A trained Mask R-CNN model over PlanetScope imagery for very-high resolution surface water mapping in boreal forest-tundra
REMOTE SENSING OF ENVIRONMENT(2024)
Abstract
Small water bodies (< 0.01 km(2)) showing diverse limnological properties occur in great abundance across the boreal forest and tundra landscapes of the Arctic and Subarctic. However, their classification, geographical distribution and collective importance for water, heat, nutrient, contaminant and carbon cycles are still poorly constrained. One important step for better understanding the role and evolution of small water bodies in the fastchanging northern landscapes is to develop image analysis protocols that allow their automatic remote sensing detection, delineation and inventory. In this study, we set an image analysis protocol (High Latitude Water - HLWATER V1.0) based on a trained supervised Mask R-CNN deep learning model over PlanetScope imagery for the automatic detection and delineation of small lakes and ponds that were absent in existing datasets. Most of our training dataset comprised water bodies smaller than 0.01 km(2) (97%) and spanned a wide range of environmental and hydrological settings, from the sporadic to the continuous permafrost zones of Canada. The model was tested as a fully autonomous approach for eastern Hudson Bay, Nunavik (Subarctic Canada), a region that poses challenges for water remote sensing given the abundance and variety of small water bodies. These are mainly permafrost thaw and glacial basin ponds in the boreal forest-tundra in challenging optical settings influenced by vegetation or topography shadowing, or revealing peat water logging, fen and bog pond conditions. A multi-scale validation approach was developed using water body delineations from PlanetScope imagery and ultra-high resolution orthomosaics from Unoccupied Aerial Systems. This procedure allowed a sub-pixel assessment and identified the limitations and strengths of the trained model for detecting small and large water bodies. The results varied according to different landscape units, with mean Intersection over Union (IoU) 0.5 F1 Scores of 0.53 to 0.71 and mean F1 Scores of 0.62 to 0.95. Considering 166 m(2) as the minimum pond size detection threshold, the IoU 0.5 F1 Scores were 0.7 to 0.91 and F1 Scores were 0.76 to 0.83, evaluated by comparing the model results with ultra-high resolution manual delineations. The image analysis protocol and trained model show high potential for extension to other boreal forest-tundra regions of the Arctic and Subarctic, allowing for detailed inventories of optically and morphologically diverse small water bodies over large areas of the circumpolar North.
MoreTranslated text
Key words
Mask R-CNN,Deep learning,PlanetScope,Arctic and subarctic,Water mapping,Small water bodies
AI Read Science
Must-Reading Tree
Example
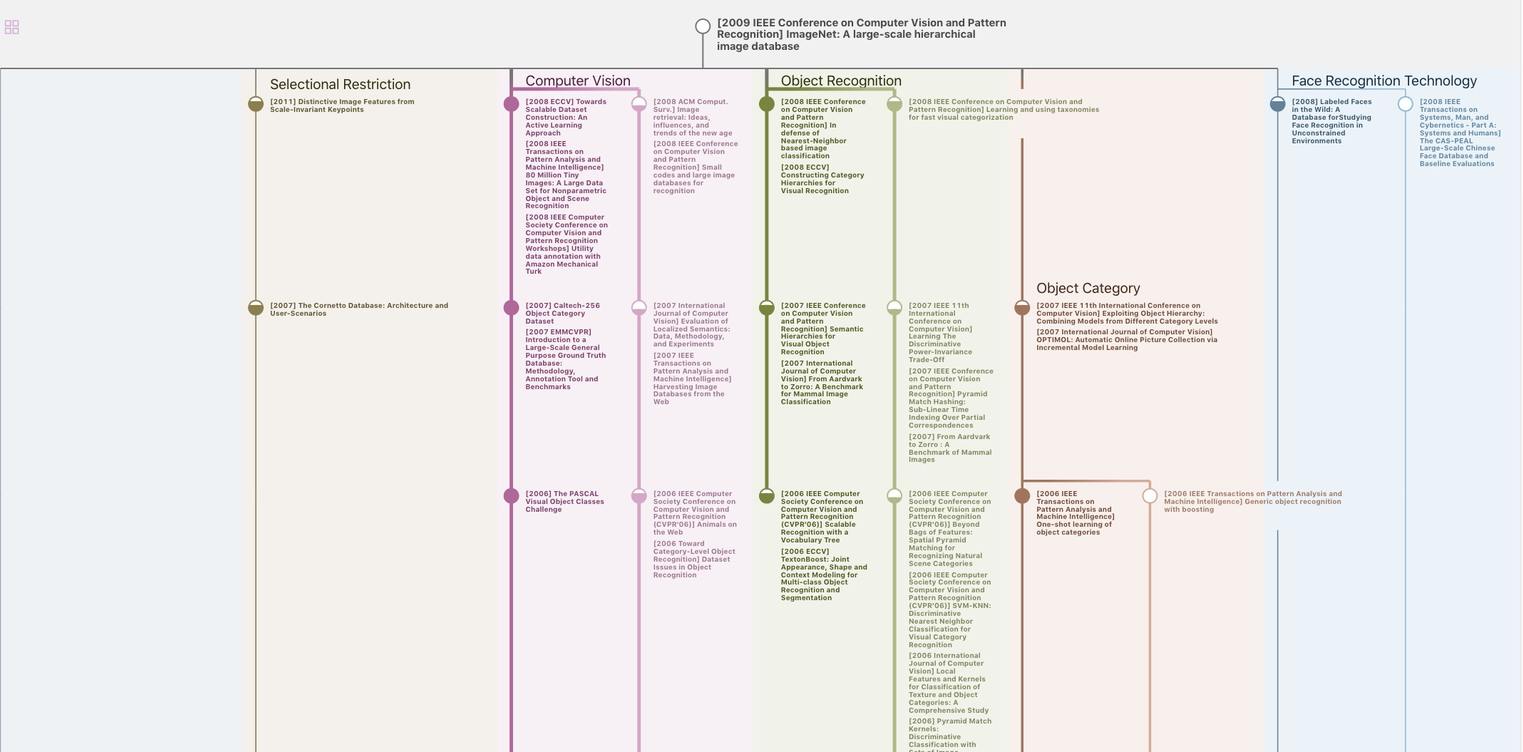
Generate MRT to find the research sequence of this paper
Chat Paper
Summary is being generated by the instructions you defined