Learning to rank influential nodes in complex networks via convolutional neural networks
Applied Intelligence(2024)
摘要
Identifying influential nodes is crucial for enhancing information diffusion in complex networks. Several approaches have been proposed to find these influential nodes based on the network structure that significantly impacts the node influence. Recently, several deep learning algorithms have also been introduced to identify influential nodes based on network exploration and node feature selection. However, this has led to challenges in enhancing efficiency and minimizing computation time. To address these challenges, we propose a novel framework called LCNN that uses convolutional neural networks and node-local representations to identify influential nodes in complex networks. We argue that we can measure node influence capacity using multi-scale metrics and a node’s adjacent matrix of one-hop neighbors to improve extracted information while reducing running time. According to the susceptible-infectious-recovered (SIR) model, the experiment results demonstrate that our proposed LCNN outperforms the state-of-the-art methods on both real-world and synthetic networks. Additionally, it exhibits a moderate time consumption, which makes it suitable for large-scale networks.
更多查看译文
关键词
Influential nodes,Complex network,Information diffusion,Convolutional neural networks
AI 理解论文
溯源树
样例
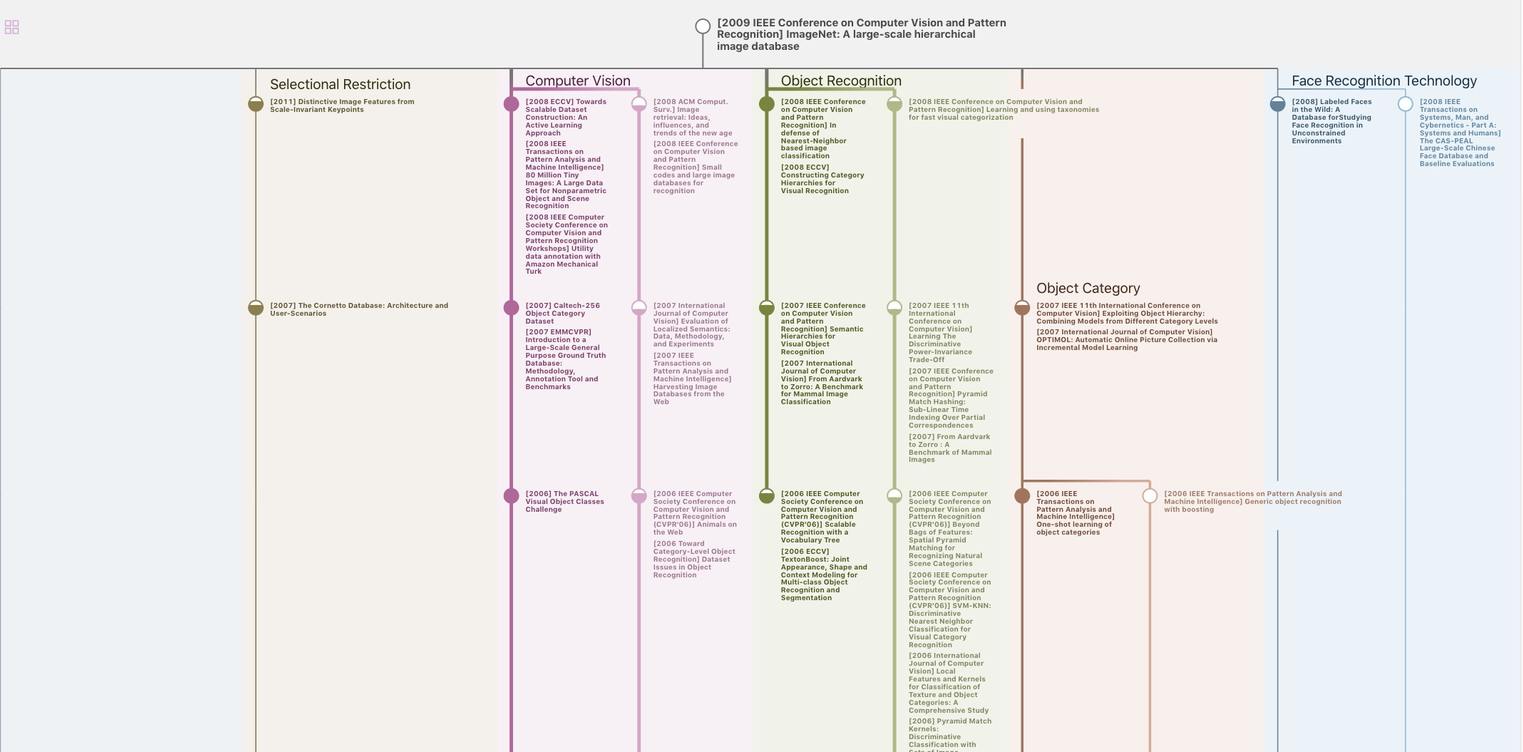
生成溯源树,研究论文发展脉络
Chat Paper
正在生成论文摘要