LocalRQA: From Generating Data to Locally Training, Testing, and Deploying Retrieval-Augmented QA Systems
CoRR(2024)
Abstract
Retrieval-augmented question-answering systems combine retrieval techniques
with large language models to provide answers that are more accurate and
informative. Many existing toolkits allow users to quickly build such systems
using off-the-shelf models, but they fall short in supporting researchers and
developers to customize the model training, testing, and deployment process. We
propose LocalRQA, an open-source toolkit that features a wide selection of
model training algorithms, evaluation methods, and deployment tools curated
from the latest research. As a showcase, we build QA systems using online
documentation obtained from Databricks and Faire's websites. We find 7B-models
trained and deployed using LocalRQA reach a similar performance compared to
using OpenAI's text-ada-002 and GPT-4-turbo.
MoreTranslated text
AI Read Science
Must-Reading Tree
Example
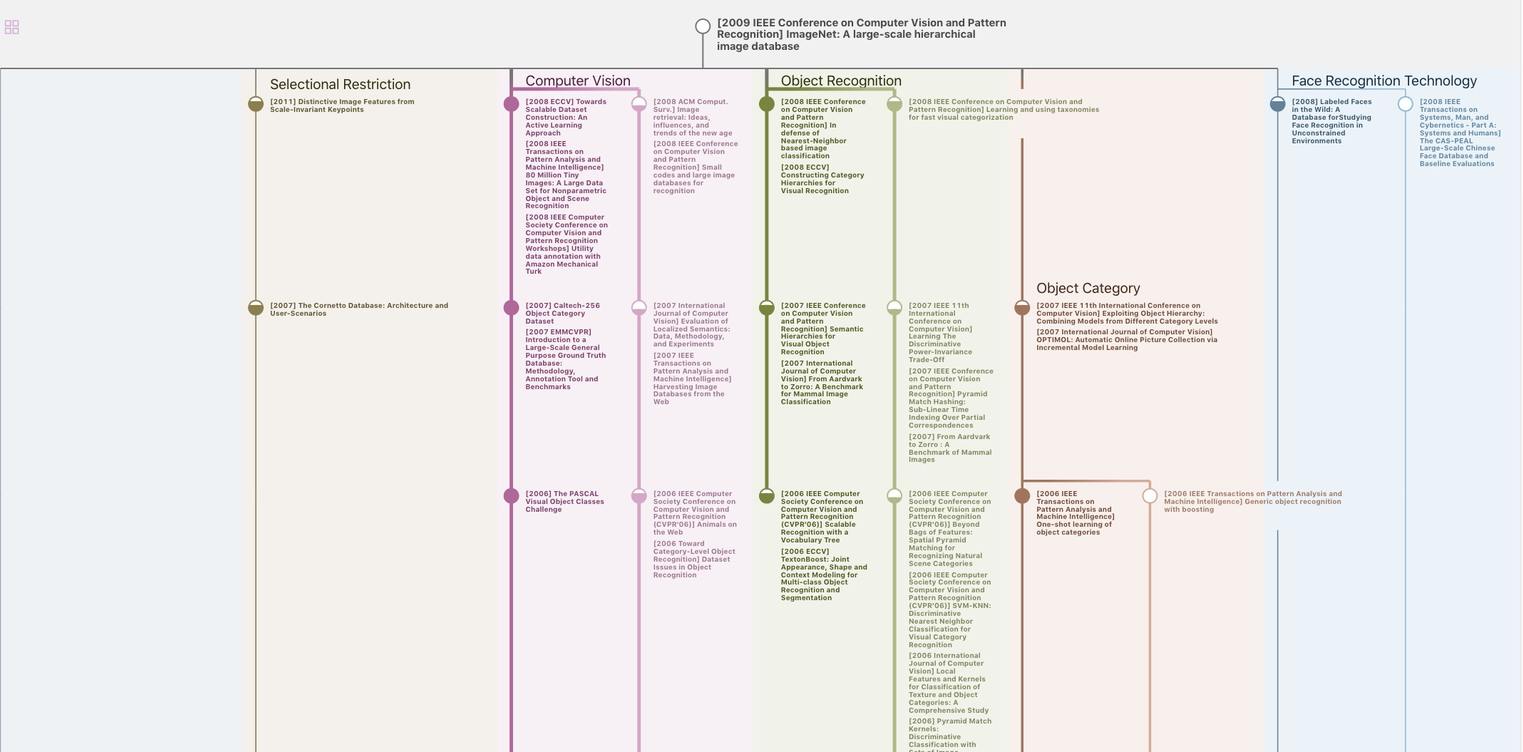
Generate MRT to find the research sequence of this paper
Chat Paper
Summary is being generated by the instructions you defined