Unified Generation, Reconstruction, and Representation: Generalized Diffusion with Adaptive Latent Encoding-Decoding
ICML 2024(2024)
摘要
The vast applications of deep generative models are anchored in three core capabilities---generatingnew instances,reconstructinginputs, and learning compactrepresentations---across various data types, such as discrete text/protein sequences and continuous images. Existing model families, like variational autoencoders (VAEs), generative adversarial networks (GANs), autoregressive models, and (latent) diffusion models, generally excel in specific capabilities and data types but fall short in others. We introduceGeneralizedEncoding-DecodingDiffusionProbabilisticModels(EDDPMs) which integrate the core capabilities for broad applicability and enhanced performance. EDDPMs generalize the Gaussian noising-denoising in standard diffusion by introducing parameterized encoding-decoding. Crucially, EDDPMs are compatible with the well-established diffusion model objective and training recipes, allowing effective learning of the encoder-decoder parametersjointlywith diffusion. By choosing appropriate encoder/decoder (e.g., large language models), EDDPMs naturally apply to different data types. Extensive experiments on text, proteins, and images demonstrate the flexibility to handle diverse data and tasks and the strong improvement over various existing models. Code is available at https://github.com/guangyliu/EDDPM .
更多查看译文
AI 理解论文
溯源树
样例
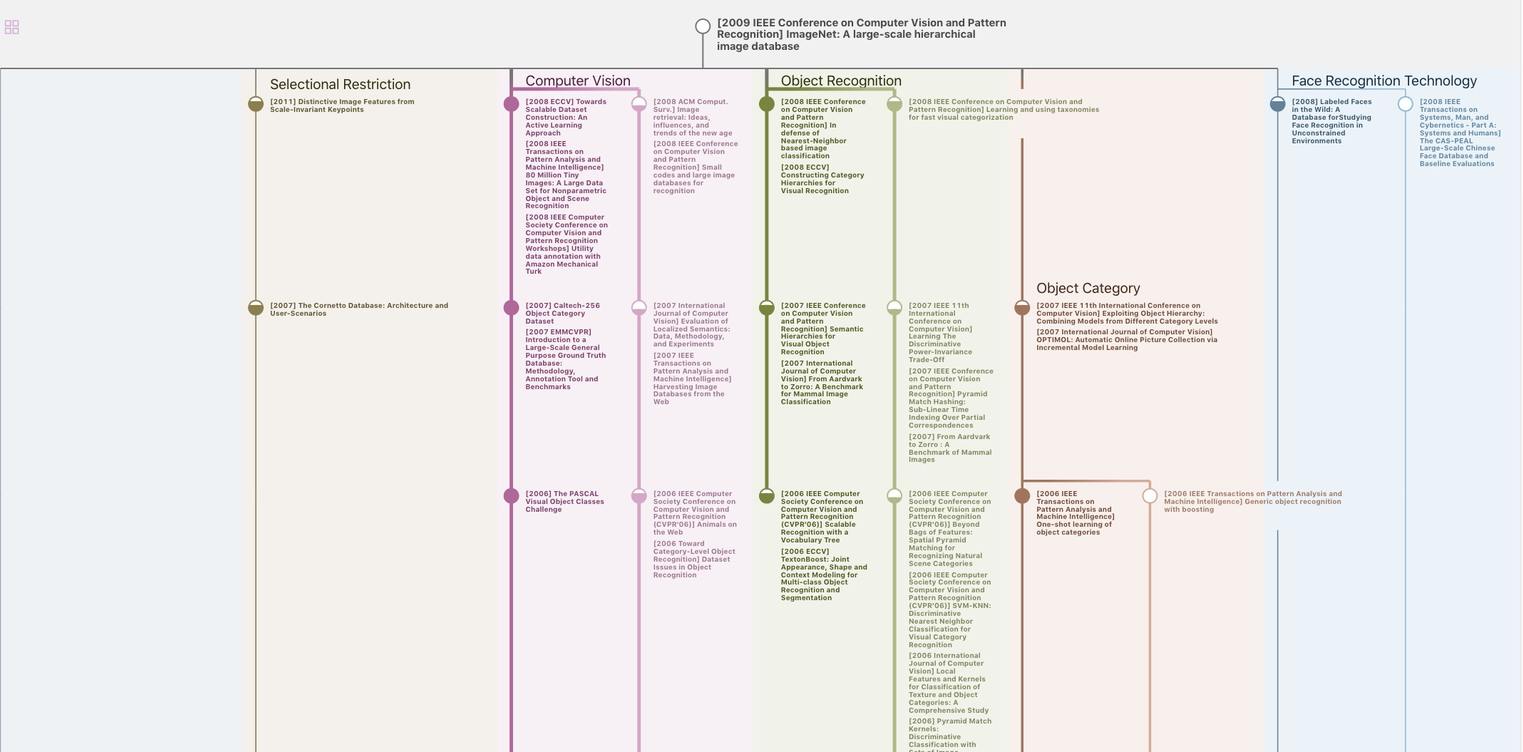
生成溯源树,研究论文发展脉络
Chat Paper
正在生成论文摘要