YOLO-MED : Multi-Task Interaction Network for Biomedical Images
ICASSP 2024 - 2024 IEEE International Conference on Acoustics, Speech and Signal Processing (ICASSP)(2024)
摘要
Object detection and semantic segmentation are pivotal components in
biomedical image analysis. Current single-task networks exhibit promising
outcomes in both detection and segmentation tasks. Multi-task networks have
gained prominence due to their capability to simultaneously tackle segmentation
and detection tasks, while also accelerating the segmentation inference.
Nevertheless, recent multi-task networks confront distinct limitations such as
the difficulty in striking a balance between accuracy and inference speed.
Additionally, they often overlook the integration of cross-scale features,
which is especially important for biomedical image analysis. In this study, we
propose an efficient end-to-end multi-task network capable of concurrently
performing object detection and semantic segmentation called YOLO-Med. Our
model employs a backbone and a neck for multi-scale feature extraction,
complemented by the inclusion of two task-specific decoders. A cross-scale
task-interaction module is employed in order to facilitate information fusion
between various tasks. Our model exhibits promising results in balancing
accuracy and speed when evaluated on the Kvasir-seg dataset and a private
biomedical image dataset.
更多查看译文
关键词
Object Detection,Semantic Segmentation,Multi-Task Learning,Task-interaction,Biomedical Images
AI 理解论文
溯源树
样例
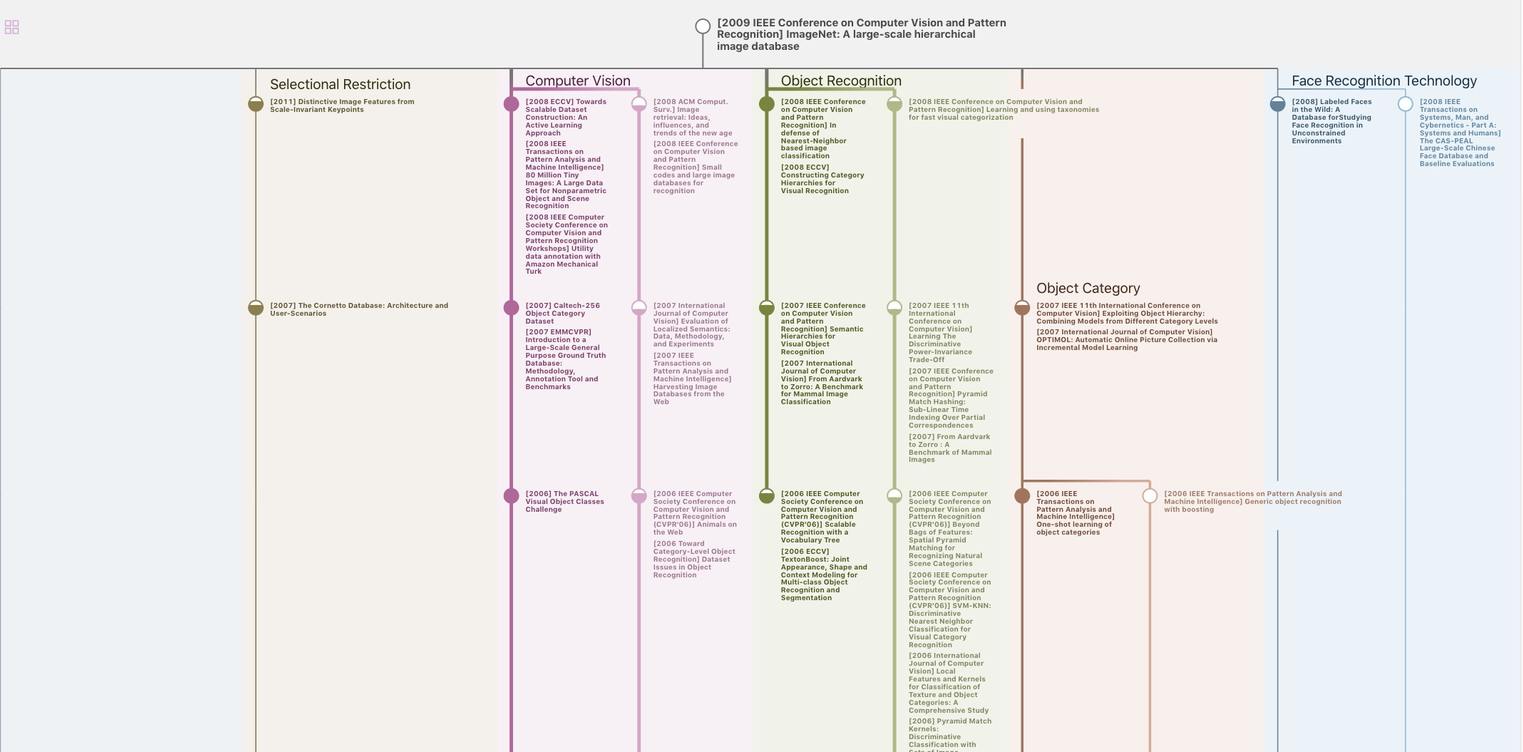
生成溯源树,研究论文发展脉络
Chat Paper
正在生成论文摘要