Optimization of industrial robot grasping processes with Q-learning
2023 5TH INTERNATIONAL CONFERENCE ON CONTROL AND ROBOTICS, ICCR(2023)
Abstract
Grasping objects in unstructured and dynamic environments remains a challenging task for robotic systems. Traditional grasp planning algorithms often struggle to adapt to the variability and uncertainty present in real-world scenarios. Deep reinforcement learning has emerged as a promising approach for training robotic agents to improve their grasping capabilities. This paper presents a novel grasp refinement strategy that exploits a deep Q-learning algorithm to improve the precision of robotic grasping despite the presence of position and orientation inaccuracies. The proposed strategy focuses on industrial robot grasping processes which are predominantly comprised of a robot with force and torque sensor values and parallel jaw grippers with force and position feedback. Experimental results demonstrate that our RL-based approach increases the grasping accuracy without the presence of complex and high-dimensional tactile sensor input. Moreover, the learned grasping policies exhibit a higher level of robustness against noise in the preceding pose estimation system and object uncertainties.
MoreTranslated text
Key words
machine learning,reinforcement learning,simulation,gripping,robot
AI Read Science
Must-Reading Tree
Example
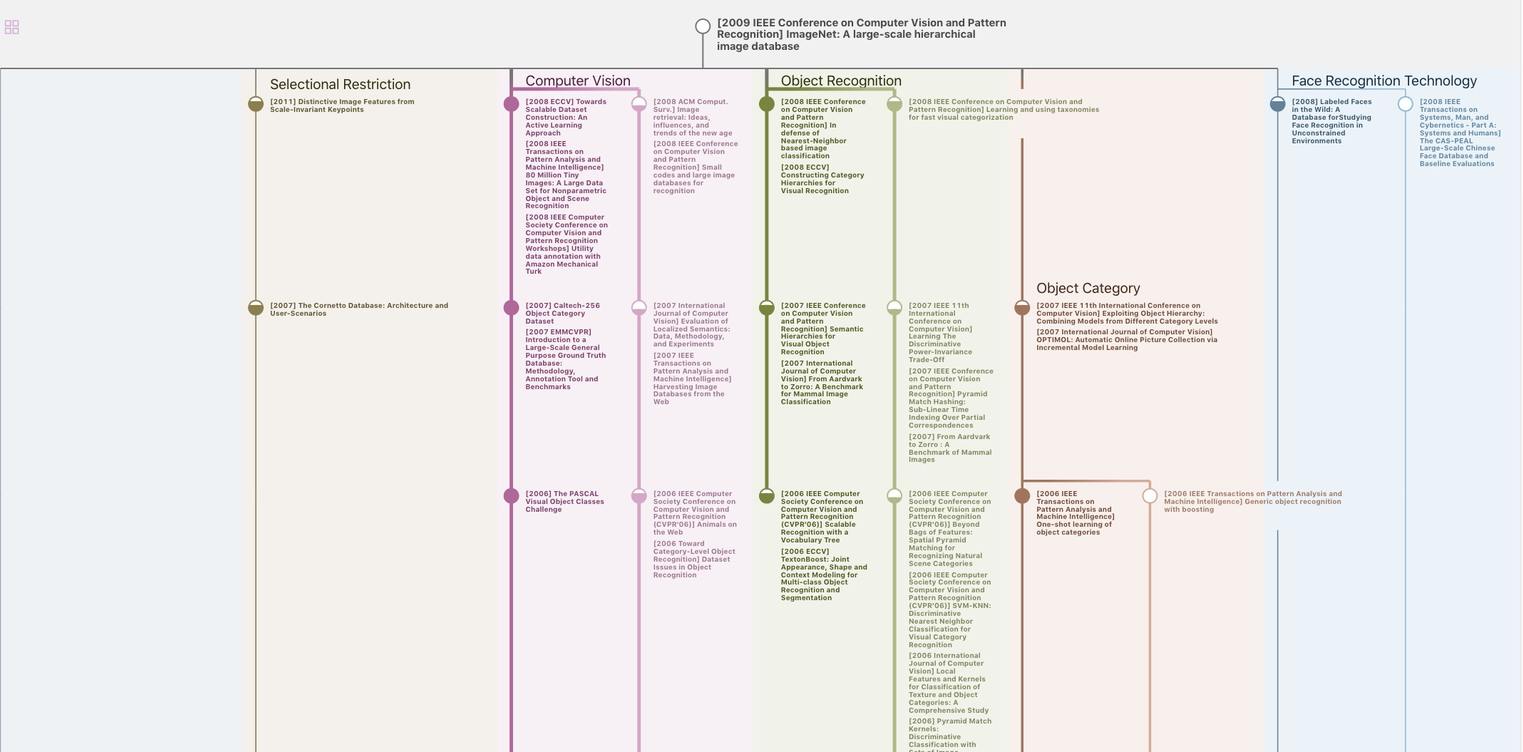
Generate MRT to find the research sequence of this paper
Chat Paper
Summary is being generated by the instructions you defined