Dual Branch Deep Network for Ship Classification of Dual-Polarized SAR Images
IEEE TRANSACTIONS ON GEOSCIENCE AND REMOTE SENSING(2024)
摘要
Ship classification is usually a challenging task due to the small sizes of ship targets and the lack of significant differences between different categories. In terms of synthetic aperture radar (SAR) images, most existing deep learning (DL)-based methods are not designed from the angle of polarimetric characteristics to achieve ship classification. Thus, when facing the ship classification task of dual-polarized SAR images, these networks are often unsatisfactory. To cure this shortcoming, we here propose a novel dual branch deep network (DBDN) specifically designed for dual-polarized SAR ship classification. Our approach consists of three key modules: the image construction module (ICM), the feature extraction module (FEM), and the feature fusion and classifier module (FFCM). In ICM, two novel pseudo-RGB images are constructed for the first time, i.e., the polarimetric features-guided pseudo-RGB image (PF-RGB) and the texture features-guided pseudo-RGB image (TF-RGB), which can more accurately and comprehensively reflect ships' characteristics. FEM enables the network to focus on important ship features and suppress irrelevant noise through transferred layers and designed ConvNeXt-Attention block (CNABlock), enhancing the discriminative capability of different ships. Finally, FFCM extracts and combines various ship features for classification, wherein the enhanced inverted residual block (EIRBlock) and the channel spatial attention module (CSAM) components are proposed as well. The performance of DBDN is evaluated on the OpenSARShip2.0 dataset, and experimental results show that DBDN achieves excellent performance in all evaluation metrics in comparison with some state-of-the-art (SOTA) algorithms. For example, compared to the recently proposed method deep SAR-net (DSN), DBDN further improves the accuracy by 4.74% and 4.07% in the three-class and six-class classification tasks, respectively.
更多查看译文
关键词
Dual branch deep network (DBDN),dual-polarized synthetic aperture radar (SAR),feature extraction module (FEM),feature fusion and classifier module (FFCM),image construction module (ICM),ship classification
AI 理解论文
溯源树
样例
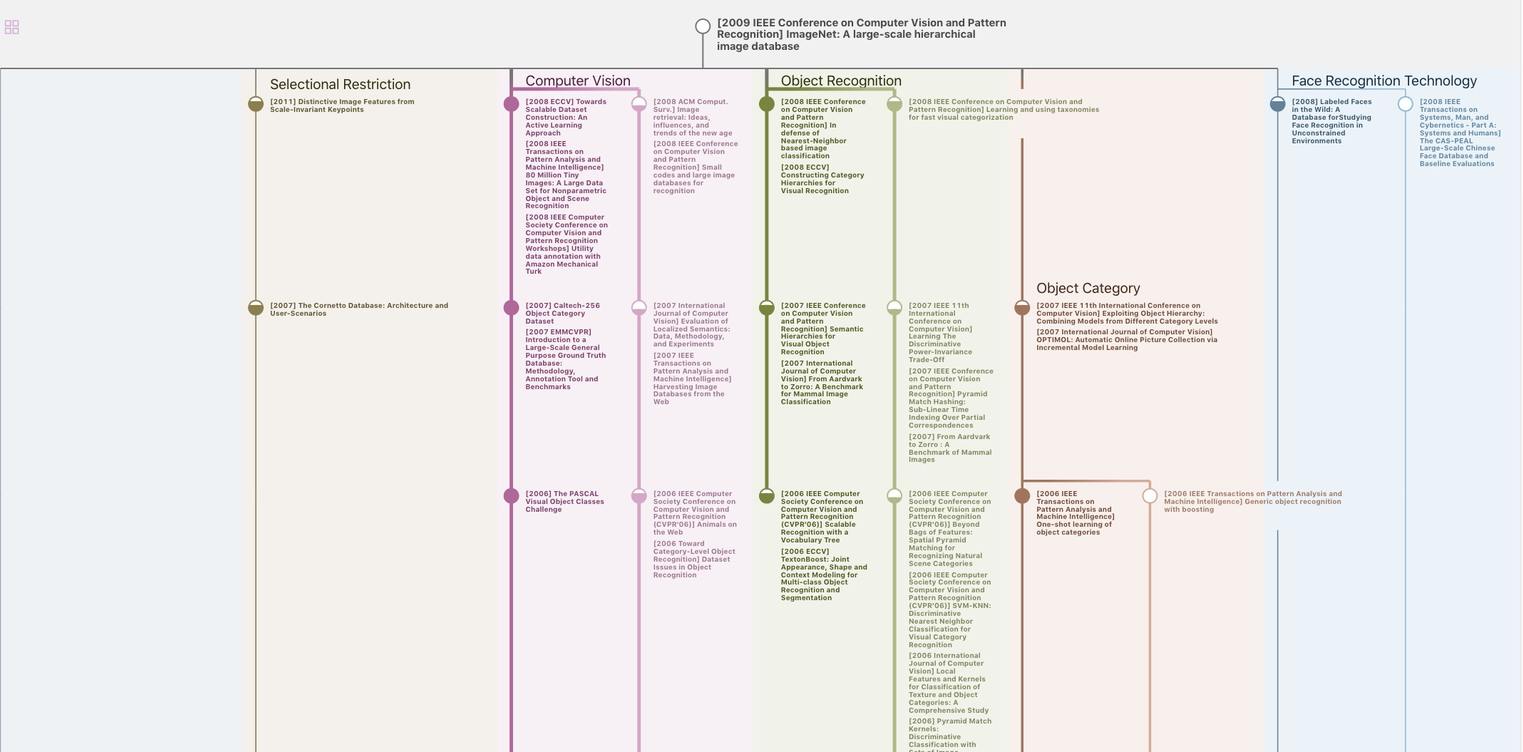
生成溯源树,研究论文发展脉络
Chat Paper
正在生成论文摘要