Seismic Random Noise Suppression Based on Deep Image Prior and Total Variation
IEEE TRANSACTIONS ON GEOSCIENCE AND REMOTE SENSING(2024)
摘要
Deep-learning methods have gained widespread popularity for effectively suppressing random noise in seismic data. The recent progress in techniques based on supervised learning for attenuating seismic random noise underscores their potential, particularly when an abundant set of training examples is accessible. Unfortunately, collecting an adequate amount of representative training samples is not always feasible. Deep image prior (DIP) aims to capture a lot of low-level statistical information by using rich implicit prior knowledge inherent in the structure of the generation network itself. Therefore, it is not essential to provide a training database or uncontaminated data as a truth label, whereas only requires a noisy seismic image. To boost the performance, we add an explicit prior, weighted total variation (WTV), to the standard DIP, which leverages sparsity-promoting priors and restricts the solutions of DIP to satisfy a prior inherent in the seismic data. The proposed method is tested on synthetic seismic data with random noise that follows different distributions and then is applied to field pre- and poststack seismic data. Furthermore, a comparison is drawn between the new method and the traditional DIP-based denoising method in terms of signal-to-noise ratio and local similarity. Our method shows more promising results because prior information from both the structure of the network and the seismic data is considered in the denoising processing.
更多查看译文
关键词
Deep image prior (DIP),seismic data,seismic denoising,total variation (TV)
AI 理解论文
溯源树
样例
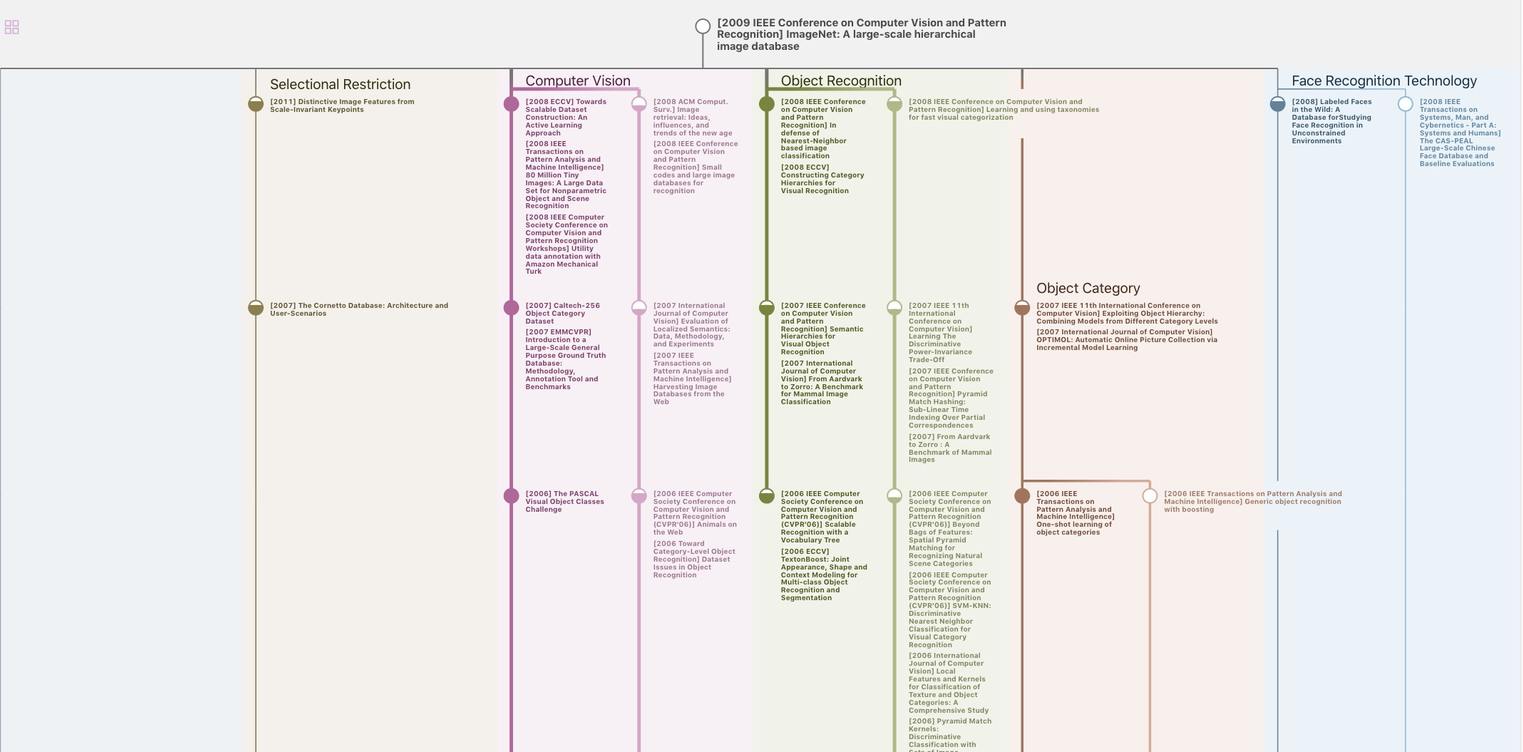
生成溯源树,研究论文发展脉络
Chat Paper
正在生成论文摘要