Task Indicating Transformer for Task-conditional Dense Predictions
ICASSP 2024 - 2024 IEEE International Conference on Acoustics, Speech and Signal Processing (ICASSP)(2024)
摘要
The task-conditional model is a distinctive stream for efficient multi-task
learning. Existing works encounter a critical limitation in learning
task-agnostic and task-specific representations, primarily due to shortcomings
in global context modeling arising from CNN-based architectures, as well as a
deficiency in multi-scale feature interaction within the decoder. In this
paper, we introduce a novel task-conditional framework called Task Indicating
Transformer (TIT) to tackle this challenge. Our approach designs a Mix Task
Adapter module within the transformer block, which incorporates a Task
Indicating Matrix through matrix decomposition, thereby enhancing long-range
dependency modeling and parameter-efficient feature adaptation by capturing
intra- and inter-task features. Moreover, we propose a Task Gate Decoder module
that harnesses a Task Indicating Vector and gating mechanism to facilitate
adaptive multi-scale feature refinement guided by task embeddings. Experiments
on two public multi-task dense prediction benchmarks, NYUD-v2 and
PASCAL-Context, demonstrate that our approach surpasses state-of-the-art
task-conditional methods.
更多查看译文
关键词
Multi-Task Learning,Task-conditional Model,Dense Prediction,Vision Transformer
AI 理解论文
溯源树
样例
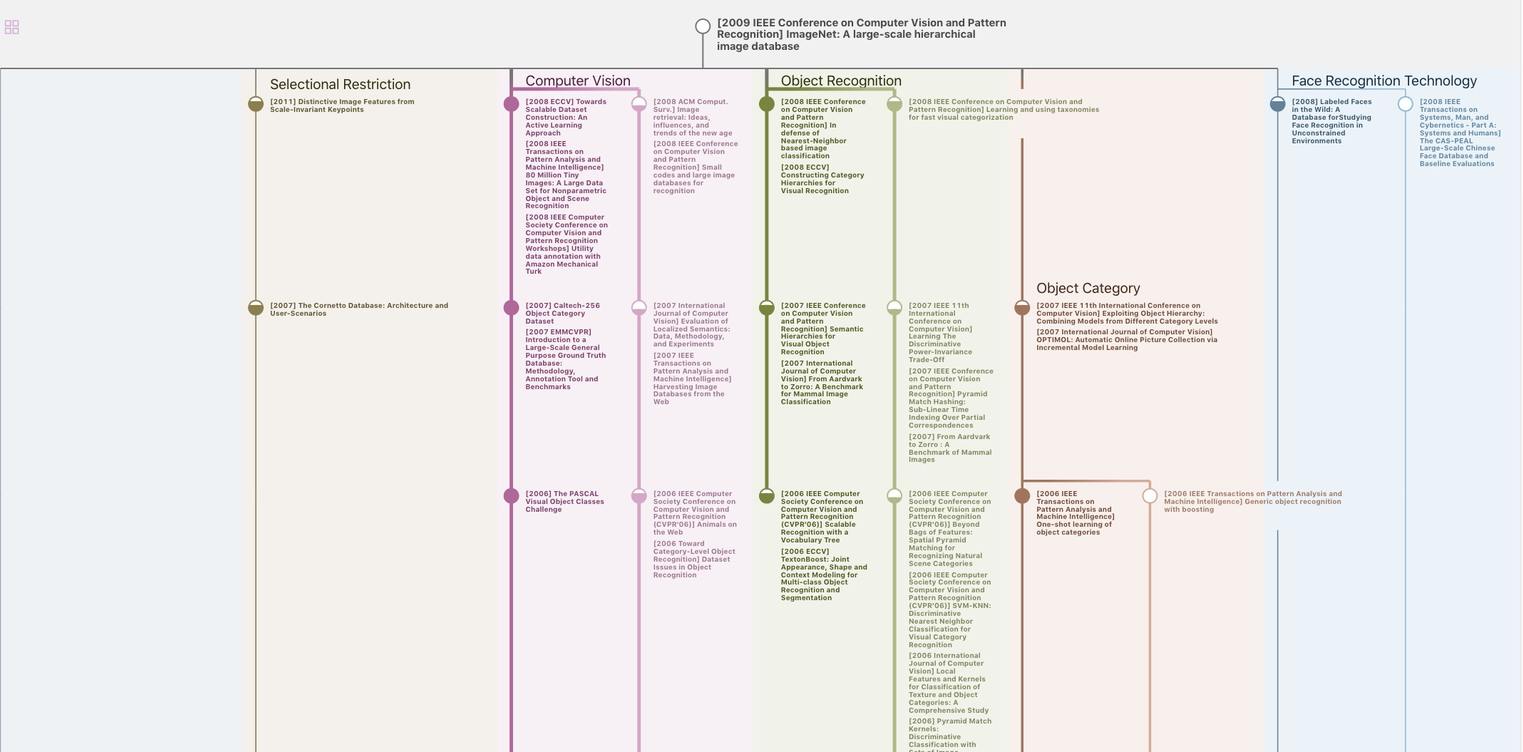
生成溯源树,研究论文发展脉络
Chat Paper
正在生成论文摘要