Lightweight network with masks for light field image super-resolution based on swin attention
Multimedia Tools and Applications(2024)
摘要
Light field (LF) image super-resolution (SR) is a technique designed to enhance the details and clarity of low-resolution (LR) light field images by leveraging the additional information and structure present within the LF data. With the rise of deep learning, the performance of LF image super-resolution has been significantly improved, but it has led to an increase in model parameters and computational complexity, resulting in a phenomenon of excessive reliance on computational resources. To address this problem, this paper proposes a lightweight but effective model. In our approach, we employ Swin Attention to extract features from LF images. Window and Shifted Window are the main components of Swin Attention. Therefore, the local features of the LF images are captured using Window, and feature correlations are established through Shifted Window. Furthermore, we introduce Extensive Attention (EA) blocks to capture the global features of the LF image. In addition to the aforementioned configurations, we have also engineered an iteration of the Low Computation Convolution (LCC) that is capable of eliminating redundant information prior to the feature extraction process in LF images. This design aims to mitigate superfluous computations, thereby enhancing computational efficiency. Experimental results show that our approach achieves suboptimal performance compared to other state-of-the-art models, while having fewer parameters, lower computational complexity, and faster inference speed.
更多查看译文
关键词
Light-field,Spatial super resolution,Lightweight,Swin attention
AI 理解论文
溯源树
样例
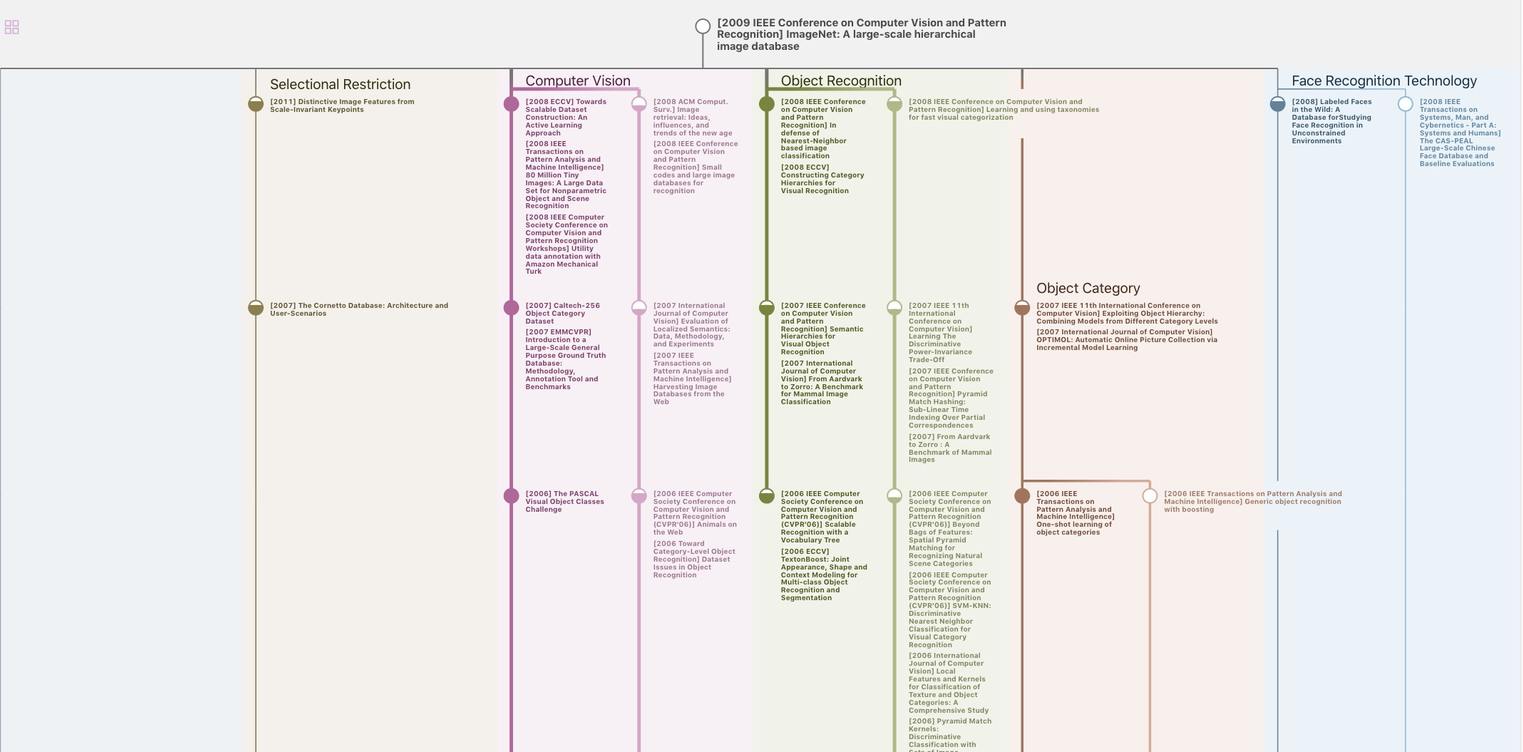
生成溯源树,研究论文发展脉络
Chat Paper
正在生成论文摘要