QFSM: A Novel Quantum Federated Learning Algorithm for Speech Emotion Recognition With Minimal Gated Unit in 5G IoV
IEEE Transactions on Intelligent Vehicles(2024)
摘要
The technology of speech emotion recognition (SER) has been widely applied in the field of human-computer interaction within the Internet of Vehicles (IoV). The incorporation of emerging technologies such as artificial intelligence and big data has accelerated the advancement of SER technology. However, this reveals challenges such as limited computational resources, data processing inefficiency, and security and privacy concerns. In recent years, quantum machine learning has been applied to the field of intelligent transportation, which has demonstrated its various advantages, including high prediction accuracy, robust noise resistance, and strong security. This study first integrates quantum federated learning (QFL) into 5G IoV using a quantum minimal gated unit (QMGU) recurrent neural network for local training. Then, it proposes a novel quantum federated learning algorithm, QFSM, to further enhance computational efficiency and privacy protection. Experimental results demonstrate that compared to existing algorithms using quantum long short-term memory network or quantum gated recurrent unit models, the QFSM algorithm has a higher recognition accuracy and faster training convergence rate. It also performs better in terms of privacy protection and noise robustness, enhancing its applicability and practicality.
更多查看译文
关键词
Quantum federated learning,speech emotion recognition,quantum recurrent neural network,quantum minimal gated unit
AI 理解论文
溯源树
样例
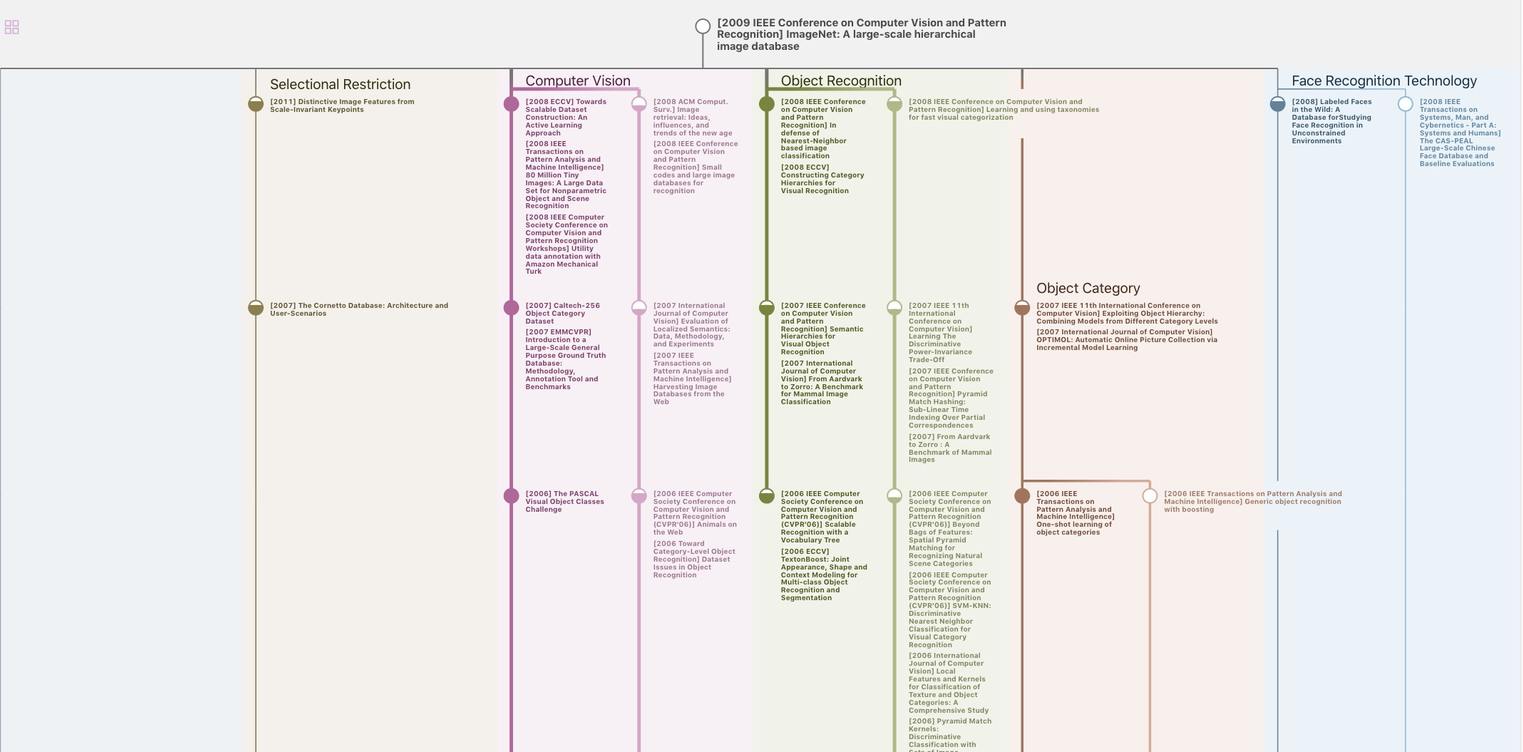
生成溯源树,研究论文发展脉络
Chat Paper
正在生成论文摘要