A Neural Architecture Search Method with Multi-Dimensional Correlation Representation for Fault Diagnosis
2023 IEEE Smart World Congress (SWC)(2023)
摘要
As deep learning has become more widely used for fault diagnosis, the shortcomings of model transferability and human model design costs are growing increasingly evident. The current work has tackled each of these two issues. Domain adaptation methods can provide transportability by mapping data to a common domain. For the consumption of manually constructed networks, Neural Architecture Search (NAS) is an intelligent solution that automatically select the ideal network structure. Unfortunately, there is little work to connect these two methodologies in fault diagnosis. Furthermore, these approaches are incapable in extracting internal correlations from instrumental signal data. In this paper, we propose a transferrable NAS fault diagnosis method, which maximizes savings in manual costs. We also integrate graph neural networks (GNN) in a stepwise manner into the NAS and domain adaptation to provide a multi-dimension correlation representation. Firstly, we structure the signal data into graph based on temporal relationships. Then, NAS designs automatically for selecting the optimal network structure and useful features. In NAS, graph encoder of GNN is used to establish internal relationships. Finally, domain adaptation provides transferability. At the same time, GNN’s weights in domain adaptation help to further mine data internal associations. We tested our method on two publicly available datasets and performed comparisons. The experimental results show the proposed method has superior performance.
更多查看译文
关键词
Fault Diagnosis,Neural Architecture Search,Domain Adaptation,Graph Neural Network
AI 理解论文
溯源树
样例
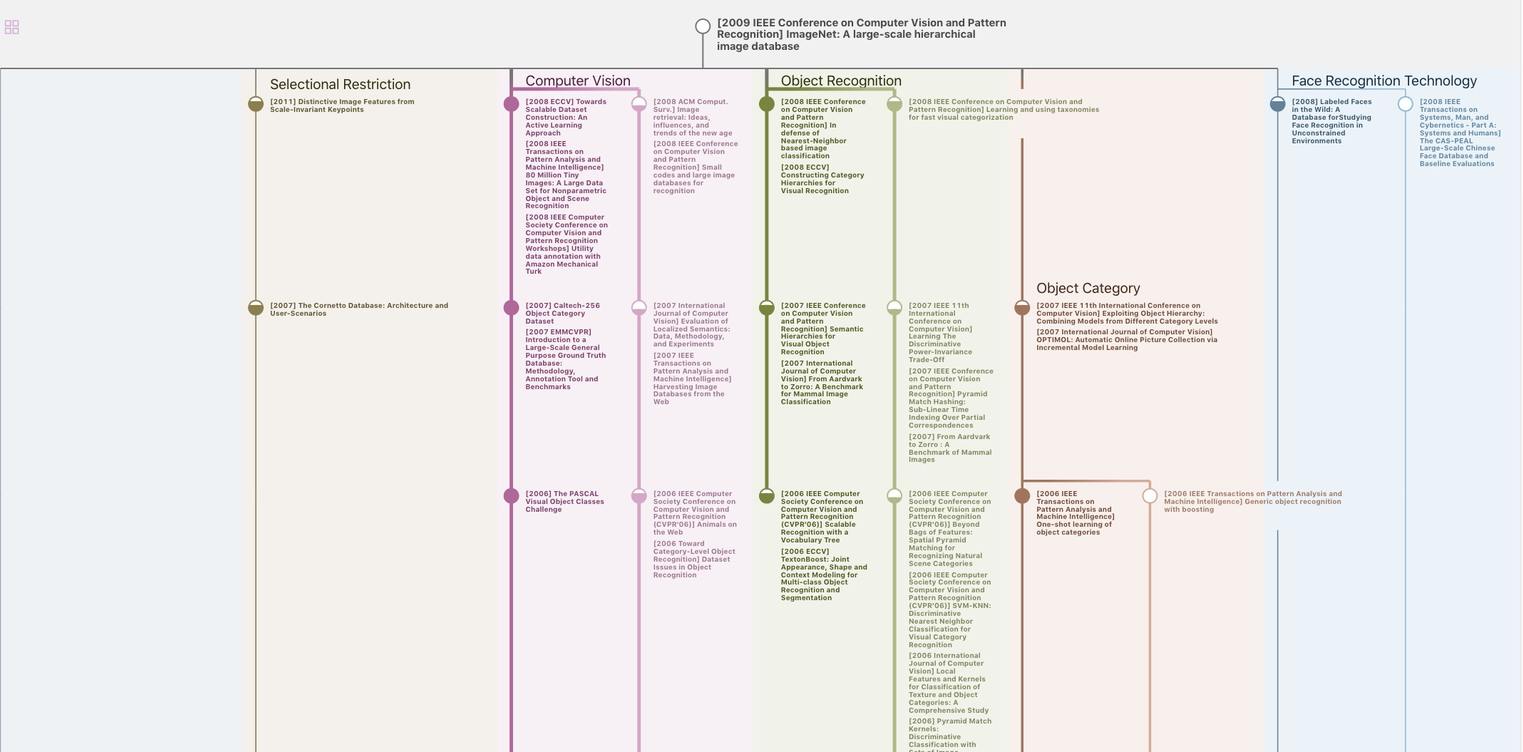
生成溯源树,研究论文发展脉络
Chat Paper
正在生成论文摘要