MAGRO: Inferring Root Causes of Poor Wireless Network Performance Using Knowledge Graph and Heterogeneous Graph Neural Networks
2023 IEEE Smart World Congress (SWC)(2023)
摘要
Cellular networks have experienced swift development to address the high-speed data service demands of rapidly expanding mobile devices. However, as the complexity of the network increases, localizing network problems becomes increasingly intricate, and depending solely on on-site analysis by network optimization experts and engineers can no longer ensure the accuracy and timeliness of existing Root Cause Analysis (RCA). In response to this challenge, data-driven RCA solutions, such as rule-based methods and probabilistic graphical models, have been proposed to improve the efficiency of problem resolution. Despite their advantages, these methods are inadequate in effectively identifying graph data, such as the connection relationships between base stations, which is crucial for resolving optimization problems involving multiple base stations. Thus, this study introduces a novel graph-based approach, MAGRO (Metapath Aggregation Graph based Root-cause Operation), for RCA in telecommunications networks, which leverages a Knowledge Graph and Graph Neural Networks (GNNs) to model the heterogeneous relationships among various components of the telecommunications system and identify the root causes of poor wireless network performance. This approach is evaluated against several baseline methods using the real-world dataset, and the results demonstrate that the proposed approach significantly outperforms other approaches.
更多查看译文
关键词
Root Cause Analysis,Knowledge Graph,Graph Neural Networks,Telecommunications Networks
AI 理解论文
溯源树
样例
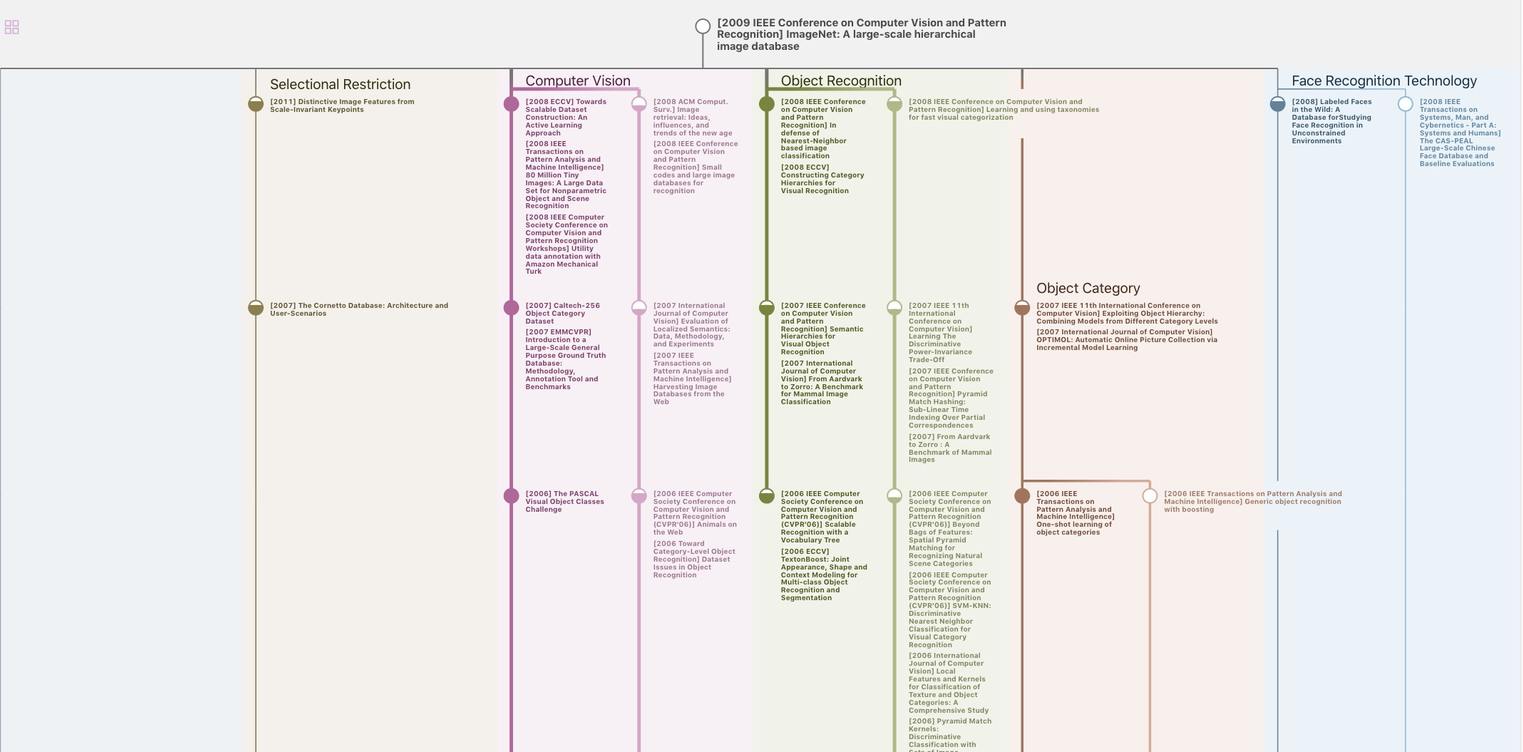
生成溯源树,研究论文发展脉络
Chat Paper
正在生成论文摘要