Models for predicting vehicle emissions: A comprehensive review
SCIENCE OF THE TOTAL ENVIRONMENT(2024)
摘要
Air pollution is a primary concern, causing around 7 million premature deaths annually, with traffic-related sources contributing 23 %-45 % of emissions. While several studies have surveyed vehicle emission models, they are either outdated or focus on specific data-driven models. This paper systematically reviews vehicle emission prediction models, comparing traditional approaches with data-driven emission models. The traditional emission models can be divided into average-speed, modal, and other models, noting their reliance on empirical assumptions and parameters that may not be universally applicable. In contrast, we delve into data-driven models utilizing dynamometer and on-road test data for time-series and spatial-temporal predictions. The application of these models is discussed across various scenarios, highlighting the progress and gap. We observed that traditional models, primarily estimating total traffic emissions in study regions, lack micro-level detail crucial for tailored decisions. The direct link between road emission model accuracy and input data quality poses challenges in disaggregating on-road vehicle emission inventories. Due to unique transportation instruments, traffic fleet components, and patterns, exploring the effects of emission-reduction policies in specific cities or regions is urgent. Vehicle characteristics, environmental conditions, traffic scenarios, and prediction scales are common effect factors, while instantaneous driving profiles prove effective in model calibration. In data -driven models, ANN outperforms in estimating emissions and performance of low -power diesel engines with errors not exceeding 5 %. However, no single data -driven method performed excellently in predicting all pollutants. Be- sides, integrated methods utilizing LSTM, GRU, and RNN outperform individual models. To enhance prediction accuracy considering the inherent connectivity of road networks and spatiotemporal variation patterns of vehicle emissions, GCN is an emerging approach for capturing spatial -temporal relationships based on remote sensing data. Moreover, limited data -driven studies have been performed to forecast particle matter emissions, the main contributors to urban pollution, calling for more attention for future research.
更多查看译文
关键词
Vehicle emission,Data -driven,Time -series,Spatial -temporal,Survey
AI 理解论文
溯源树
样例
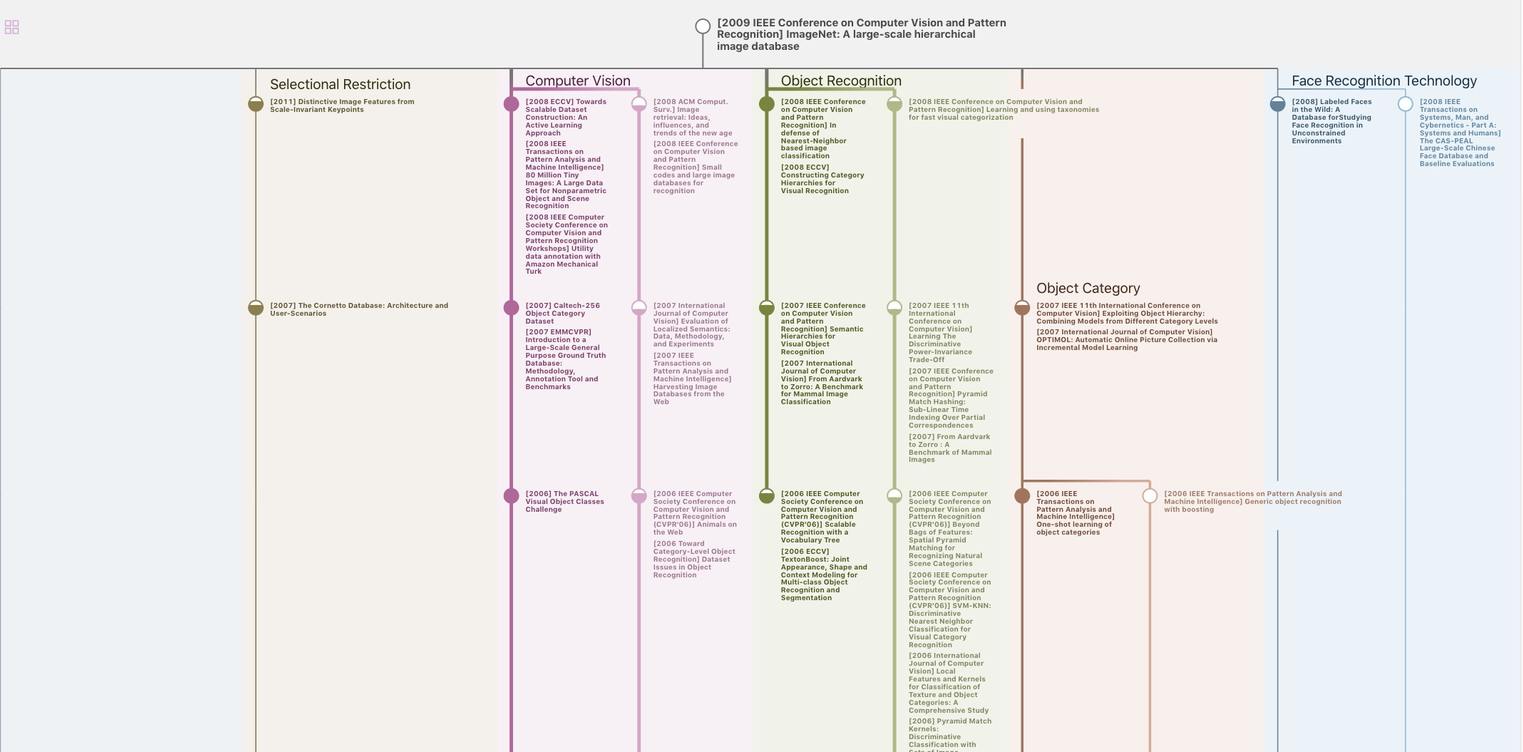
生成溯源树,研究论文发展脉络
Chat Paper
正在生成论文摘要