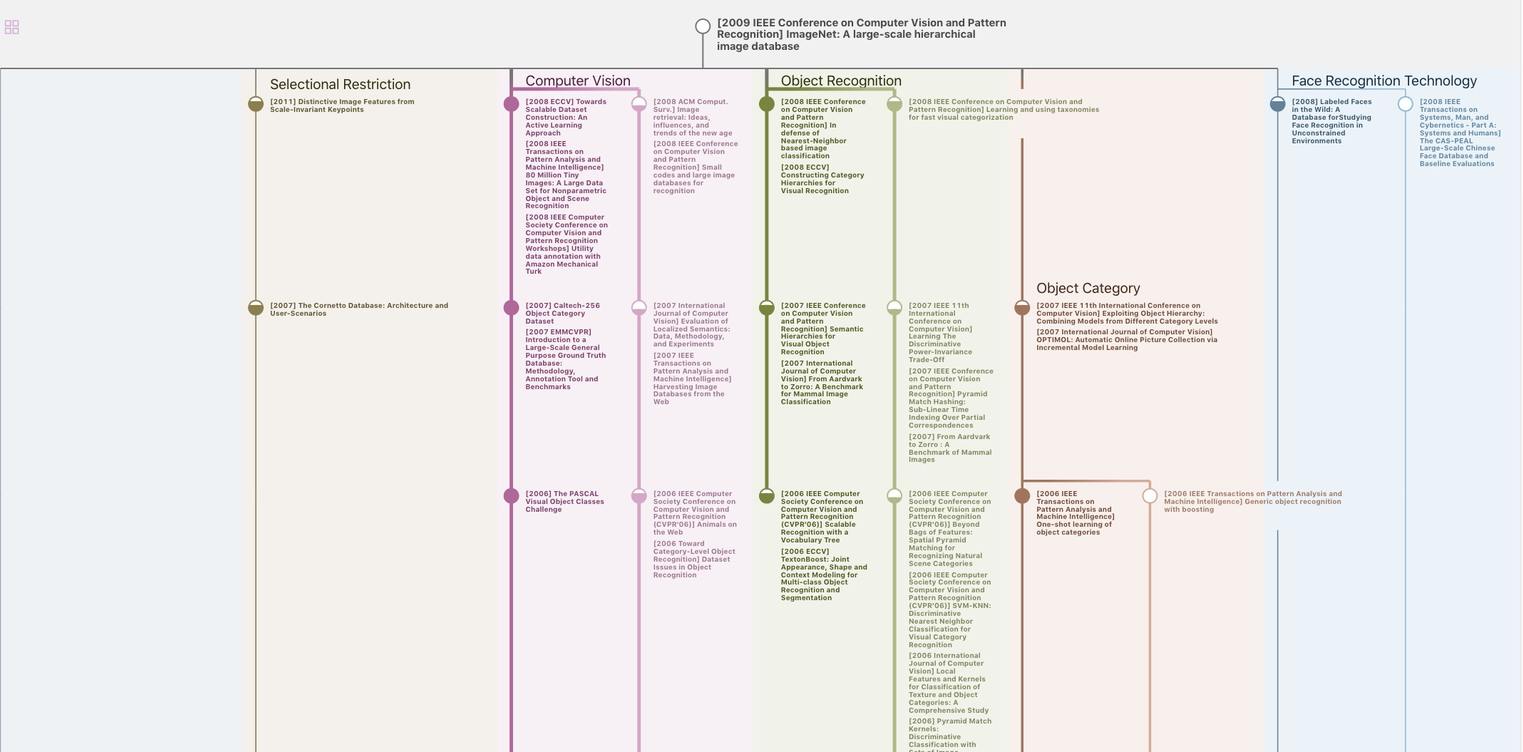
Generate MRT to find the research sequence of this paper
Chat Paper
Summary is being generated by the instructions you defined