An advanced deep learning model for predicting water quality index
Ecological Indicators(2024)
摘要
Predicting a water quality index (WQI) is important because it serves as an important metric for assessing the overall health and safety of water bodies. Our paper develops a new hybrid model for predicting the WQI. The study uses a combination of a convolutional neural network (CNN), clockwork recurrent neural network (Clockwork RNN), and M5 Tree (CNN-CRNN-M5T) to predict a WQI. The M5T model lacks advanced operators for extracting meaningful data from water quality parameters, so the new model enhances its ability to analyze intricate patterns. The general linear model analysis of variance (GLM-ANOVA) is an improved version of the ANOVA. Our study uses the GLM-ANOVA to determine significant inputs. As all input variables had p < 0.050, they were defined as significant variables. Results showed that NH-NL and PH had the highest and lowest impact, respectively. Our study used the CNN-CRNN-M5T, CNN-CRNN, CRNN-M5T, CNN-M5T, CRNN, CNN, and M5T models to predict the WQI of a large basin in Malaysia. The CNN-CRNN decreased testing mean absolute error (MAE) of the CRNN, CNN, and M5T models by 2.1 %, 12 %, and 15 %, respectively. The CNN-CRNN-M5T model increased Nash–Sutcliffe efficiency coefficient of the other models by 4–20 % and 2.1–19 %, respectively. The CNN-CRNN-M5T model was a reliable tool for spatial and temporal predictions of WQI.
更多查看译文
关键词
Deep Learning model,Water resource management,Water quality index,Hybrid models
AI 理解论文
溯源树
样例
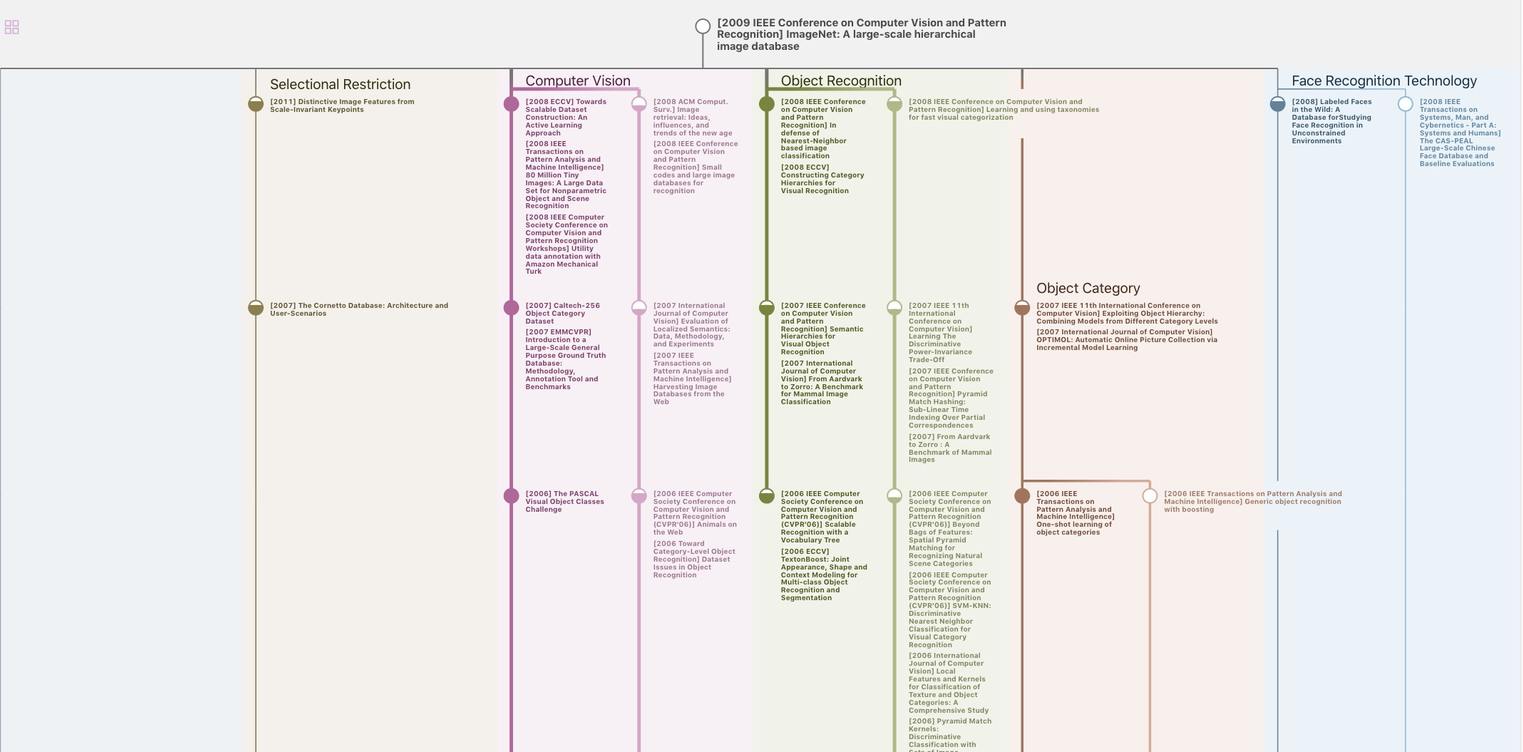
生成溯源树,研究论文发展脉络
Chat Paper
正在生成论文摘要