Lifespan Face Age Progression using 3D-Aware Generative Adversarial Networks
2023 IEEE INTERNATIONAL JOINT CONFERENCE ON BIOMETRICS, IJCB(2023)
摘要
Today, face recognition systems (FRS) are widely used in applications such as forensic and border control systems. Despite the increasing ability of deep neural networks to identify individuals based on their facial images, it remains challenging to recognize faces with long age gaps between reference and probe samples. To improve the robustness of FRS towards aging, training datasets can be enriched with synthetic data by simulating recurring aging effects. Typical aging signs include craniofacial changes during the child-to-adult age transition or textural changes that occur during adult-to-adult aging (e.g., wrinkles or furrows). Building upon the recent achievements of 3D-aware generative adversarial networks, we propose a geometry-aware face age modification algorithm (Age-EG3D) that enables lifespan face age simulation. We demonstrate the effectiveness of our approach by providing a comprehensive performance evaluation and comparison of Age-EG3D to prior works. All code and pre-trained models are available at https://github.com/johndoe133/eg3d-age.
更多查看译文
关键词
Generative Adversarial Networks,Age Progression,Facial Age,Neural Network,Training Dataset,Face Recognition,Face Images,Image Quality,Latent Space,Image Generation,High-quality Images,Age Estimation,Viewing Angle,Latent Representation,Loss Of Identity,Forensic Investigations,Target Age,Explicit Method,Latent Vector,Implicit Method,Fréchet Inception Distance,Identity Preservation,Head Pose,Camera Pose,Facial Appearance,Facial Traits,Spatial Consistency,Variety Of Factors,Inversion Process,3D Representation
AI 理解论文
溯源树
样例
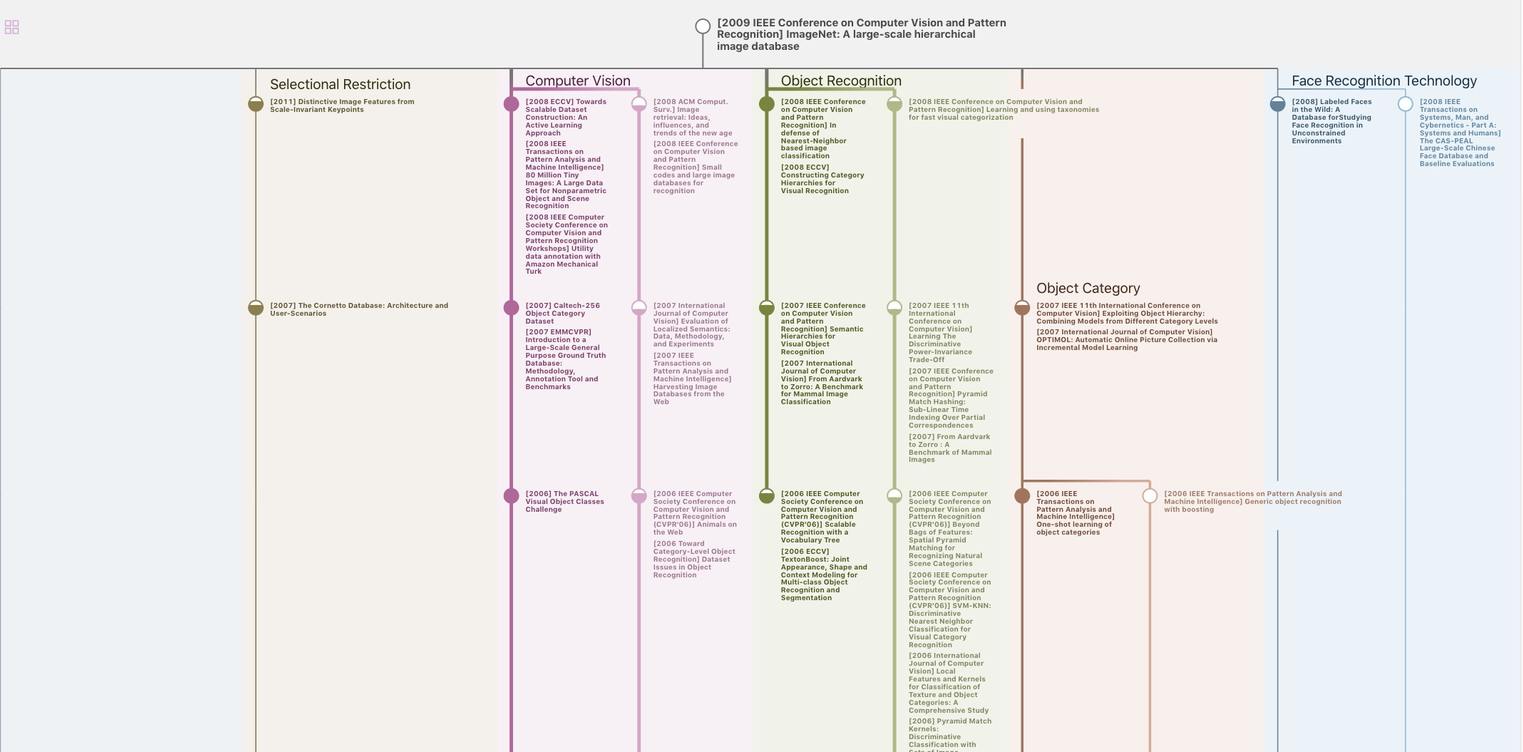
生成溯源树,研究论文发展脉络
Chat Paper
正在生成论文摘要