Validation of ML-UQ calibration statistics using simulated reference values: a sensitivity analysis
CoRR(2024)
摘要
Some popular Machine Learning Uncertainty Quantification (ML-UQ) calibration
statistics do not have predefined reference values and are mostly used in
comparative studies. In consequence, calibration is almost never validated and
the diagnostic is left to the appreciation of the reader. Simulated reference
values, based on synthetic calibrated datasets derived from actual
uncertainties, have been proposed to palliate this problem. As the generative
probability distribution for the simulation of synthetic errors is often not
constrained, the sensitivity of simulated reference values to the choice of
generative distribution might be problematic, shedding a doubt on the
calibration diagnostic. This study explores various facets of this problem, and
shows that some statistics are excessively sensitive to the choice of
generative distribution to be used for validation when the generative
distribution is unknown. This is the case, for instance, of the correlation
coefficient between absolute errors and uncertainties (CC) and of the expected
normalized calibration error (ENCE). A robust validation workflow to deal with
simulated reference values is proposed.
更多查看译文
AI 理解论文
溯源树
样例
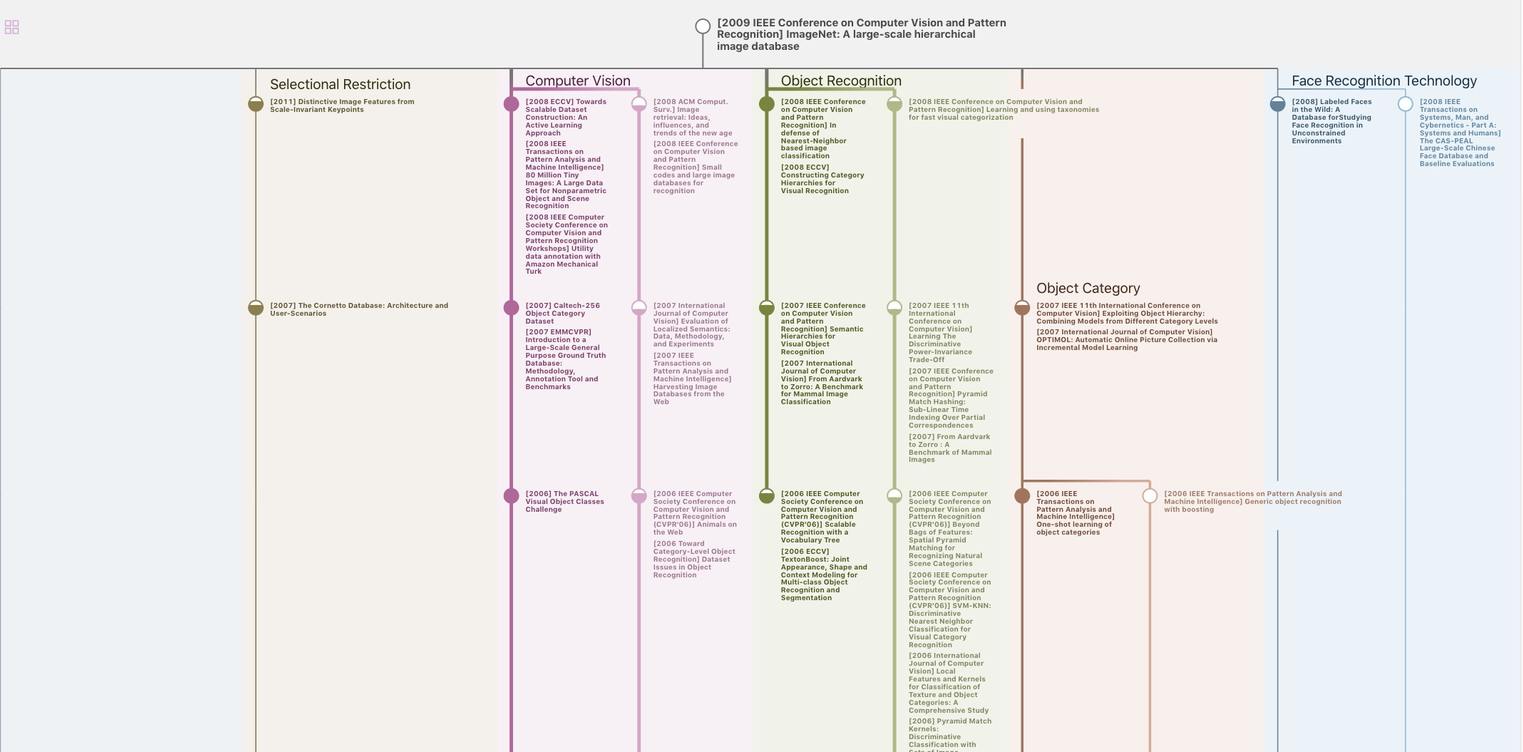
生成溯源树,研究论文发展脉络
Chat Paper
正在生成论文摘要