APIM: An Antiferromagnetic MRAM-Based Processing-In-Memory System for Efficient Bit-level Operations of Quantized Convolutional Neural Networks
IEEE Transactions on Computer-Aided Design of Integrated Circuits and Systems(2024)
Abstract
Quantized Convolutional Neural Network (QCNN) is an attractive approach that reduces hardware overheads, especially for energy-constrained systems. However, existing QCNNs still require non-trivial hardware resources and memory capacity in order not to compromise model accuracy. To address this issue, we propose an antiferromagnetic magnetic random-access memory (ARAM)-based processing-in-memory (PIM) system, leveraging bit-level sparsity. Three optimization techniques are proposed to optimize hardware resource utilization while preserving CNN accuracy. Firstly, the ARAM-based memory subsystem allows dynamic adaptation of variable bit-width across CNN layers. Secondly, the bit-level accelerator employs the bit-fusion format engineered for processing data from the ARAM subsystem. Thirdly, a customized data path within the RISC-V core guarantees efficient instruction processing to the ARAM-based memory subsystem and bit-level accelerator, enabling optimal bit-level data transmission and computation. Experimental results demonstrate that this design remarkably reduces data movement by 50%-83% across existing CNNs. Compared to state-of-the-art designs, it enhances throughput and latency by an average of 5x and 10x, respectively. In addition, this design achieves speedups between 1.63x and 2.96x, outstripping other designs in AlexNet, VGG16, and ResNet18 benchmarks.
MoreTranslated text
Key words
Quantization,Convolutional Neural Network,ARAM,PIM System
AI Read Science
Must-Reading Tree
Example
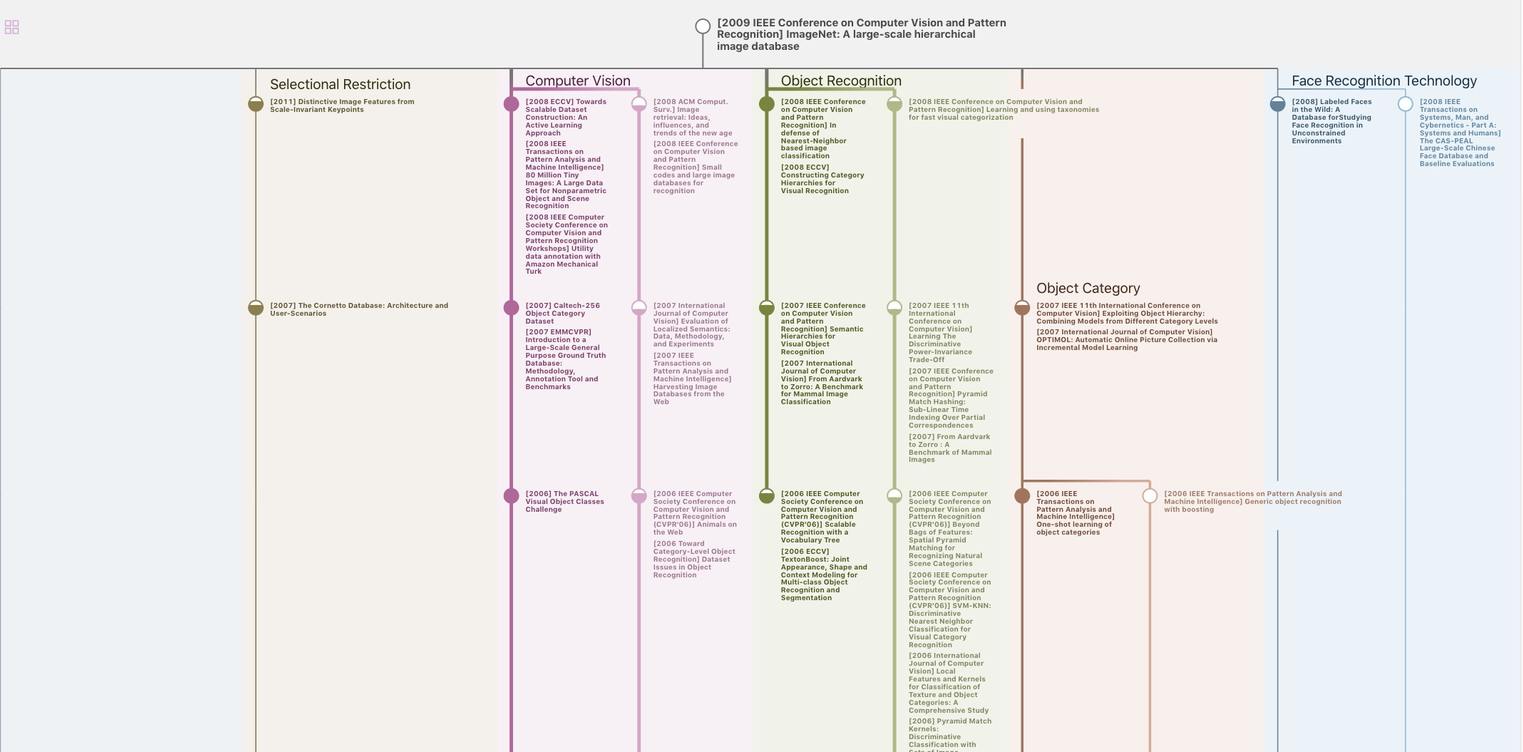
Generate MRT to find the research sequence of this paper
Chat Paper
Summary is being generated by the instructions you defined