Teach LLMs to Phish: Stealing Private Information from Language Models
ICLR 2024(2024)
摘要
When large language models are trained on private data, it can be a
significant privacy risk for them to memorize and regurgitate sensitive
information. In this work, we propose a new practical data extraction attack
that we call "neural phishing". This attack enables an adversary to target and
extract sensitive or personally identifiable information (PII), e.g., credit
card numbers, from a model trained on user data with upwards of 10
success rates, at times, as high as 50
adversary can insert as few as 10s of benign-appearing sentences into the
training dataset using only vague priors on the structure of the user data.
更多查看译文
关键词
LLMs,machine learning,memorization,privacy,data poisoning,federated learning,large language models,privacy risks
AI 理解论文
溯源树
样例
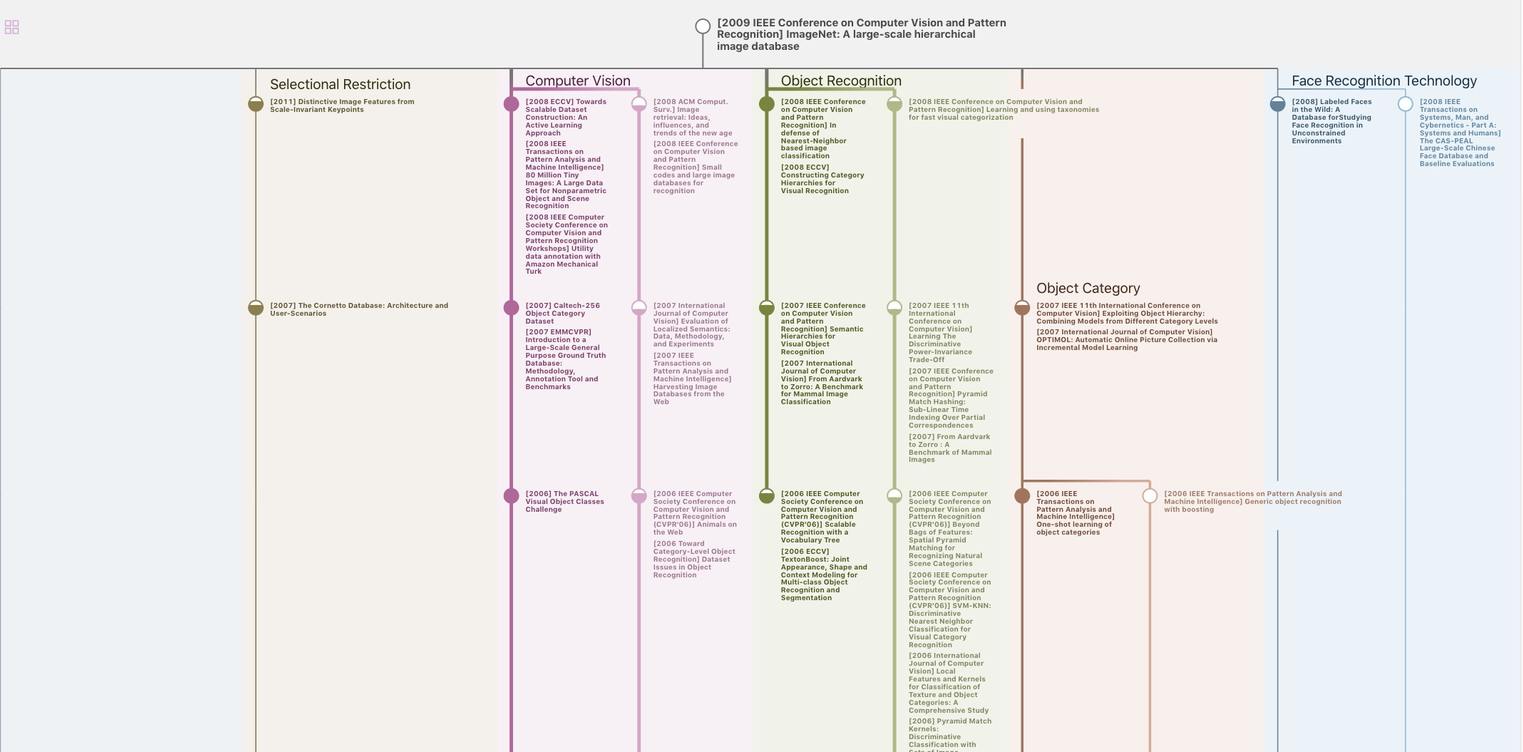
生成溯源树,研究论文发展脉络
Chat Paper
正在生成论文摘要