Flattening Singular Values of Factorized Convolution for Medical Images
ICASSP 2024 - 2024 IEEE International Conference on Acoustics, Speech and Signal Processing (ICASSP)(2024)
摘要
Convolutional neural networks (CNNs) have long been the paradigm of choice
for robust medical image processing (MIP). Therefore, it is crucial to
effectively and efficiently deploy CNNs on devices with different computing
capabilities to support computer-aided diagnosis. Many methods employ
factorized convolutional layers to alleviate the burden of limited
computational resources at the expense of expressiveness. To this end, given
weak medical image-driven CNN model optimization, a Singular value equalization
generalizer-induced Factorized Convolution (SFConv) is proposed to improve the
expressive power of factorized convolutions in MIP models. We first decompose
the weight matrix of convolutional filters into two low-rank matrices to
achieve model reduction. Then minimize the KL divergence between the two
low-rank weight matrices and the uniform distribution, thereby reducing the
number of singular value directions with significant variance. Extensive
experiments on fundus and OCTA datasets demonstrate that our SFConv yields
competitive expressiveness over vanilla convolutions while reducing complexity.
更多查看译文
关键词
Factorized Convolution,KL Divergence,Convolutional Neural Network,Medical Image Processing
AI 理解论文
溯源树
样例
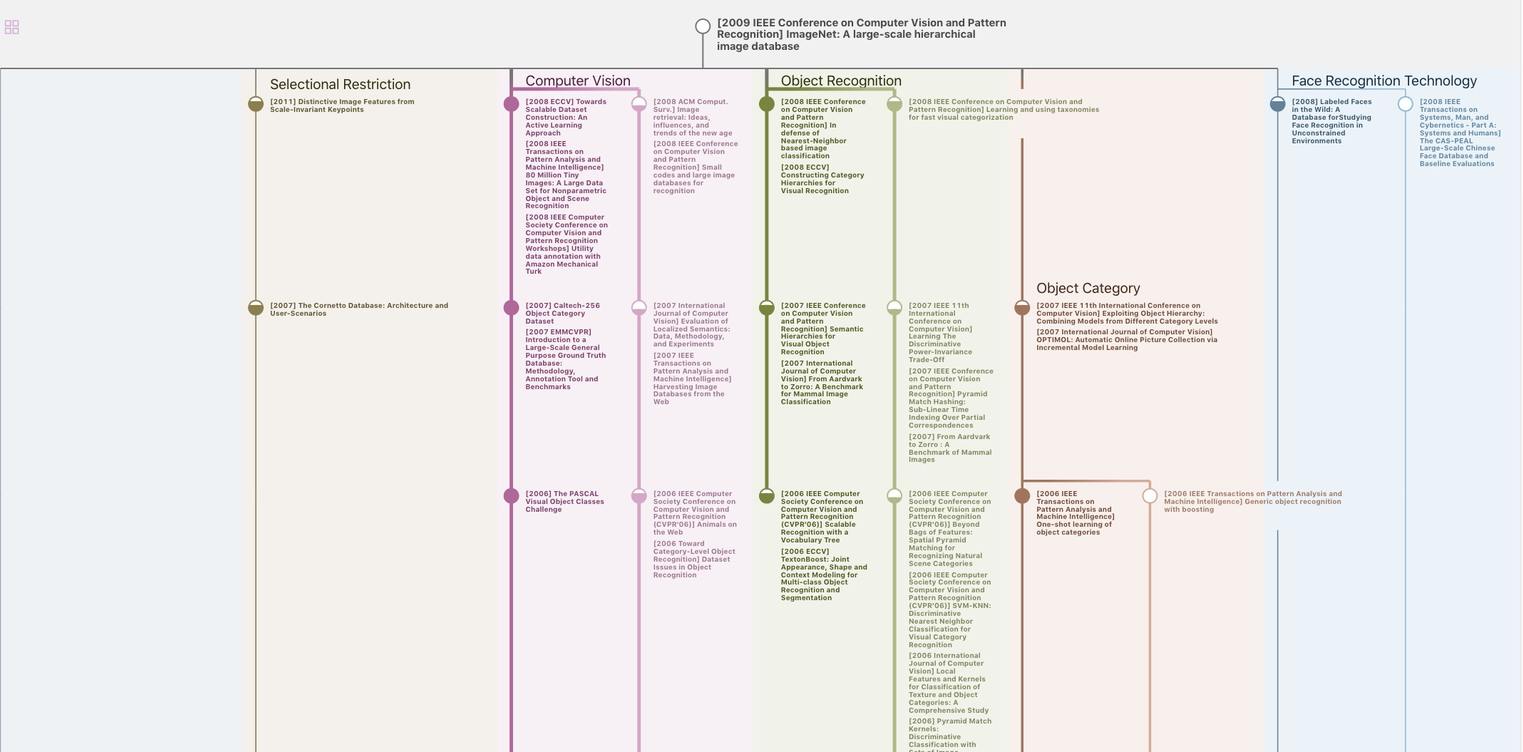
生成溯源树,研究论文发展脉络
Chat Paper
正在生成论文摘要