Leveraging Contrastive Learning for Few-shot Geolocation of Social Posts
CoRR(2024)
摘要
Social geolocation is an important problem of predicting the originating
locations of social media posts. However, this task is challenging due to the
need for a substantial volume of training data, alongside well-annotated
labels. These issues are further exacerbated by new or less popular locations
with insufficient labels, further leading to an imbalanced dataset. In this
paper, we propose ContrastGeo, a Contrastive learning
enhanced framework for few-shot social Geolocation. Specifically, a
Tweet-Location Contrastive learning objective is introduced to align
representations of tweets and locations within tweet-location pairs. To capture
the correlations between tweets and locations, a Tweet-Location Matching
objective is further adopted into the framework and refined via an online hard
negative mining approach. We also develop three fusion strategies with various
fusion encoders to better generate joint representations of tweets and
locations. Comprehensive experiments on three social media datasets highlight
ContrastGeo's superior performance over several state-of-the-art baselines in
few-shot social geolocation.
更多查看译文
AI 理解论文
溯源树
样例
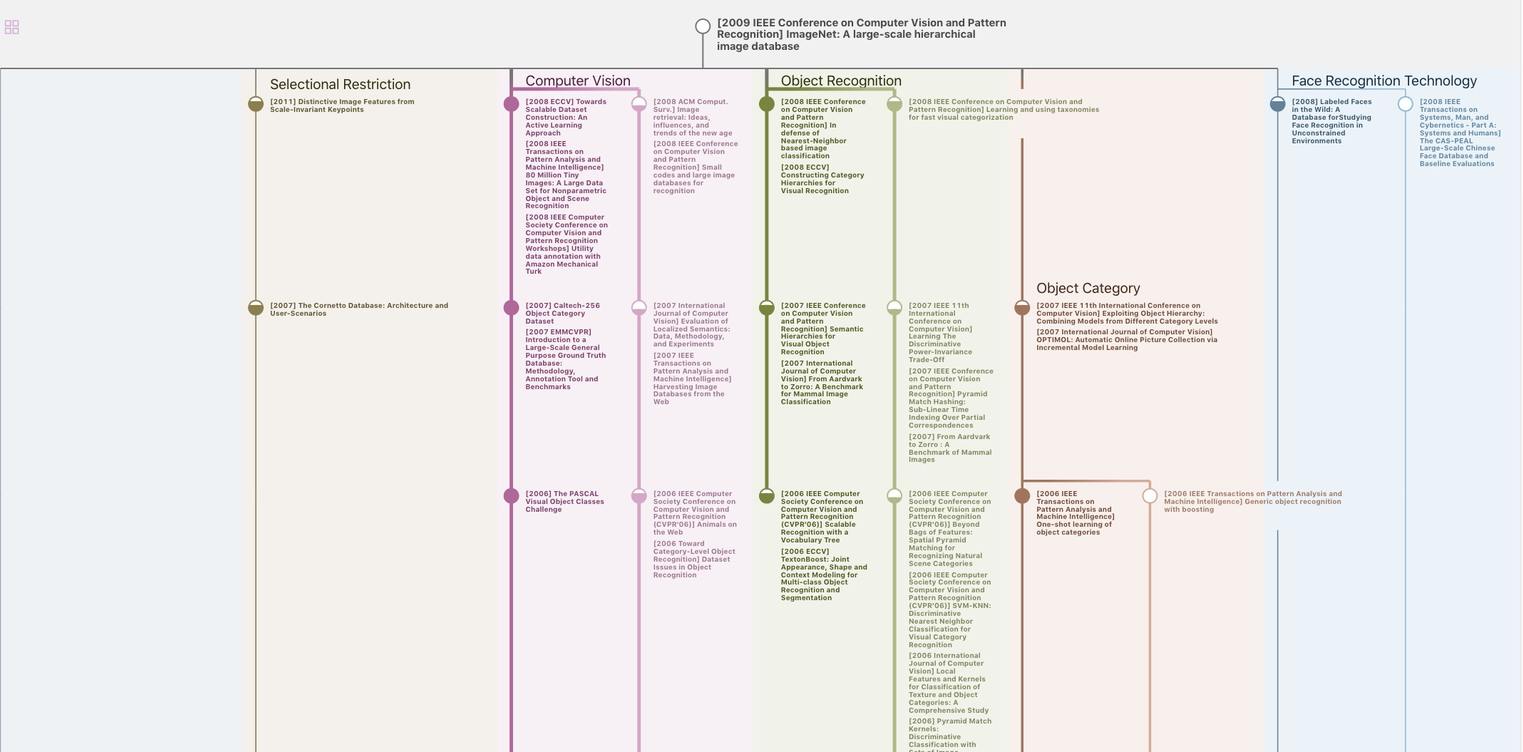
生成溯源树,研究论文发展脉络
Chat Paper
正在生成论文摘要