Exploring Genomic Large Language Models: Bridging the Gap between Natural Language and Gene Sequences
biorxiv(2024)
摘要
Motivation: With the rapid development of genomic sequencing technologies and accumulation of sequencing data, there is an increasing demand for analysis tools that are more user-friendly for non-programmer users. In support of this initiative, we developed an all-in-one tool called GenomicLLM that can understand simple grammar in the question input and perform different types of analyses and tasks accordingly. Reaults: We trained the GenomicLLM model using three large open-access datasets, namely GenomicLLM_GRCh38, Genome Understanding Evaluation and GenomicBenchmarks, and developed a hybrid tokenization approach to allow better comprehension from mixed corpora that include sequence and non-sequence inputs. GenomicLLM can carry out a wider range of tasks. In the classification tasks that are also available in the state-of-the-art DNABERT-2 and HyenaDNA, GenomicLLM has comparable performance. Moreover, GenomicLLM can also carry out other regression and generation tasks that are not accomplishable by these tools. In summary, we demonstrated here a successful large language model with a mixture of gene sequences and natural language corpus that enables a wider range of applications. Availability and implementation: Codes and data can be accessed at https://github.com/Huatsing-Lau/GenomicLLM and https://zenodo.org/records/10695802
### Competing Interest Statement
The authors have declared no competing interest.
更多查看译文
AI 理解论文
溯源树
样例
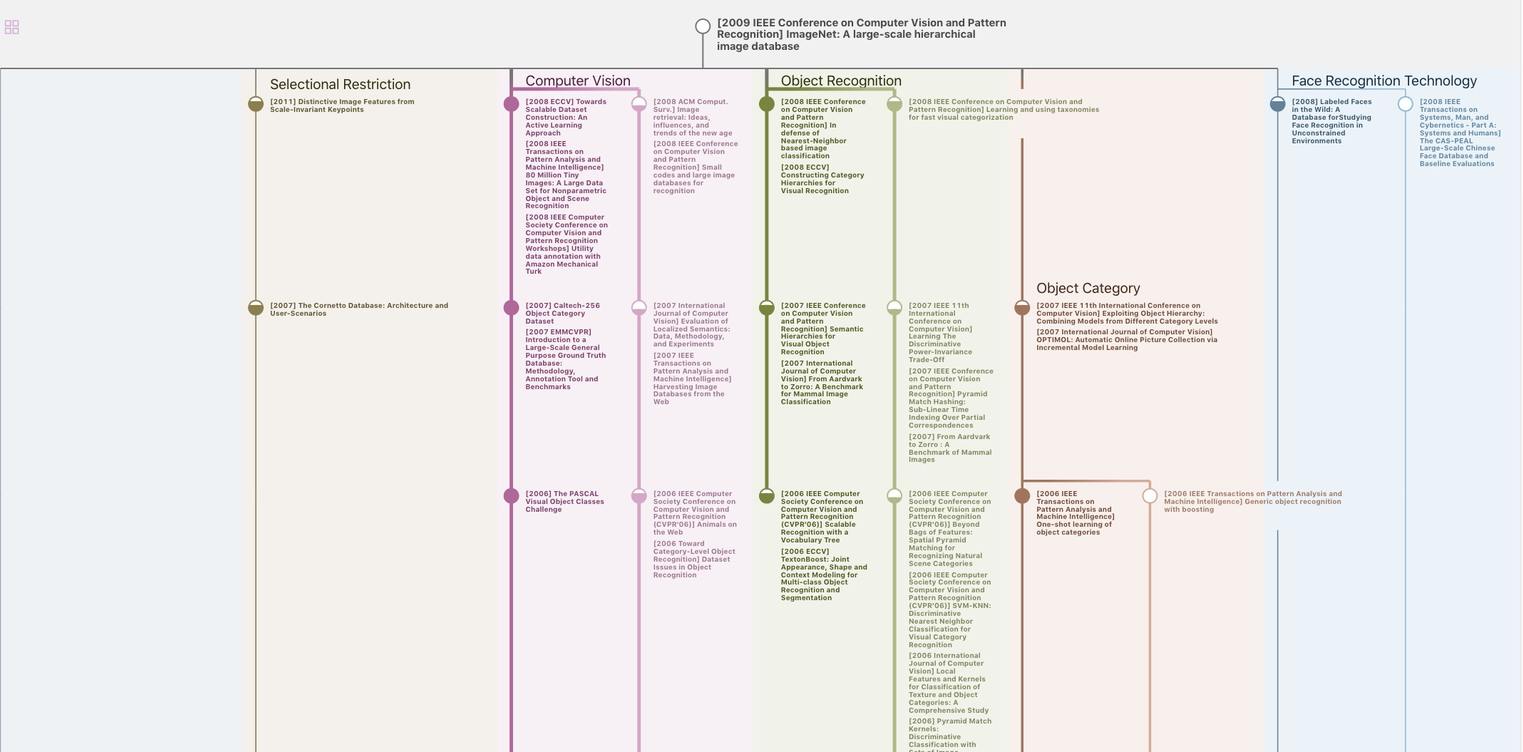
生成溯源树,研究论文发展脉络
Chat Paper
正在生成论文摘要