Universal scaling between wave speed and size enables nanoscale high-performance reservoir computing based on propagating spin-waves
npj Spintronics(2024)
Abstract
Physical implementation of neuromorphic computing using spintronics technology has attracted recent attention for the future energy-efficient AI at nanoscales. Reservoir computing (RC) is promising for realizing the neuromorphic computing device. By memorizing past input information and its nonlinear transformation, RC can handle sequential data and perform time-series forecasting and speech recognition. However, the current performance of spintronics RC is poor due to the lack of understanding of its mechanism. Here we demonstrate that nanoscale physical RC using propagating spin waves can achieve high computational power comparable with other state-of-art systems. We develop the theory with response functions to understand the mechanism of high performance. The theory clarifies that wave-based RC generates Volterra series of the input through delayed and nonlinear responses. The delay originates from wave propagation. We find that the scaling of system sizes with the propagation speed of spin waves plays a crucial role in achieving high performance.
MoreTranslated text
AI Read Science
Must-Reading Tree
Example
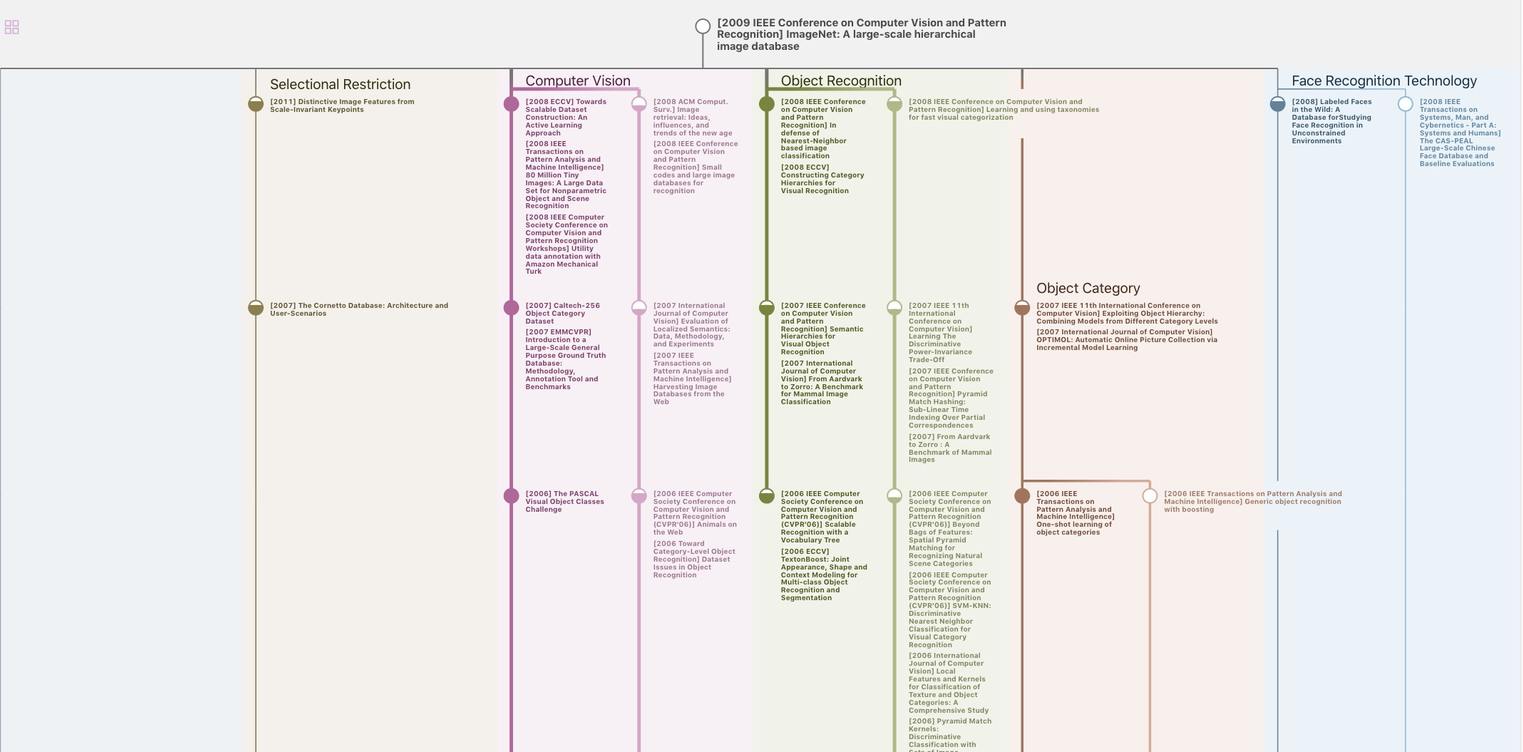
Generate MRT to find the research sequence of this paper
Chat Paper
Summary is being generated by the instructions you defined