Dynamic Feature Distillation and Pyramid Split Large Kernel Attention Network for Lightweight Image Super-Resolution
MULTIMEDIA TOOLS AND APPLICATIONS(2024)
Key words
Single image super-resolution,Large kernel attention,Pyramid split module,Lightweight network
AI Read Science
Must-Reading Tree
Example
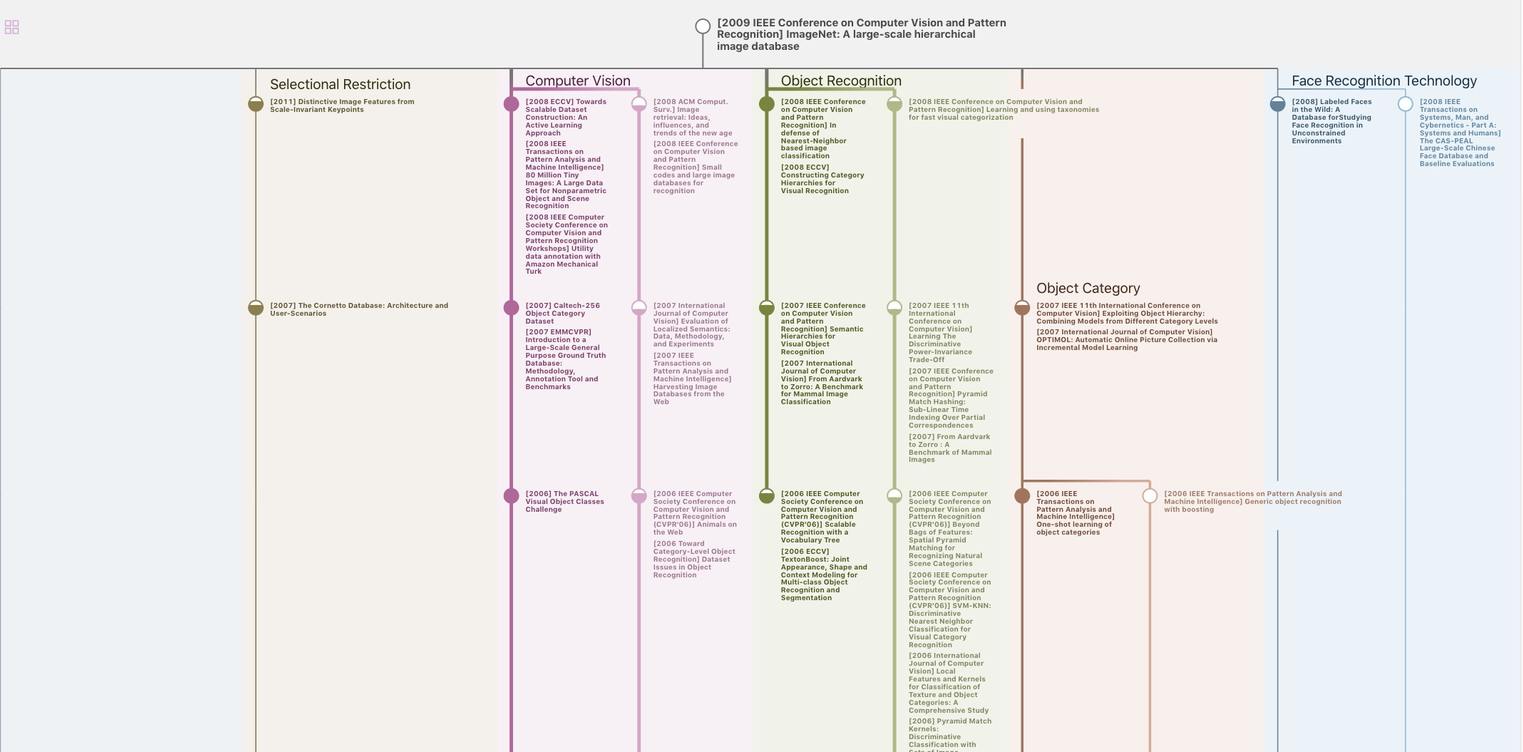
Generate MRT to find the research sequence of this paper
Chat Paper
Summary is being generated by the instructions you defined