Negative Sampling in Knowledge Graph Representation Learning: A Review
CoRR(2024)
摘要
Knowledge graph representation learning (KGRL) or knowledge graph embedding
(KGE) plays a crucial role in AI applications for knowledge construction and
information exploration. These models aim to encode entities and relations
present in a knowledge graph into a lower-dimensional vector space. During the
training process of KGE models, using positive and negative samples becomes
essential for discrimination purposes. However, obtaining negative samples
directly from existing knowledge graphs poses a challenge, emphasizing the need
for effective generation techniques. The quality of these negative samples
greatly impacts the accuracy of the learned embeddings, making their generation
a critical aspect of KGRL. This comprehensive survey paper systematically
reviews various negative sampling (NS) methods and their contributions to the
success of KGRL. Their respective advantages and disadvantages are outlined by
categorizing existing NS methods into five distinct categories. Moreover, this
survey identifies open research questions that serve as potential directions
for future investigations. By offering a generalization and alignment of
fundamental NS concepts, this survey provides valuable insights for designing
effective NS methods in the context of KGRL and serves as a motivating force
for further advancements in the field.
更多查看译文
AI 理解论文
溯源树
样例
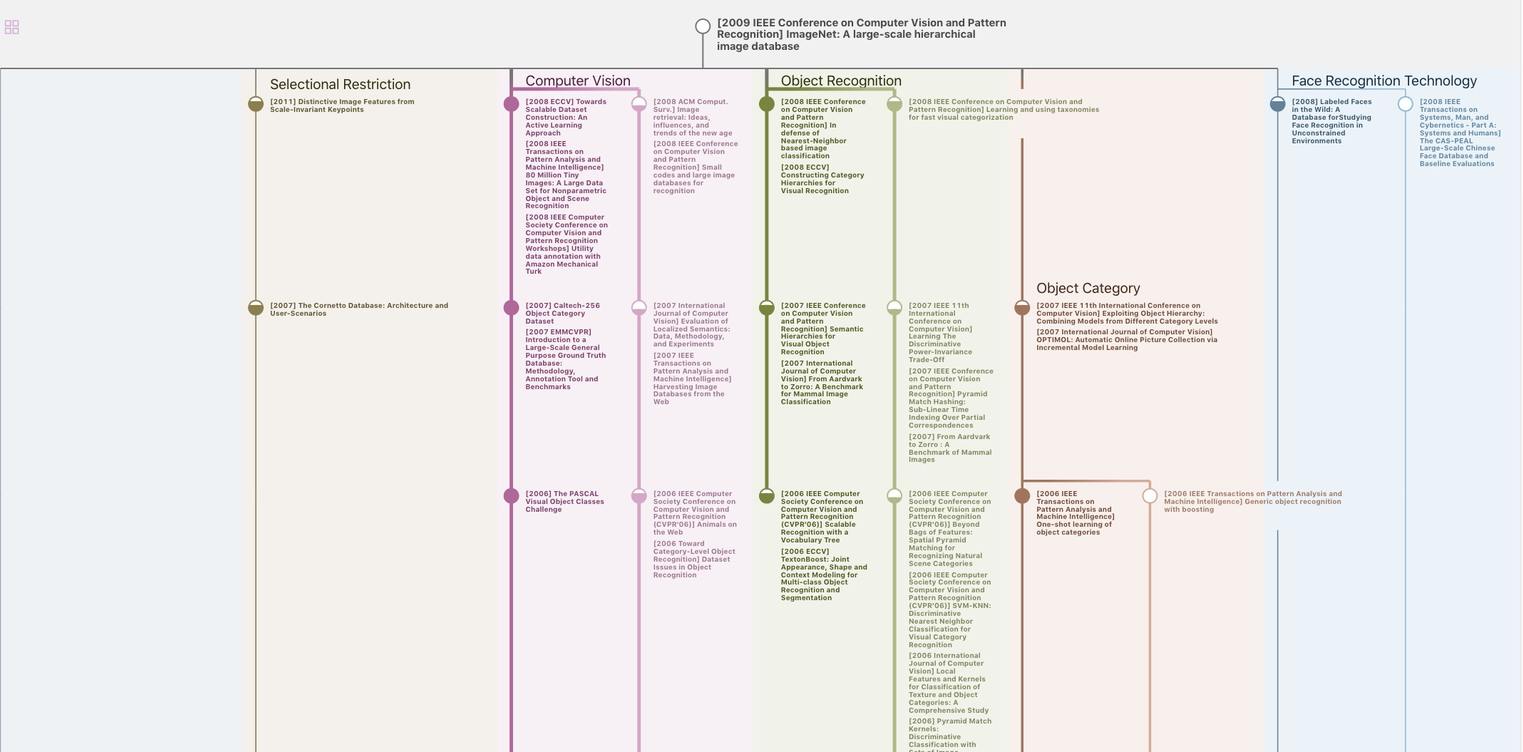
生成溯源树,研究论文发展脉络
Chat Paper
正在生成论文摘要